Image-Derived Geometric Characteristics Predict Abdominal Aortic Aneurysm Growth in a Machine Learning Model.
FIMH(2021)
摘要
Abdominal aortic aneurysm (AAA) growth is correlated with rupture risk, but predicting either AAA growth or rupture remains challenging. Global aneurysm geometric properties have been linked with elevated peak AAA wall stress when using finite element analysis (FEA) and may predict AAA growth. We used a machine learning model to evaluate whether image-derived geometric parameters, calculated both globally and locally over the surface of the aneurysm can predict local AAA wall growth, avoiding material property assumptions used in FEA. Sequential CTAs one year apart were collected from 10 patients with AAAs. The luminal and aortic wall were segmented in patient’s baseline CTA. In order to calculate local geometric properties, each baseline AAA was divided into 64 regions to define regional geometric aneurysm characteristics from vertices in that region, and into 1,500 sub-regions in order to define sub-regional geometric characteristics. The global and local (regional and sub-regional) aortic geometric properties were all derived from the images and determined from the aortic segmentation and surface mesh. Local AAA growth between CTAs was determined at the sub-regional level using deformable image registration and was the outcome variable for the model. Patient demographics, as well as the global and local geometric aneurysm properties were used to predict local AAA growth using an eXtreme gradient boosted regression tree using a performance metric of root-mean-square error (RMSE) with 80/20 training to testing split. Mean relative error in predicting maximum AAA growth was 10.5% in the testing set. The most impactful predictors were AAA volume, regional maximum diameter, regional maximum Gaussian surface curvature, regional median aneurysm thickness, and patient age. Removal of local geometric properties from the model increased RMSE from 0.5 to 1.1 and decreased model performance by likelihood test (P = 0.01). Utilizing both global and local aneurysm geometric characteristics better predicts local aortic wall growth in AAAs, avoiding assumptions required using FEA.
更多查看译文
关键词
abdominal aortic aneurysm,machine learning,model,image-derived
AI 理解论文
溯源树
样例
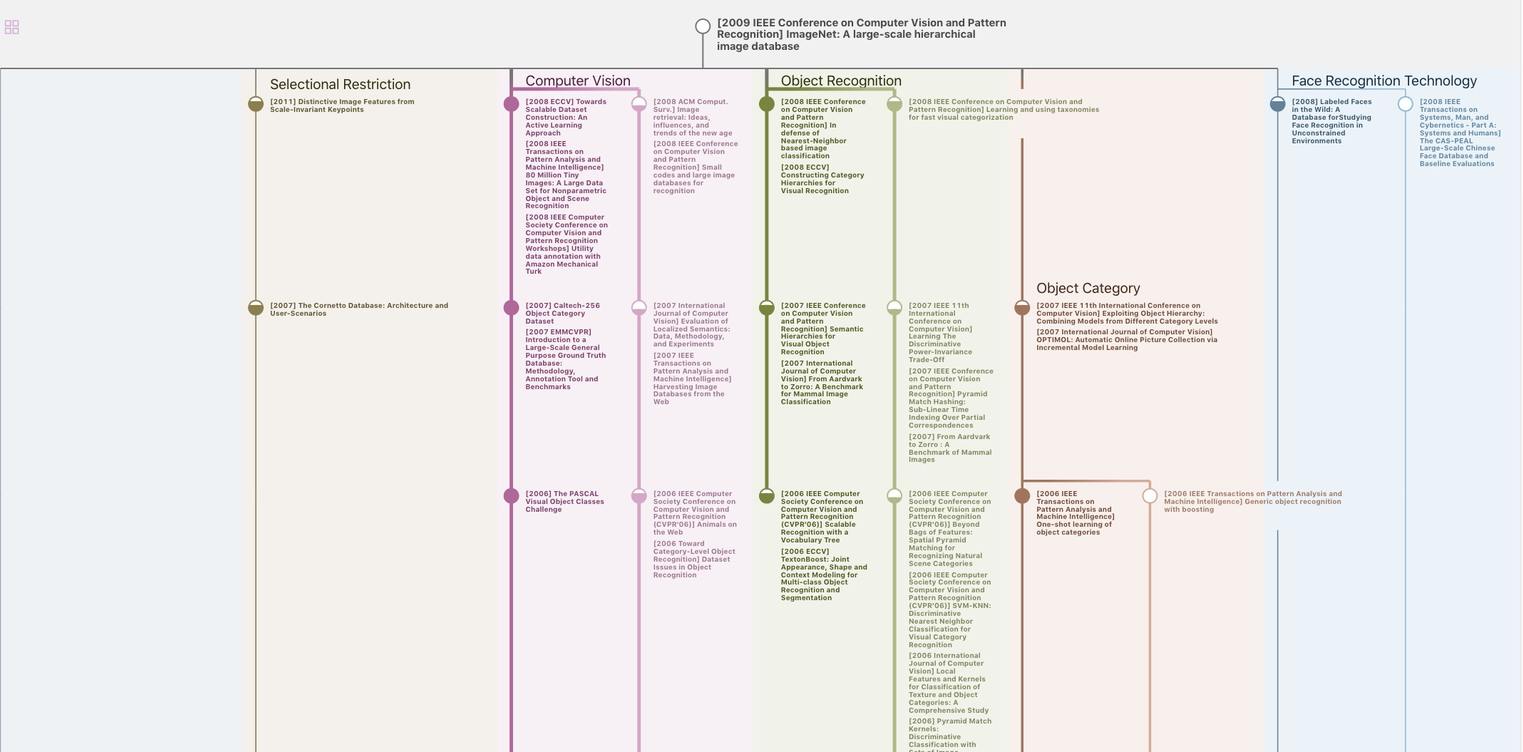
生成溯源树,研究论文发展脉络
Chat Paper
正在生成论文摘要