Evaluating the Robustness of Trigger Set-Based Watermarks Embedded in Deep Neural Networks
arxiv(2023)
摘要
Trigger set-based watermarking schemes have gained emerging attention as they provide a means to prove ownership for deep neural network model owners. In this paper, we argue that state-of-the-art trigger set-based watermarking algorithms do not achieve their designed goal of proving ownership. We posit that this impaired capability stems from two common experimental flaws that the existing research practice has committed when evaluating the robustness of watermarking algorithms: (1) incomplete adversarial evaluation and (2) overlooked adaptive attacks. We conduct a comprehensive adversarial evaluation of 11 representative watermarking schemes against six of the existing attacks and demonstrate that each of these watermarking schemes lacks robustness against at least two non-adaptive attacks. We also propose novel adaptive attacks that harness the adversary's knowledge of the underlying watermarking algorithm of a target model. We demonstrate that the proposed attacks effectively break all of the 11 watermarking schemes, consequently allowing adversaries to obscure the ownership of any watermarked model. We encourage follow-up studies to consider our guidelines when evaluating the robustness of their watermarking schemes via conducting comprehensive adversarial evaluation that includes our adaptive attacks to demonstrate a meaningful upper bound of watermark robustness.
更多查看译文
关键词
watermarks,robustness,neural networks,set-based
AI 理解论文
溯源树
样例
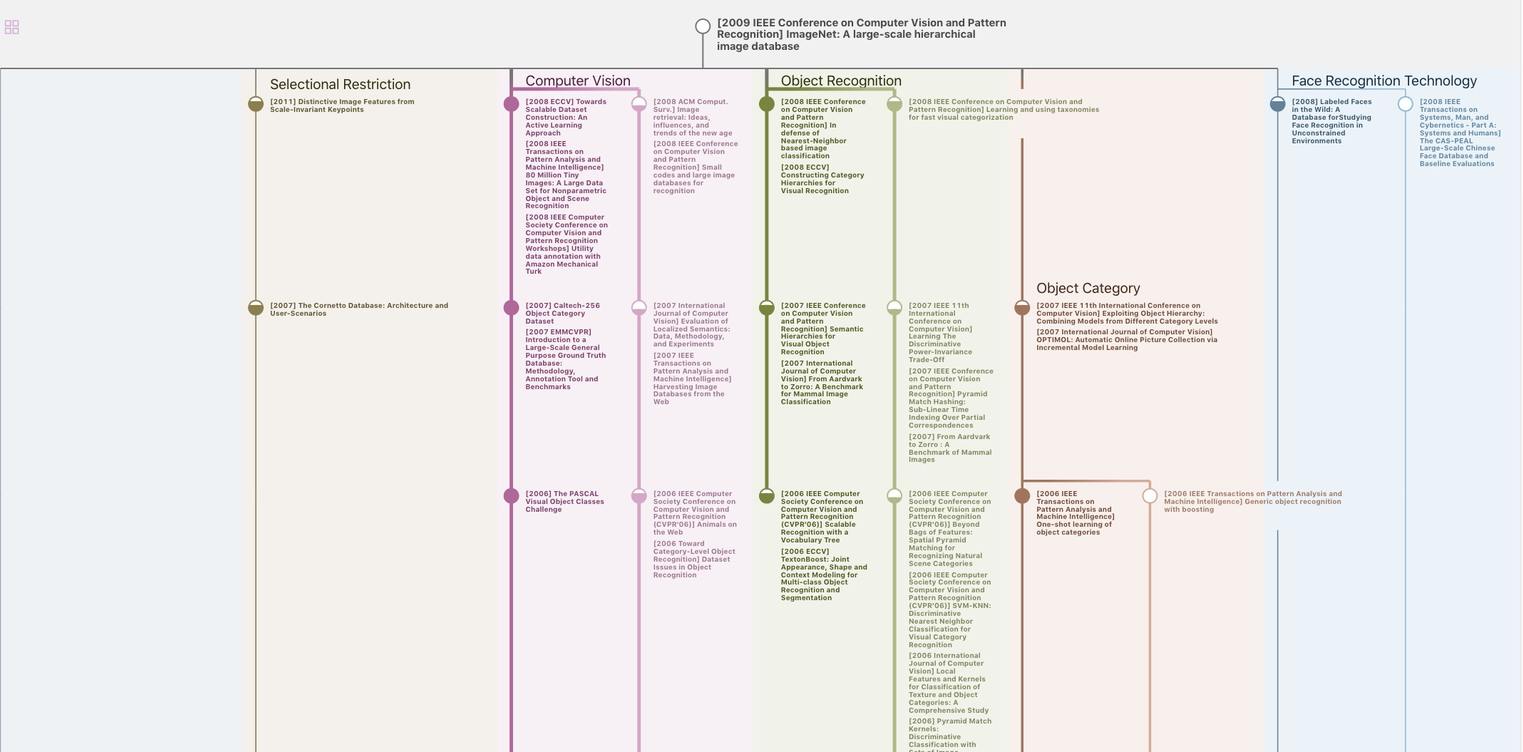
生成溯源树,研究论文发展脉络
Chat Paper
正在生成论文摘要