MHNF: Multi-Hop Heterogeneous Neighborhood Information Fusion Graph Representation Learning
arxiv(2023)
摘要
The attention mechanism enables graph neural networks (GNNs) to learn the attention weights between the target node and its one-hop neighbors, thereby improving the performance further. However, most existing GNNs are oriented toward homogeneous graphs, and in which each layer can only aggregate the information of one-hop neighbors. Stacking multilayer networks introduces considerable noise and easily leads to over smoothing. We propose here a multihop heterogeneous neighborhood information fusion graph representation learning method (MHNF). Specifically, we propose a hybrid metapath autonomous extraction model to efficiently extract multihop hybrid neighbors. Then, we formulate a hop-level heterogeneous information aggregation model, which selectively aggregates different-hop neighborhood information within the same hybrid metapath. Finally, a hierarchical semantic attention fusion model (HSAF) is constructed, which can efficiently integrate different-hop and different-path neighborhood information. In this fashion, this paper solves the problem of aggregating multihop neighborhood information and learning hybrid metapaths for target tasks. This mitigates the limitation of manually specifying metapaths. In addition, HSAF can extract the internal node information of the metapaths and better integrate the semantic information present at different levels. Experimental results on real datasets show that MHNF achieves the best or competitive performance against state-of-the-art baselines with only a fraction of 1/10 similar to 1/100 parameters and computational budgets. Our code is publicly available at https://github.com/PHD-lanyu/MHNF.
更多查看译文
关键词
Graph machine learning,heterogeneous graph,graph represent learning,graph neural networks,metapath
AI 理解论文
溯源树
样例
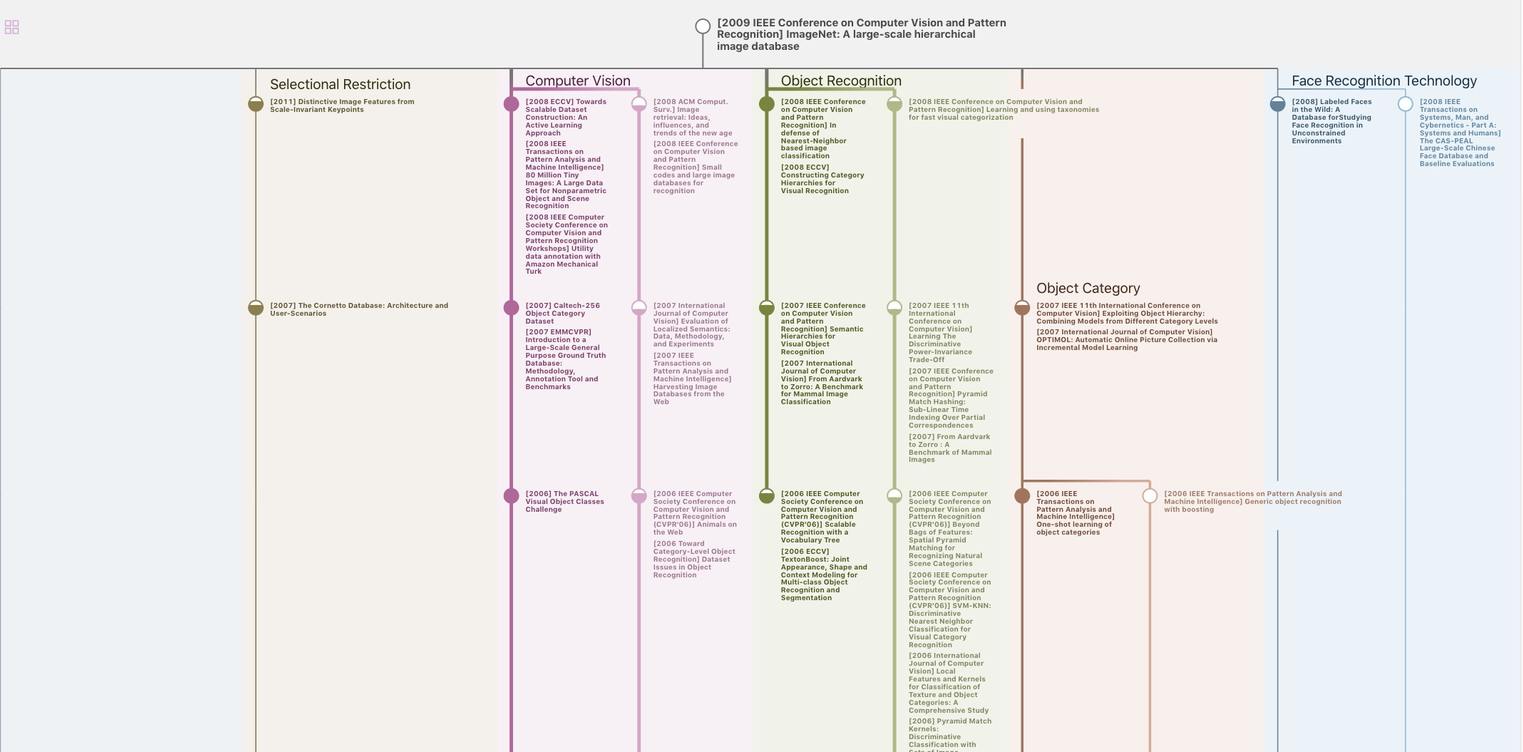
生成溯源树,研究论文发展脉络
Chat Paper
正在生成论文摘要