Nonparametric Empirical Bayes Estimation and Testing for Sparse and Heteroscedastic Signals
arxiv(2021)
摘要
Large-scale modern data often involves estimation and testing for high-dimensional unknown parameters. It is desirable to identify the sparse signals, ``the needles in the haystack'', with accuracy and false discovery control. However, the unprecedented complexity and heterogeneity in modern data structure require new machine learning tools to effectively exploit commonalities and to robustly adjust for both sparsity and heterogeneity. In addition, estimates for high-dimensional parameters often lack uncertainty quantification. In this paper, we propose a novel Spike-and-Nonparametric mixture prior (SNP) -- a spike to promote the sparsity and a nonparametric structure to capture signals. In contrast to the state-of-the-art methods, the proposed methods solve the estimation and testing problem at once with several merits: 1) an accurate sparsity estimation; 2) point estimates with shrinkage/soft-thresholding property; 3) credible intervals for uncertainty quantification; 4) an optimal multiple testing procedure that controls false discovery rate. Our method exhibits promising empirical performance on both simulated data and a gene expression case study.
更多查看译文
关键词
empirical,sparse,signals,estimation
AI 理解论文
溯源树
样例
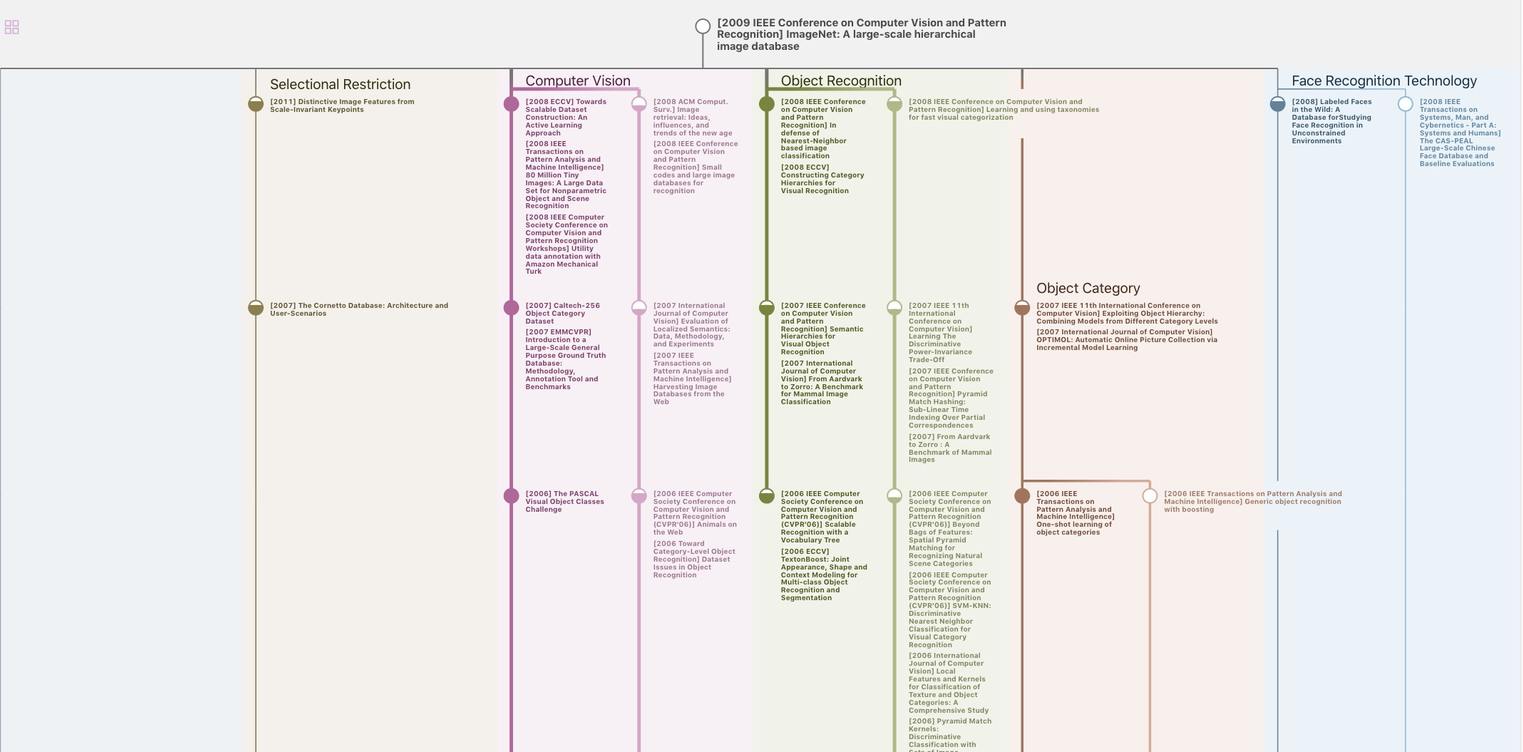
生成溯源树,研究论文发展脉络
Chat Paper
正在生成论文摘要