Real-Time Outdoor Localization Using Radio Maps: A Deep Learning Approach
IEEE TRANSACTIONS ON WIRELESS COMMUNICATIONS(2023)
摘要
Global Navigation Satellite Systems typically perform poorly in urban environments, where the likelihood of line-of-sight conditions between devices and satellites is low. Therefore, alternative location methods are required to achieve good accuracy. We present LocUNet: A convolutional, end-to-end trained neural network (NN) for the localization task, which is able to estimate the position of a user from the received signal strength (RSS) of a small number of Base Stations (BS). Using estimations of pathloss radio maps of the BSs and the RSS measurements of the users to be localized, LocUNet can localize users with state-of-the-art accuracy and enjoys high robustness to inaccuracies in the estimations of radio maps. The proposed method does not require generating RSS fingerprints of each specific area where the localization task is performed and is suitable for real-time applications. Moreover, two novel datasets that allow for numerical evaluations of RSS and ToA methods in realistic urban environments are presented and made publicly available for the research community. By using these datasets, we also provide a fair comparison of state-of-the-art RSS and ToA-based methods in the dense urban scenario and show numerically that LocUNet outperforms all the compared methods.
更多查看译文
关键词
Location awareness,Fingerprint recognition,Wireless communication,Estimation,Urban areas,Standards,Global navigation satellite system,Wireless localization,radio maps,pathloss,deep learning,dataset
AI 理解论文
溯源树
样例
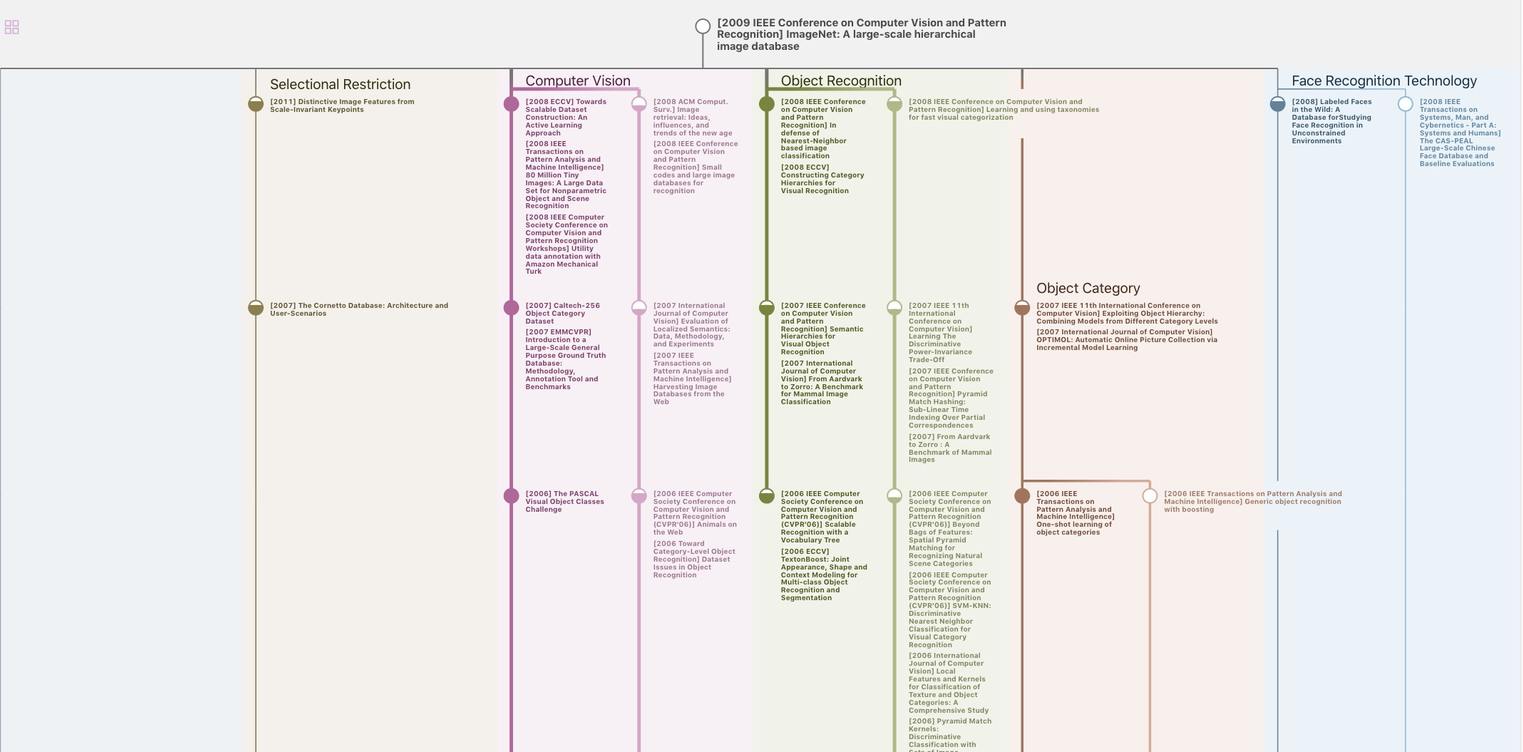
生成溯源树,研究论文发展脉络
Chat Paper
正在生成论文摘要