A deep network construction that adapts to intrinsic dimensionality beyond the domain
Neural Networks(2021)
摘要
We study the approximation of two-layer compositions f(x)=g(ϕ(x)) via deep networks with ReLU activation, where ϕ is a geometrically intuitive, dimensionality reducing feature map. We focus on two intuitive and practically relevant choices for ϕ: the projection onto a low-dimensional embedded submanifold and a distance to a collection of low-dimensional sets. We achieve near optimal approximation rates, which depend only on the complexity of the dimensionality reducing map ϕ rather than the ambient dimension. Since ϕ encapsulates all nonlinear features that are material to the function f, this suggests that deep nets are faithful to an intrinsic dimension governed by f rather than the complexity of the domain of f. In particular, the prevalent assumption of approximating functions on low-dimensional manifolds can be significantly relaxed using functions of type f(x)=g(ϕ(x)) with ϕ representing an orthogonal projection onto the same manifold.
更多查看译文
关键词
Deep neural networks,Approximation theory,Curse of dimensionality,Composite functions,Noisy manifold models
AI 理解论文
溯源树
样例
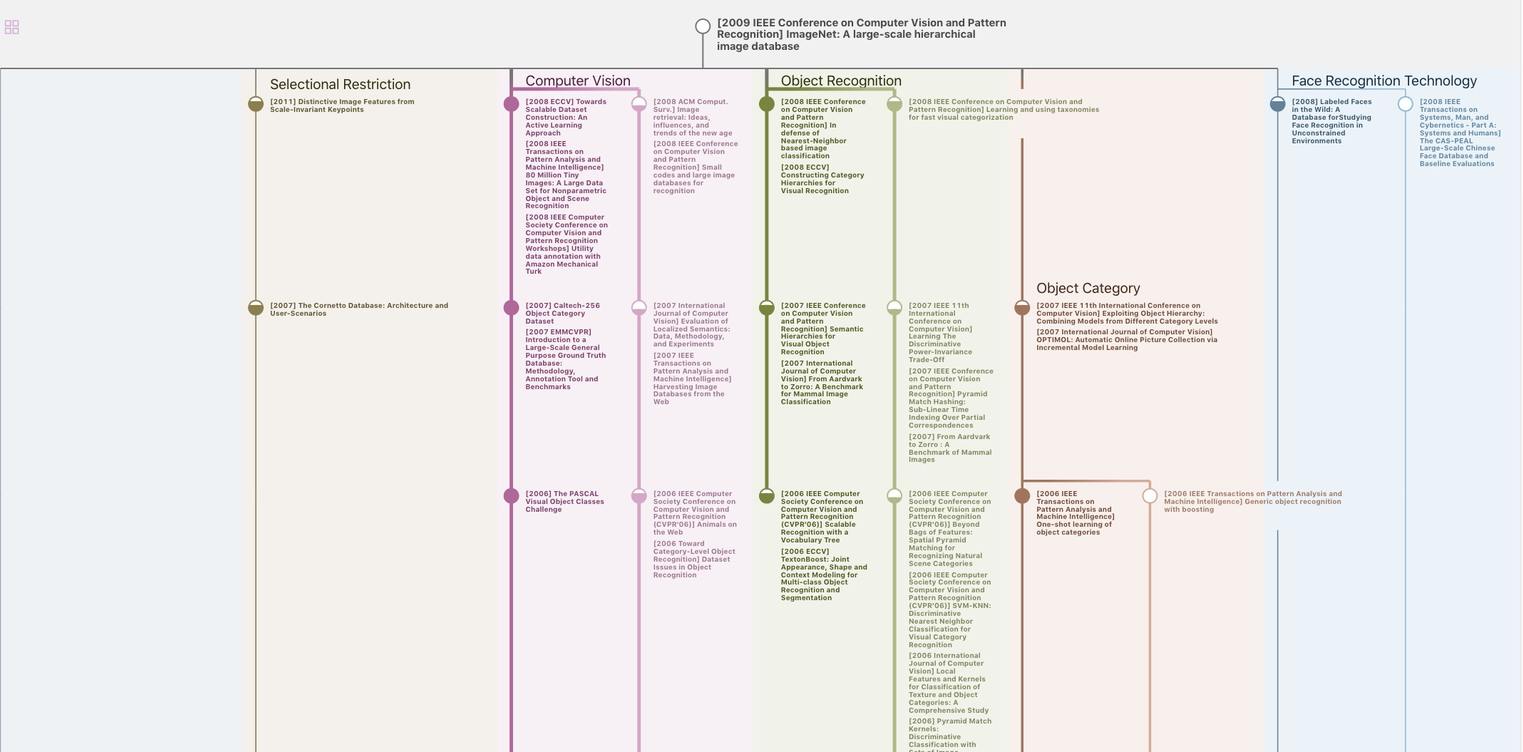
生成溯源树,研究论文发展脉络
Chat Paper
正在生成论文摘要