Planning on a (Risk) Budget: Safe Non-Conservative Planning in Probabilistic Dynamic Environments
2021 IEEE INTERNATIONAL CONFERENCE ON ROBOTICS AND AUTOMATION (ICRA 2021)(2021)
摘要
Planning in environments with other agents whose future actions are uncertain often requires compromise between safety and performance. Here our goal is to design efficient planning algorithms with guaranteed bounds on the probability of safety violation, which nonetheless achieve non-conservative performance. To quantify a system's risk, we define a natural criterion called interval risk bounds (IRBs), which provide a parametric upper bound on the probability of safety violation over a given time interval or task. We present a novel receding horizon algorithm, and prove that it can satisfy a desired IRB. Our algorithm maintains a dynamic risk budget which constrains the allowable risk at each iteration, and guarantees recursive feasibility by requiring a safe set to be reachable by a contingency plan within the budget. We empirically demonstrate that our algorithm is both safer and less conservative than strong baselines in two simulated autonomous driving experiments in scenarios involving collision avoidance with other vehicles, and additionally demonstrate our algorithm running on an autonomous class 8 truck.
更多查看译文
关键词
safe nonconservative planning,probabilistic dynamic environments,agents whose future actions,efficient planning algorithms,guaranteed bounds,safety violation,nonconservative performance,system,natural criterion called interval risk,IRBs,novel receding horizon algorithm,desired IRB,dynamic risk budget,allowable risk,guarantees recursive feasibility,safe set,contingency plan
AI 理解论文
溯源树
样例
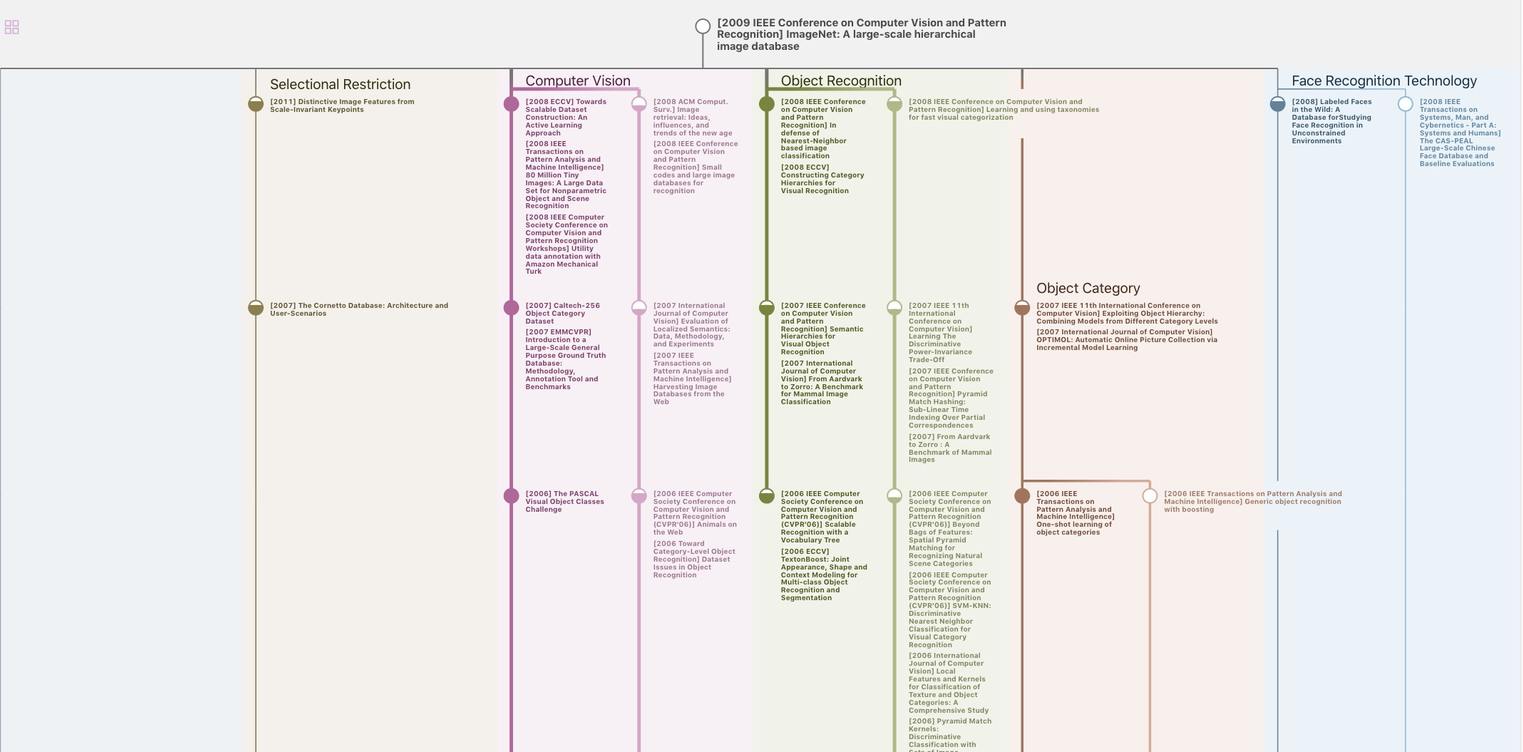
生成溯源树,研究论文发展脉络
Chat Paper
正在生成论文摘要