Doubly Robust Feature Selection with Mean and Variance Outlier Detection and Oracle Properties
arxiv(2021)
摘要
We propose a general approach to handle data contaminations that might disrupt the performance of feature selection and estimation procedures for high-dimensional linear models. Specifically, we consider the co-occurrence of mean-shift and variance-inflation outliers, which can be modeled as additional fixed and random components, respectively, and evaluated independently. Our proposal performs feature selection while detecting and down-weighting variance-inflation outliers, detecting and excluding mean-shift outliers, and retaining non-outlying cases with full weights. Feature selection and mean-shift outlier detection are performed through a robust class of nonconcave penalization methods. Variance-inflation outlier detection is based on the penalization of the restricted posterior mode. The resulting approach satisfies a robust oracle property for feature selection in the presence of data contamination -- which allows the number of features to exponentially increase with the sample size -- and detects truly outlying cases of each type with asymptotic probability one. This provides an optimal trade-off between a high breakdown point and efficiency. Computationally efficient heuristic procedures are also presented. We illustrate the finite-sample performance of our proposal through an extensive simulation study and a real-world application.
更多查看译文
关键词
robust feature selection,feature selection,variance outlier detection
AI 理解论文
溯源树
样例
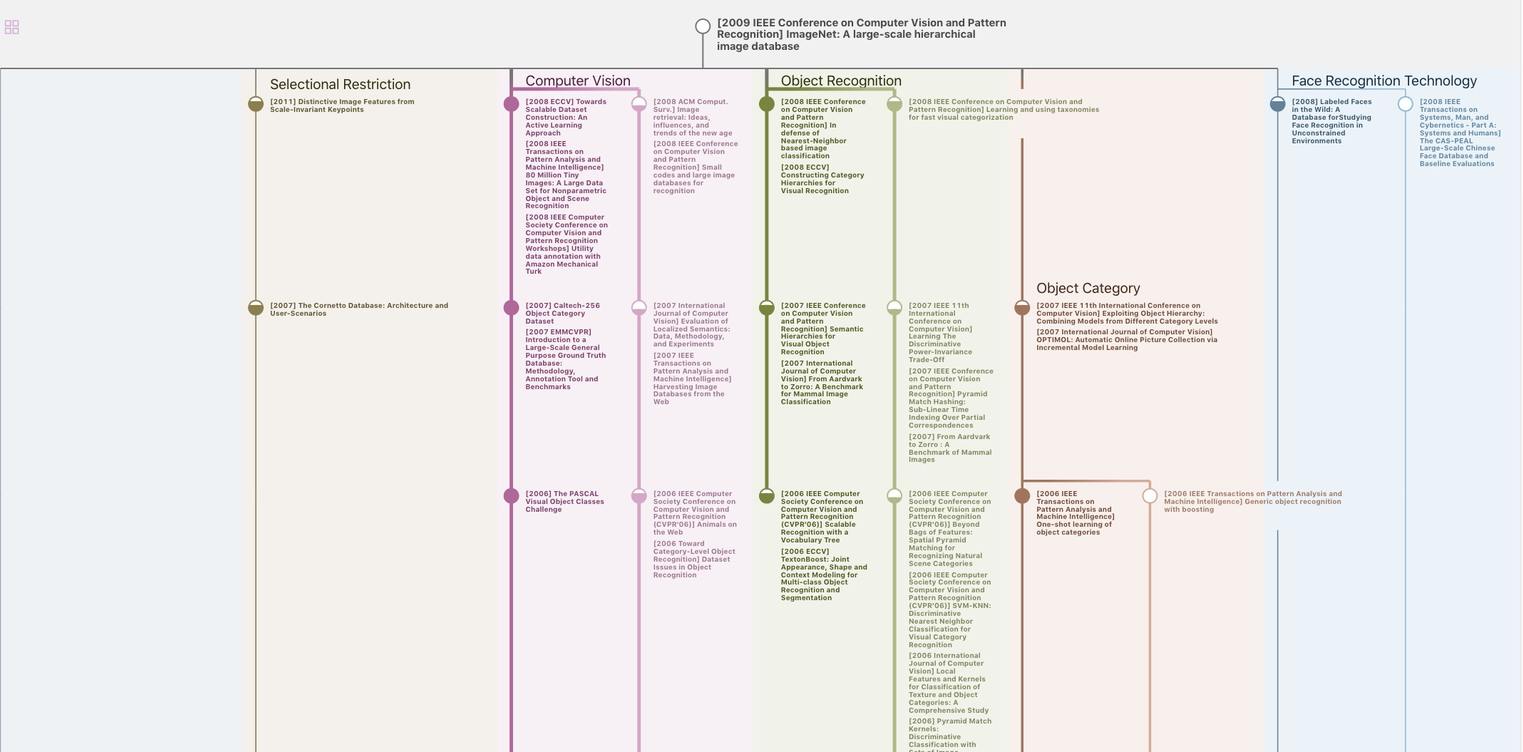
生成溯源树,研究论文发展脉络
Chat Paper
正在生成论文摘要