SPPL: Probabilistic Programming with Fast Exact Symbolic Inference
PLDI(2021)
摘要
We present the Sum-Product Probabilistic Language (SPPL), a new probabilistic programming language that automatically delivers exact solutions to a broad range of probabilistic inference queries. SPPL translates probabilistic programs into sum-product expressions, a new symbolic representation and associated semantic domain that extends standard sum-product networks to support mixed-type distributions, numeric transformations, logical formulas, and pointwise and set-valued constraints. We formalize SPPL via a novel translation strategy from probabilistic programs to sum-product expressions and give sound exact algorithms for conditioning on and computing probabilities of events. SPPL imposes a collection of restrictions on probabilistic programs to ensure they can be translated into sum-product expressions, which allow the system to leverage new techniques for improving the scalability of translation and inference by automatically exploiting probabilistic structure. We implement a prototype of SPPL with a modular architecture and evaluate it on benchmarks the system targets, showing that it obtains up to 3500x speedups over state-of-the-art symbolic systems on tasks such as verifying the fairness of decision tree classifiers, smoothing hidden Markov models, conditioning transformed random variables, and computing rare event probabilities.
更多查看译文
关键词
probabilistic programming,symbolic execution
AI 理解论文
溯源树
样例
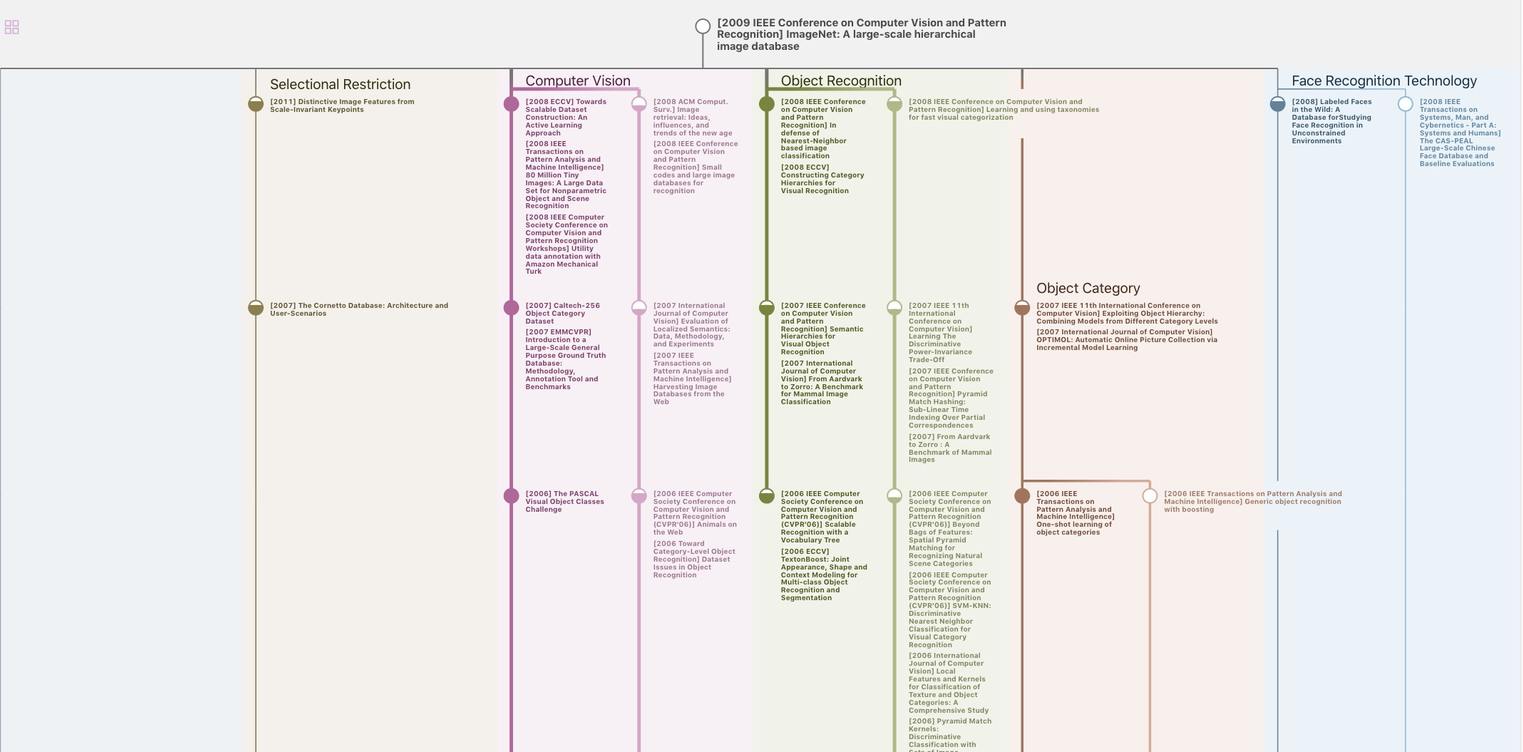
生成溯源树,研究论文发展脉络
Chat Paper
正在生成论文摘要