DiGS : Divergence guided shape implicit neural representation for unoriented point clouds
IEEE Conference on Computer Vision and Pattern Recognition(2022)
摘要
Shape implicit neural representations (INRs) have recently shown to be effective in shape analysis and reconstruction tasks. Existing INRs require point coordinates to learn the implicit level sets of the shape. When a normal vector is available for each point, a higher fidelity representation can be learned, however normal vectors are often not provided as raw data. Furthermore, the method's initialization has been shown to play a crucial role for surface reconstruction. In this paper, we propose a divergence guided shape representation learning approach that does not require normal vectors as input. We show that incorporating a soft constraint on the divergence of the distance function favours smooth solutions that reliably orients gradients to match the unknown normal at each point, in some cases even better than approaches that use ground truth normal vectors directly. Additionally, we introduce a novel geometric initialization method for sinusoidal INRs that further improves convergence to the desired solution. We evaluate the effectiveness of our approach on the task of surface reconstruction and shape space learning and show SOTA performance compared to other unoriented methods. Code and model parameters available at our project page https://chumbyte.github.io/DiGS-Site/
更多查看译文
关键词
Representation learning, Vision + graphics
AI 理解论文
溯源树
样例
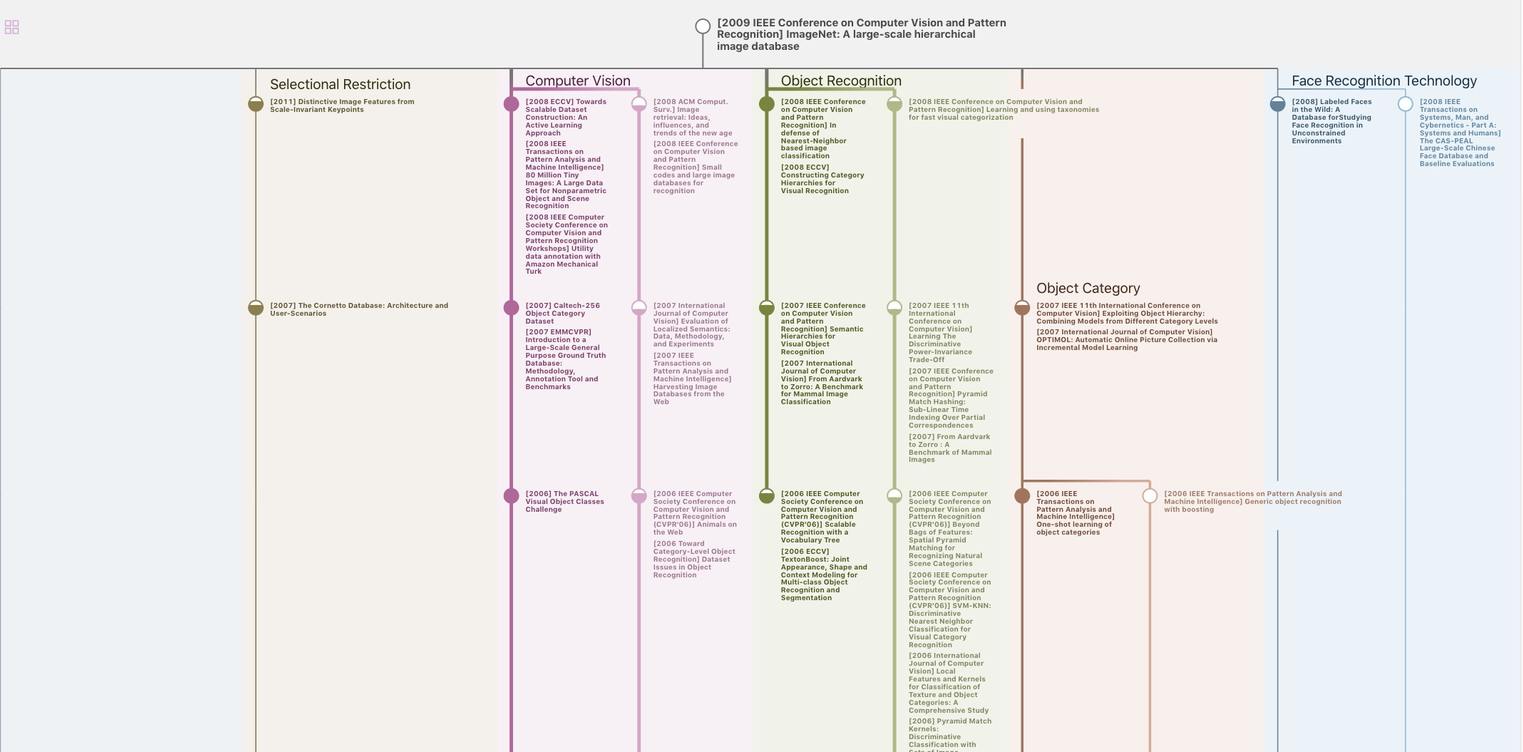
生成溯源树,研究论文发展脉络
Chat Paper
正在生成论文摘要