Learning Ising models from one or multiple samples
ACM Symposium on Theory of Computing(2021)
摘要
ABSTRACTThere have been two main lines of work on estimating Ising models: (1) estimating them from multiple independent samples under minimal assumptions about the model's interaction matrix ; and (2) estimating them from one sample in restrictive settings. We propose a unified framework that smoothly interpolates between these two settings, enabling significantly richer estimation guarantees from one, a few, or many samples. Our main theorem provides guarantees for one-sample estimation, quantifying the estimation error in terms of the metric entropy of a family of interaction matrices. As corollaries of our main theorem, we derive bounds when the model's interaction matrix is a (sparse) linear combination of known matrices, or it belongs to a finite set, or to a high-dimensional manifold. In fact, our main result handles multiple independent samples by viewing them as one sample from a larger model, and can be used to derive estimation bounds that are qualitatively similar to those obtained in the afore-described multiple-sample literature. Our technical approach benefits from sparsifying a model's interaction network, conditioning on subsets of variables that make the dependencies in the resulting conditional distribution sufficiently weak. We use this sparsification technique to prove strong concentration and anti-concentration results for the Ising model, which we believe have applications beyond the scope of this paper.
更多查看译文
关键词
Ising Model,Pseudo-Likelihood,Concentration Inequalities,Low Temperature,Single-Sample Estimation,Dependent Data
AI 理解论文
溯源树
样例
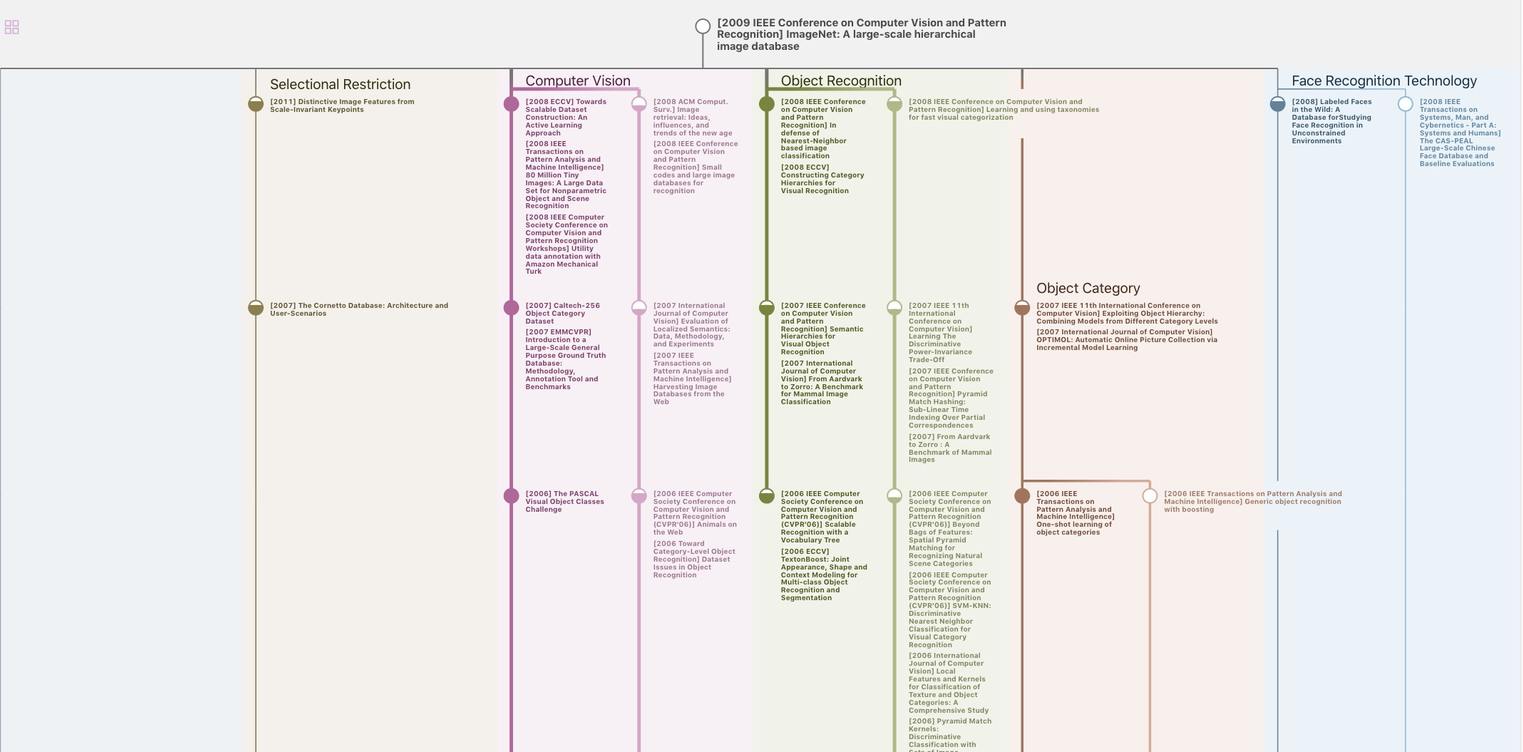
生成溯源树,研究论文发展脉络
Chat Paper
正在生成论文摘要