Scar-Related Ventricular Arrhythmia Prediction from Imaging Using Explainable Deep Learning.
FIMH(2021)
摘要
The aim of this study is to create an automatic framework for sustained ventricular arrhythmia (VA) prediction using cardiac computed tomography (CT) images. We built an image processing pipeline and a deep learning network to explore the relation between post-infarct left ventricular myocardium thickness and previous occurrence of VA. Our pipeline generated a 2D myocardium thickness map (TM) from the 3D imaging input. Our network consisted of a conditional variational autoencoder (CVAE) and a classifier model. The CVAE was used to compress the TM into a low dimensional latent space, then the classifier utilised the latent variables to predict between healthy and VA patient. We studied the network on a large clinical database of 504 healthy and 182 VA patients. Using our method, we achieved a mean classification accuracy of $$75\% \pm 4$$ on the testing dataset, compared to $$71\% \pm 4$$ from the classification using the classical left ventricular ejection fraction (LVEF).
更多查看译文
关键词
explainable deep learning,deep learning,prediction,scar-related
AI 理解论文
溯源树
样例
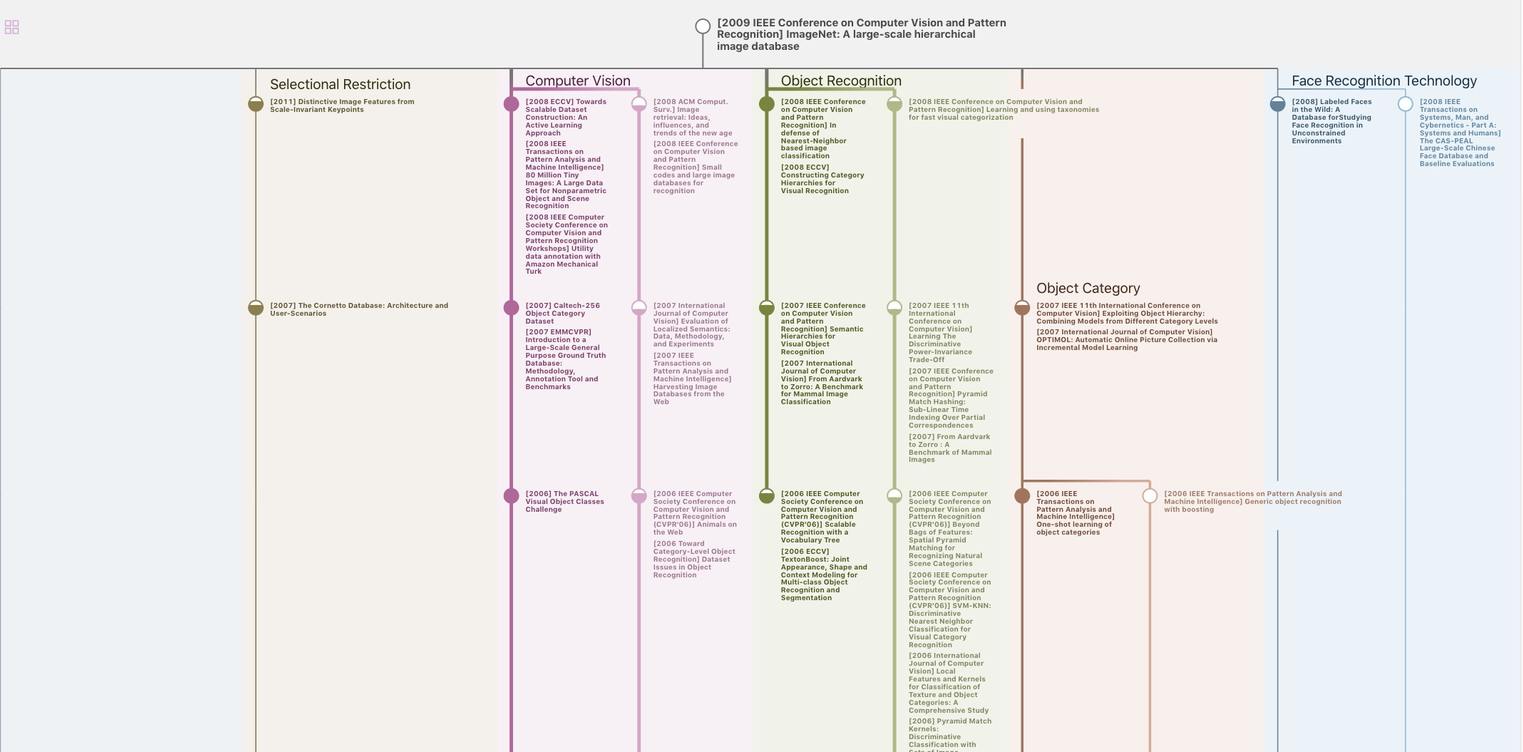
生成溯源树,研究论文发展脉络
Chat Paper
正在生成论文摘要