Vertex-Centric Visual Programming for Graph Neural Networks
International Conference on Management of Data(2021)
摘要
ABSTRACTGraph neural networks (GNNs) have achieved remarkable performance in many graph analytics tasks such as node classification, link prediction and graph clustering. Existing GNN systems (e.g., PyG and DGL) adopt a tensor-centric programming model and train GNNs with manually written operators. Such design results in poor usability due to the large semantic gap between the API and the GNN models, and suffers from inferior efficiency because of high memory consumption and massive data movement. We demonstrateSeastar, a novel GNN training framework that adopts avertex-centric programming paradigm and supportsautomatic kernel generation, to simplify model development and improve training efficiency. We will (i) show how to express GNN models succinctly using a visual "drag-and-drop'' interface or Seastar's vertex-centric python API; (ii) demonstrate the performance advantage of Seastar over existing GNN systems in convergence speed, training throughput and memory consumption; and (iii) illustrate how Seastar's optimizations (e.g., operator fusion and constant folding) improve training efficiency by profiling the run-time performance.
更多查看译文
关键词
Graph Neural Network, Deep Learning System
AI 理解论文
溯源树
样例
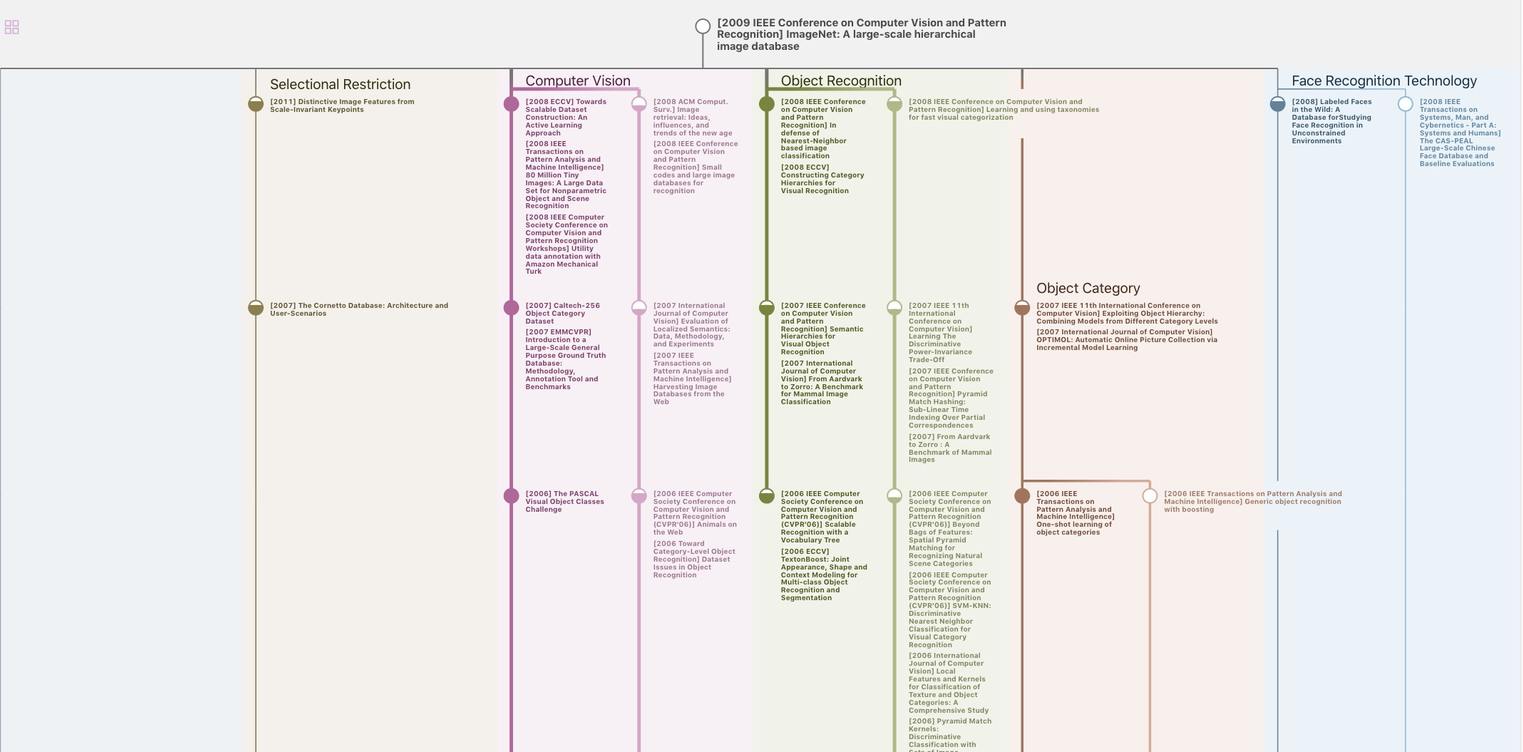
生成溯源树,研究论文发展脉络
Chat Paper
正在生成论文摘要