Encoder-Decoder Based Attractors for End-to-End Neural Diarization
IEEE-ACM TRANSACTIONS ON AUDIO SPEECH AND LANGUAGE PROCESSING(2022)
摘要
This paper investigates an end-to-end neural diarization (EEND) method for an unknown number of speakers. In contrast to the conventional cascaded approach to speaker diarization, EEND methods are better in terms of speaker overlap handling. However, EEND still has a disadvantage in that it cannot deal with a flexible number of speakers. To remedy this problem, we introduce encoder-decoder-based attractor calculation module (EDA) to EEND. Once frame-wise embeddings are obtained, EDA sequentially generates speaker-wise attractors on the basis of a sequence-to-sequence method using an LSTM encoder-decoder. The attractor generation continues until a stopping condition is satisfied; thus, the number of attractors can be flexible. Diarization results are then estimated as dot products of the attractors and embeddings. The embeddings from speaker overlaps result in larger dot product values with multiple attractors; thus, this method can deal with speaker overlaps. Because the maximum number of output speakers is still limited by the training set, we also propose an iterative inference method to remove this restriction. Further, we propose a method that aligns the estimated diarization results with the results of an external speech activity detector, which enables fair comparison against cascaded approaches. Extensive evaluations on simulated and real datasets show that EEND-EDA outperforms the conventional cascaded approach.
更多查看译文
关键词
Training, Transformers, Time-frequency analysis, Voice activity detection, Task analysis, Neural networks, Licenses, Speaker diarization, EEND, EDA
AI 理解论文
溯源树
样例
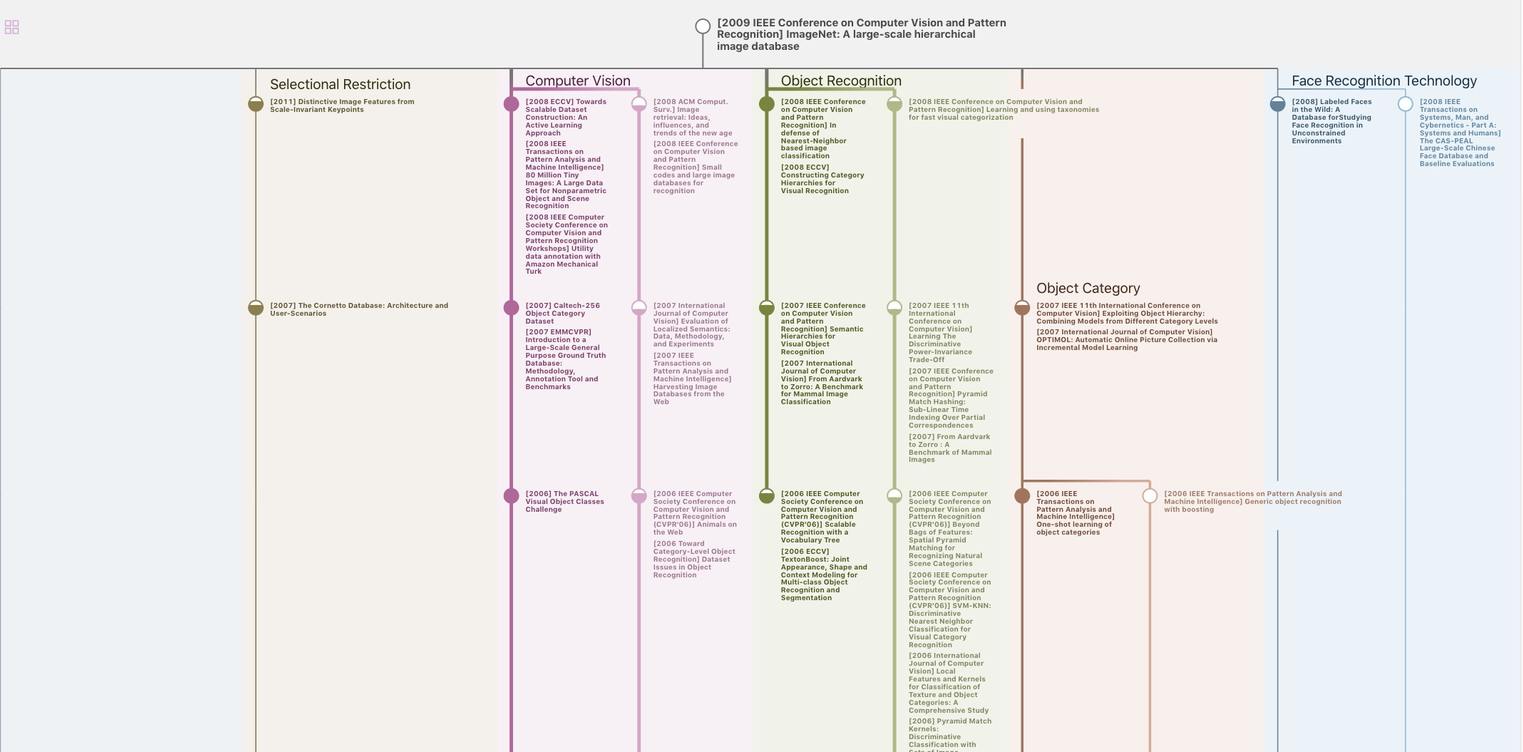
生成溯源树,研究论文发展脉络
Chat Paper
正在生成论文摘要