DeepRecS: From RECIST Diameters to Precise Liver Tumor Segmentation
IEEE Journal of Biomedical and Health Informatics(2022)
摘要
Liver tumor segmentation (LiTS) is of primary importance in diagnosis and treatment of hepatocellular carcinoma. Known automated LiTS methods could not yield satisfactory results for clinical use since they were hard to model flexible tumor shapes and locations. In clinical practice, radiologists usually estimate tumor shape and size by a Response Evaluation Criteria in Solid Tumor (RECIST) mark. Inspired by this, in this paper, we explore a deep learning (DL) based interactive LiTS method, which incorporates guidance from user-provided RECIST marks. Our method takes a three-step framework to predict liver tumor boundaries. Under this architecture, we develop a RECIST mark propagation network (RMP-Net) to estimate RECIST-like marks in off-RECIST slices. We also devise a context-guided boundary-sensitive network (CGBS-Net) to distill tumors’ contextual and boundary information from corresponding RECIST(-like) marks, and then predict tumor maps. To further refine the segmentation results, we process the tumor maps using a 3D conditional random field (CRF) algorithm and a morphology hole-filling operation. Verified on two clinical contrast-enhanced abdomen computed tomography (CT) image datasets, our proposed approach can produce promising segmentation results, and outperforms the state-of-the-art interactive segmentation methods.
更多查看译文
关键词
Deep learning,liver tumor segmentation,interactive segmentation,response evaluation criteria in solid tumor
AI 理解论文
溯源树
样例
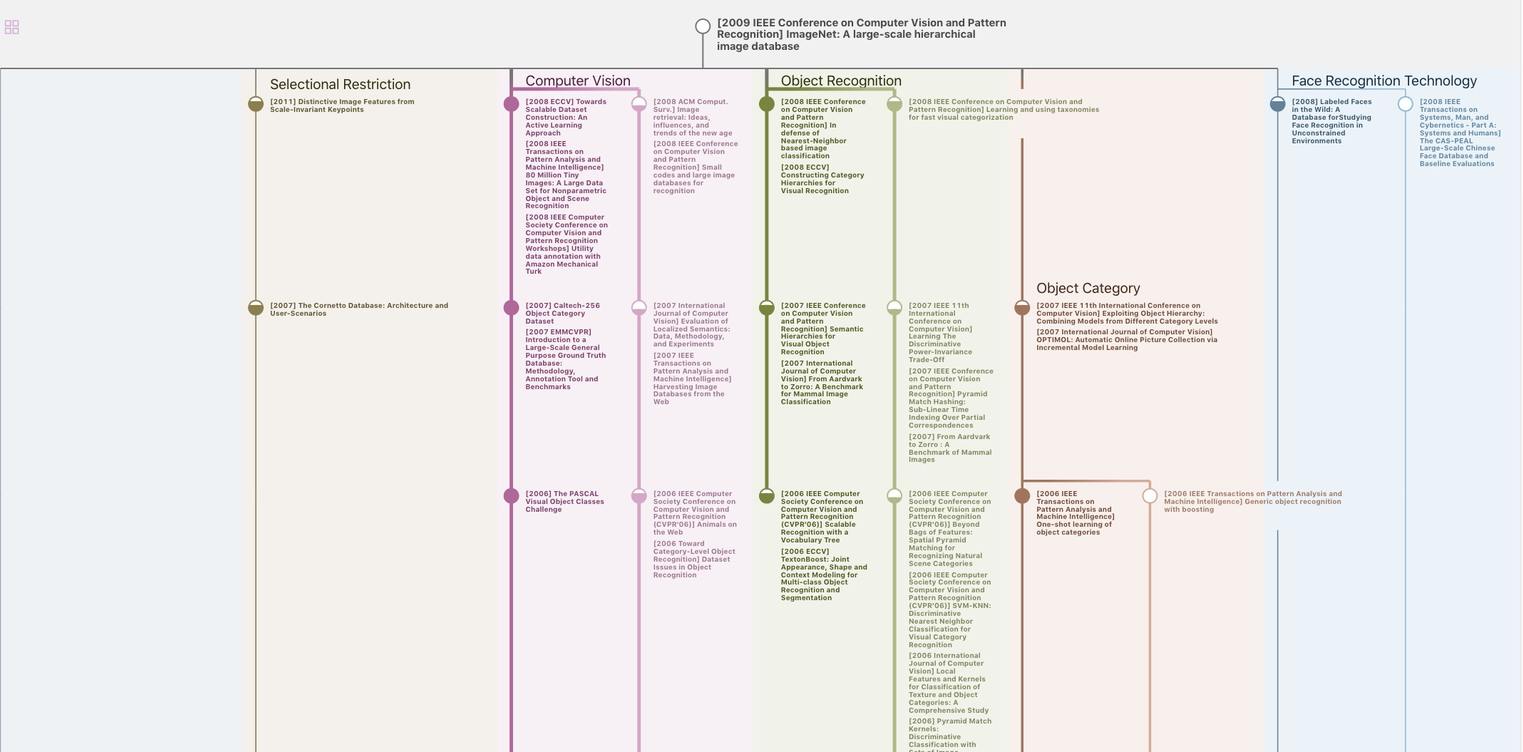
生成溯源树,研究论文发展脉络
Chat Paper
正在生成论文摘要