Matrix encoding networks for neural combinatorial optimization.
Annual Conference on Neural Information Processing Systems(2021)
摘要
Machine Learning (ML) can help solve combinatorial optimization (CO) problems better. A popular approach is to use a neural net to compute on the parameters of a given CO problem and extract useful information that guides the search for good solutions. Many CO problems of practical importance can be specified in a matrix form of parameters quantifying the relationship between two groups of items. There is currently no neural net model, however, that takes in such matrix-style relationship data as an input. Consequently, these types of CO problems have been out of reach for ML engineers. In this paper, we introduce Matrix Encoding Network (MatNet) and show how conveniently it takes in and processes parameters of such complex CO problems. Using an end-to-end model based on MatNet, we solve asymmetric traveling salesman (ATSP) and flexible flow shop (FFSP) problems as the earliest neural approach. In particular, for a class of FFSP we have tested MatNet on, we demonstrate a far superior empirical performance to any methods (neural or not) known to date.
更多查看译文
关键词
neural combinatorial optimization,networks,matrix
AI 理解论文
溯源树
样例
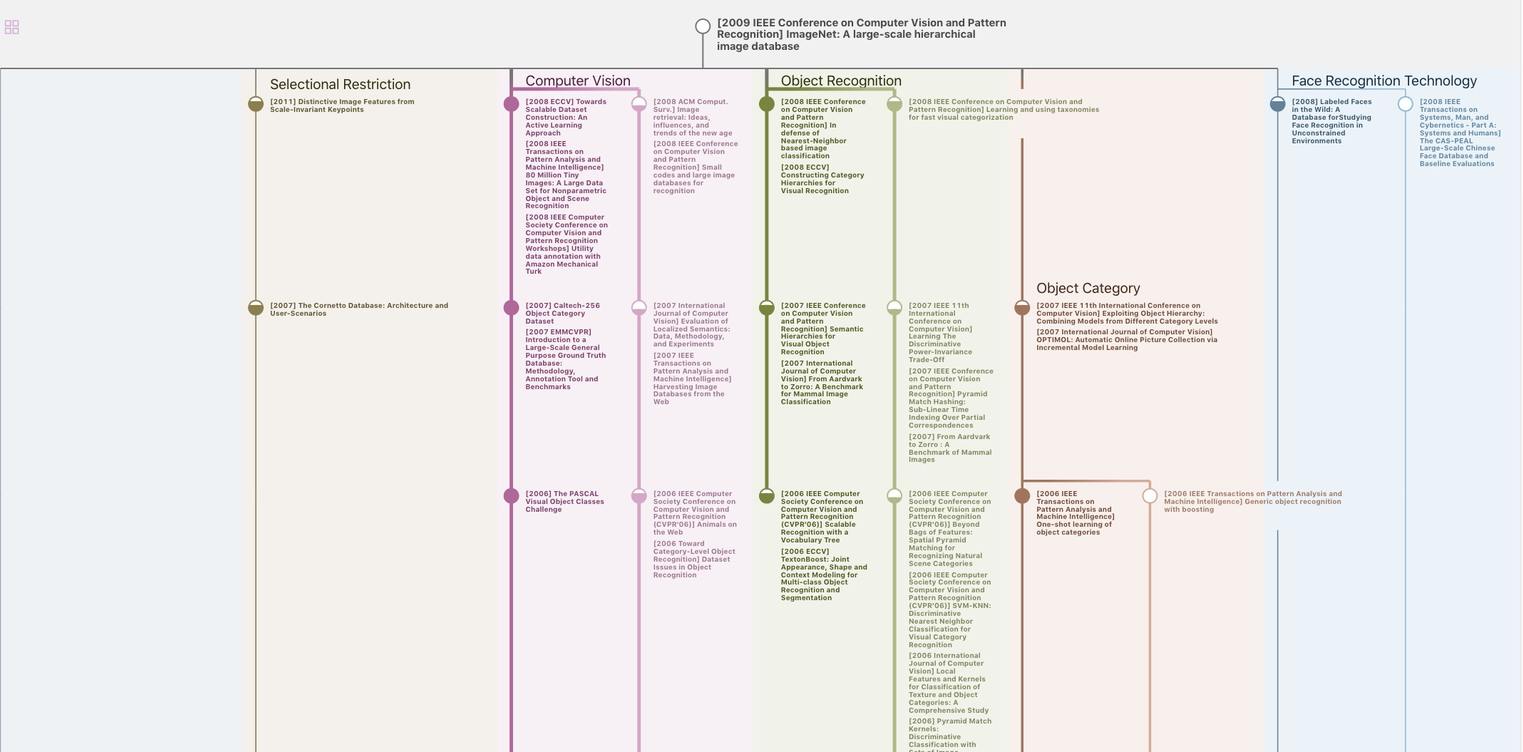
生成溯源树,研究论文发展脉络
Chat Paper
正在生成论文摘要