Boosting Offline Reinforcement Learning with Residual Generative Modeling.
IJCAI(2021)
摘要
Offline reinforcement learning (RL) tries to learn the near-optimal policy with recorded offline experience without online exploration. Current offline RL research includes: 1) generative modeling, i.e., approximating a policy using fixed data; and 2) learning the state-action value function. While most research focuses on the state-action function part through reducing the bootstrapping error in value function approximation induced by the distribution shift of training data, the effects of error propagation in generative modeling have been neglected. In this paper, we analyze the error in generative modeling. We propose AQL (action-conditioned Q-learning), a residual generative model to reduce policy approximation error for offline RL. We show that our method can learn more accurate policy approximations in different benchmark datasets. In addition, we show that the proposed offline RL method can learn more competitive AI agents in complex control tasks under the multiplayer online battle arena (MOBA) game Honor of Kings.
更多查看译文
关键词
residual generative,reinforcement,learning,modeling
AI 理解论文
溯源树
样例
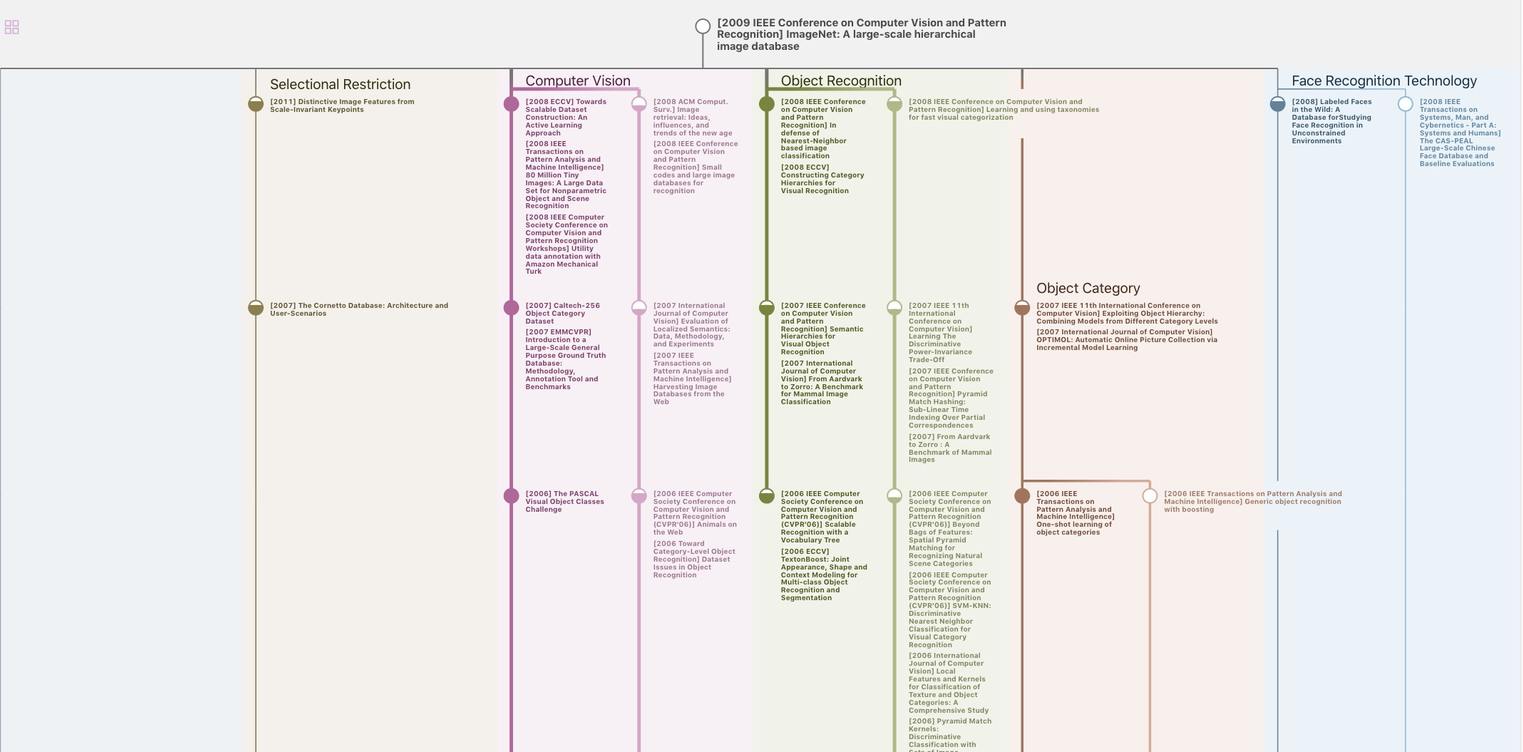
生成溯源树,研究论文发展脉络
Chat Paper
正在生成论文摘要