A Pose Proposal And Refinement Network For Better 6d Object Pose Estimation
2021 IEEE WINTER CONFERENCE ON APPLICATIONS OF COMPUTER VISION WACV 2021(2021)
摘要
In this paper, we present a novel, end-to-end 6D object pose estimation method that operates on RGB inputs. Our approach is composed of 2 main components: the first component classifies the objects in the input image and proposes an initial 6D pose estimate through a multi-task, CNN-based encoder/multi-decoder module. The second component, a refinement module, includes a renderer and a multi-attentional pose refinement network, which iteratively refines the estimated poses by utilizing both appearance features and flow vectors. Our refiner takes advantage of the hybrid representation of the initial pose estimates to predict the relative errors with respect to the target poses. It is further augmented by a spatial multi-attention block that emphasizes objects' discriminative feature parts. Experiments on three benchmarks for 6D pose estimation show that our proposed pipeline outperforms state-of-the-art RGB-based methods with competitive runtime performance.
更多查看译文
关键词
spatial multiattention block,RGB-based methods,pose proposal,6D object pose estimation,refinement module,CNN-based encoder-multidecoder module,multiattentional pose refinement network,appearance features,flow vectors,hybrid representation,object discriminative feature parts
AI 理解论文
溯源树
样例
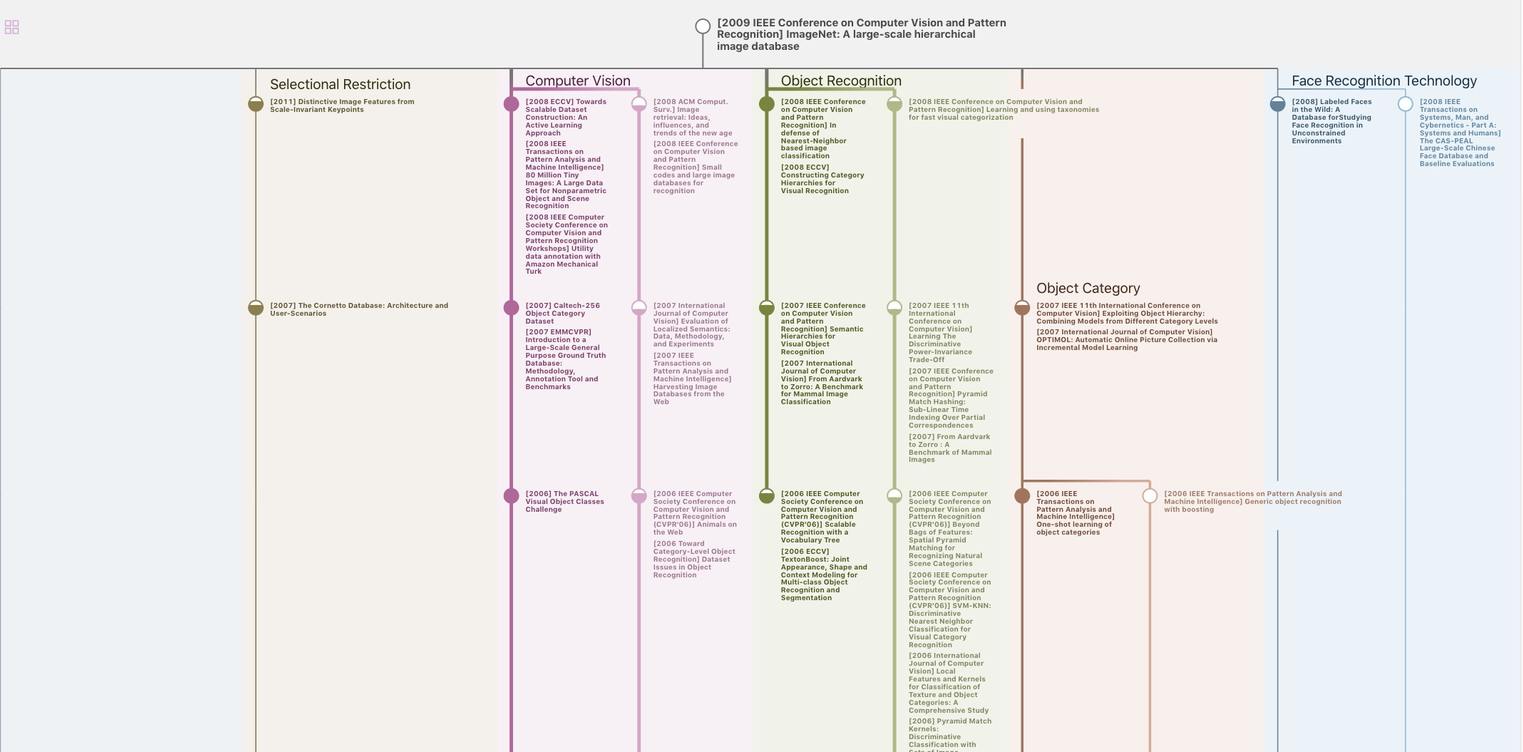
生成溯源树,研究论文发展脉络
Chat Paper
正在生成论文摘要