Terminal-Side Data Rate Prediction For High-Mobility Users
2021 IEEE 93RD VEHICULAR TECHNOLOGY CONFERENCE (VTC2021-SPRING)(2021)
摘要
The possibility of predicting Quality of Service, and particularly data rates, in mobile networks will enable new applications for future automated and connected mobility, such as teleoperated driving. Since network data is difficult to acquire and usually of low granularity, robust prediction approaches are required that need to be trained with data sets and measurements generated by end devices. In this paper, we present uplink and downlink data sets, measured in extensive drive tests, that are made available for the evaluation of machine learning methods. Based on this data, we compare the data rate prediction performance in uplink and downlink for neural network, random forest and gradient boosting approaches. Our results show a significantly higher achievable accuracy in uplink than in downlink, and that, even with reduced feature sets, gradient boosting is particularly suited for the prediction task. Moreover, we investigate the use of quantile estimation methods for predicting bounds on the achievable data rate. We show that conformalized approaches, both based on neural networks and random forests, can predict quantiles with very high accuracy.
更多查看译文
关键词
QoS prediction,data rate prediction,machine learning,drive test data,quantile regression
AI 理解论文
溯源树
样例
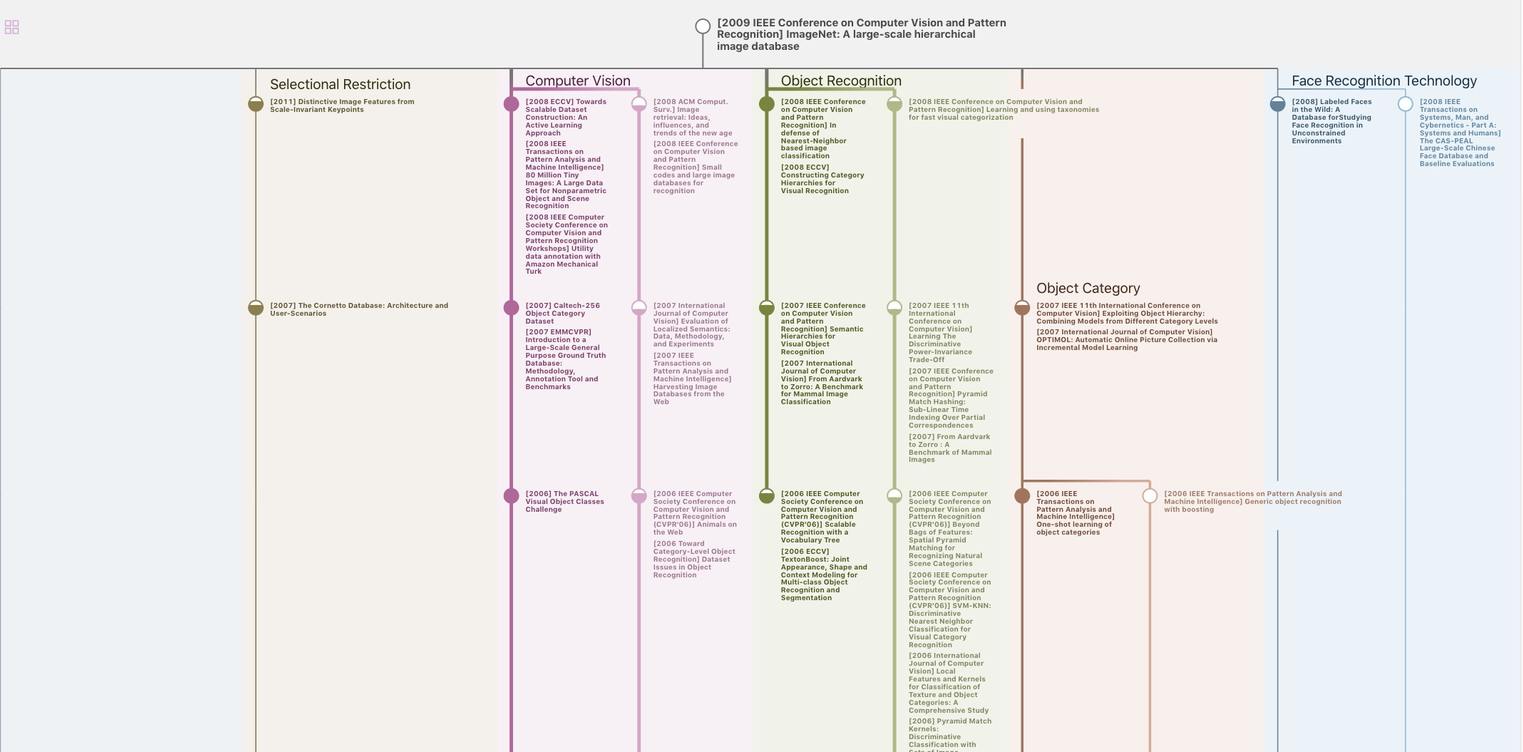
生成溯源树,研究论文发展脉络
Chat Paper
正在生成论文摘要