Localized Uncertainty Attacks
arxiv(2021)
摘要
The susceptibility of deep learning models to adversarial perturbations has stirred renewed attention in adversarial examples resulting in a number of attacks. However, most of these attacks fail to encompass a large spectrum of adversarial perturbations that are imperceptible to humans. In this paper, we present localized uncertainty attacks, a novel class of threat models against deterministic and stochastic classifiers. Under this threat model, we create adversarial examples by perturbing only regions in the inputs where a classifier is uncertain. To find such regions, we utilize the predictive uncertainty of the classifier when the classifier is stochastic or, we learn a surrogate model to amortize the uncertainty when it is deterministic. Unlike $\ell_p$ ball or functional attacks which perturb inputs indiscriminately, our targeted changes can be less perceptible. When considered under our threat model, these attacks still produce strong adversarial examples; with the examples retaining a greater degree of similarity with the inputs.
更多查看译文
关键词
uncertainty attacks
AI 理解论文
溯源树
样例
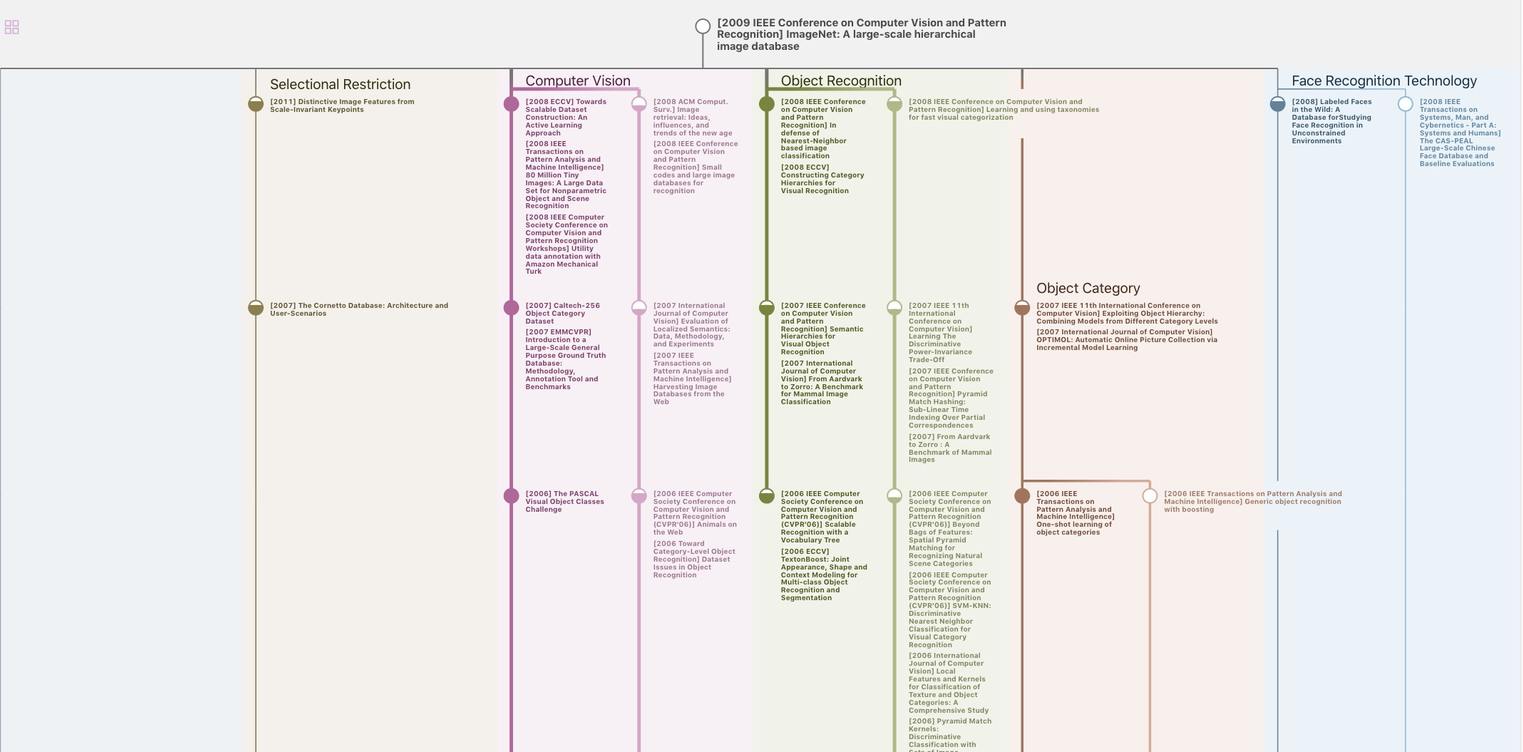
生成溯源树,研究论文发展脉络
Chat Paper
正在生成论文摘要