Imitation Learning: Progress, Taxonomies and Challenges
IEEE TRANSACTIONS ON NEURAL NETWORKS AND LEARNING SYSTEMS(2024)
摘要
Imitation learning (IL) aims to extract knowledge from human experts' demonstrations or artificially created agents to replicate their behaviors. It promotes interdisciplinary communication and real-world automation applications. However, the process of replicating behaviors still exhibits various problems, such as the performance is highly dependent on the demonstration quality, and most trained agents are limited to perform well in task-specific environments. In this survey, we provide an insightful review on IL. We first introduce the background knowledge from development history and preliminaries, followed by presenting different taxonomies within IL and key milestones of the field. We then detail challenges in learning strategies and present research opportunities with learning policy from suboptimal demonstration, voice instructions, and other associated optimization schemes.
更多查看译文
关键词
Behavioral sciences,Task analysis,Taxonomy,Training,Optimization,Games,Entropy,Imitation learning (IL),machine learning,taxonomies
AI 理解论文
溯源树
样例
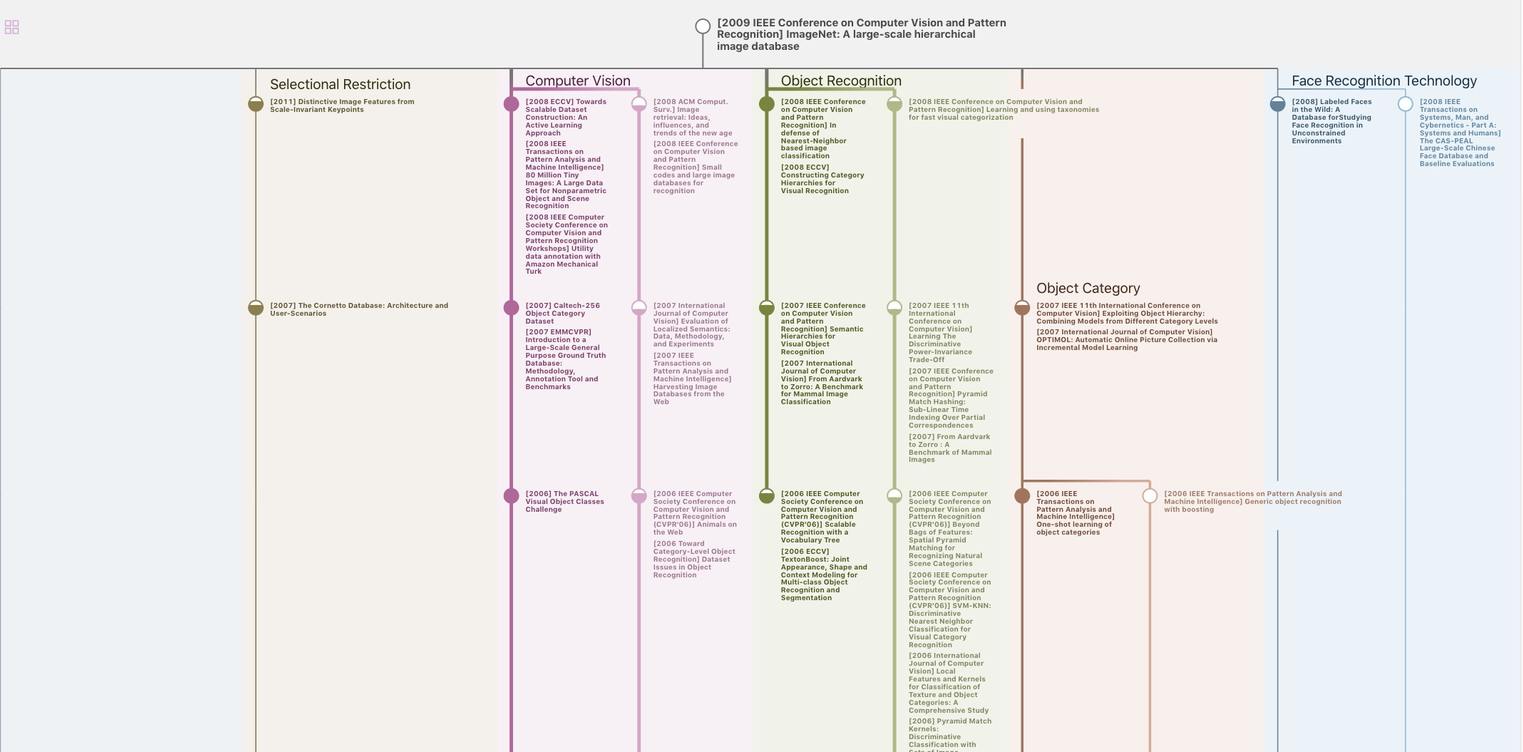
生成溯源树,研究论文发展脉络
Chat Paper
正在生成论文摘要