Rare and different: Anomaly scores from a combination of likelihood and out-of-distribution models to detect new physics at the LHC
SCIPOST PHYSICS(2022)
摘要
We propose a new method to define anomaly scores and apply this to particle physics collider events. Anomalies can be either rare, meaning that these events are a minority in the normal dataset, or different, meaning they have values that are not inside the dataset. We quantify these two properties using an ensemble of One-Class Deep Support Vector Data Description models, which quantifies differentness, and an autoregressive flow model, which quantifies rareness. These two parameters are then combined into a single anomaly score using different combination algorithms. We train the models using a dataset containing only simulated collisions from the Standard Model of particle physics and test it using various hypothetical signals in four different channels and a secret dataset where the signals are unknown to us. The anomaly detection method described here has been evaluated in a summary paper where it performed very well compared to a large number of other methods. The method is simple to implement and is applicable to other datasets in other fields as well.
更多查看译文
AI 理解论文
溯源树
样例
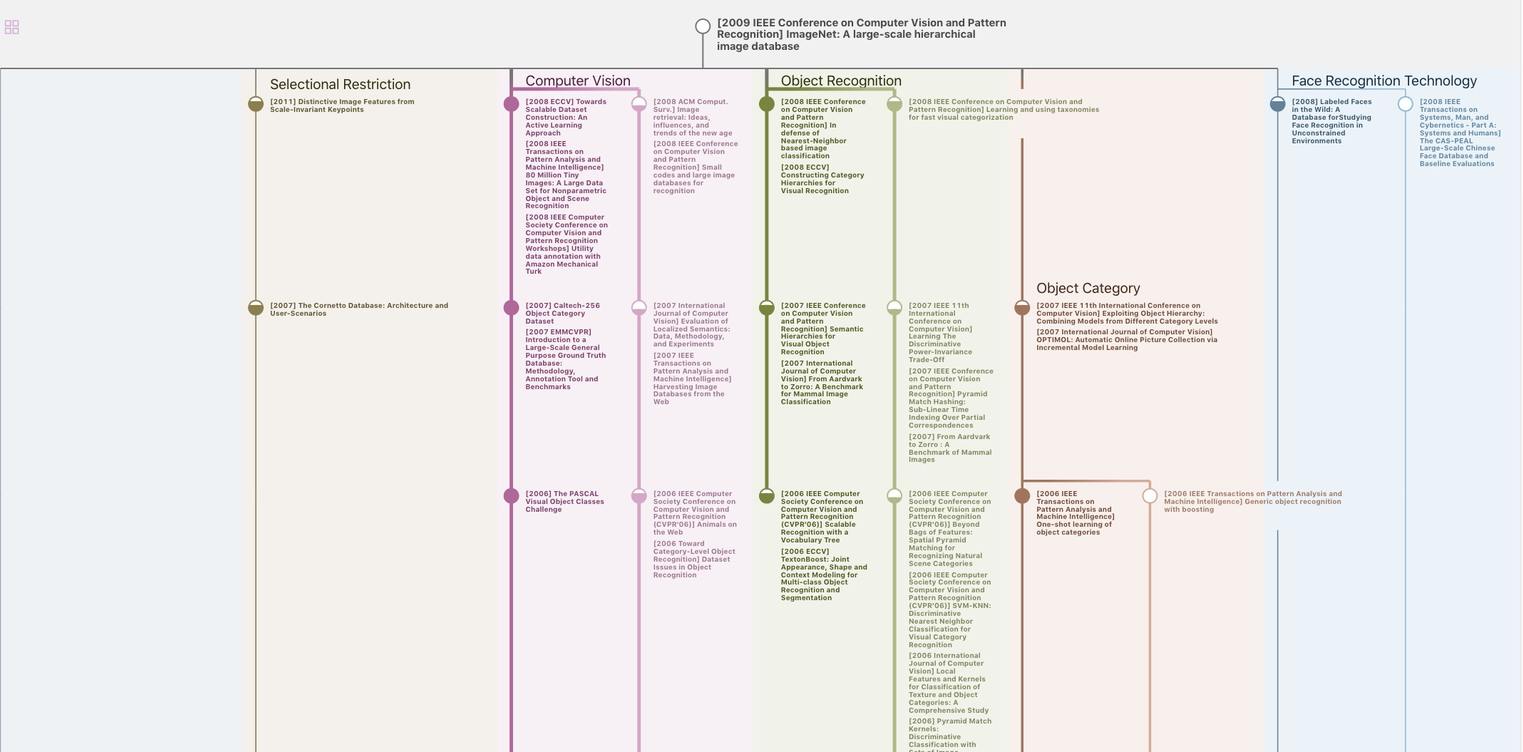
生成溯源树,研究论文发展脉络
Chat Paper
正在生成论文摘要