Video Swin Transformer
IEEE Conference on Computer Vision and Pattern Recognition(2022)
摘要
The vision community is witnessing a modeling shift from CNNs to Transformers, where pure Transformer architectures have attained top accuracy on the major video recognition benchmarks. These video models are all built on Transformer layers that globally connect patches across the spatial and temporal dimensions. In this paper, we instead advocate an inductive bias of locality in video Transformers, which leads to a better speed-accuracy trade-off compared to previous approaches which compute self-attention globally even with spatial-temporal factorization. The locality of the proposed video architecture is realized by adapting the Swin Transformer designed for the image domain, while continuing to leverage the power of pre-trained image models. Our approach achieves state-of-the-art accuracy on a broad range of video recognition benchmarks, including on action recognition (84.9 top-l accuracy on Kinetics-400 and 85.9 top-l accuracy on Kinetics-600 with ~20× less pre-training data and ~3× smaller model size) and temporal modeling (69.6 top-l accuracy on Something-Something v2).
更多查看译文
关键词
retrieval,categorization,Video analysis and understanding, Recognition: detection
AI 理解论文
溯源树
样例
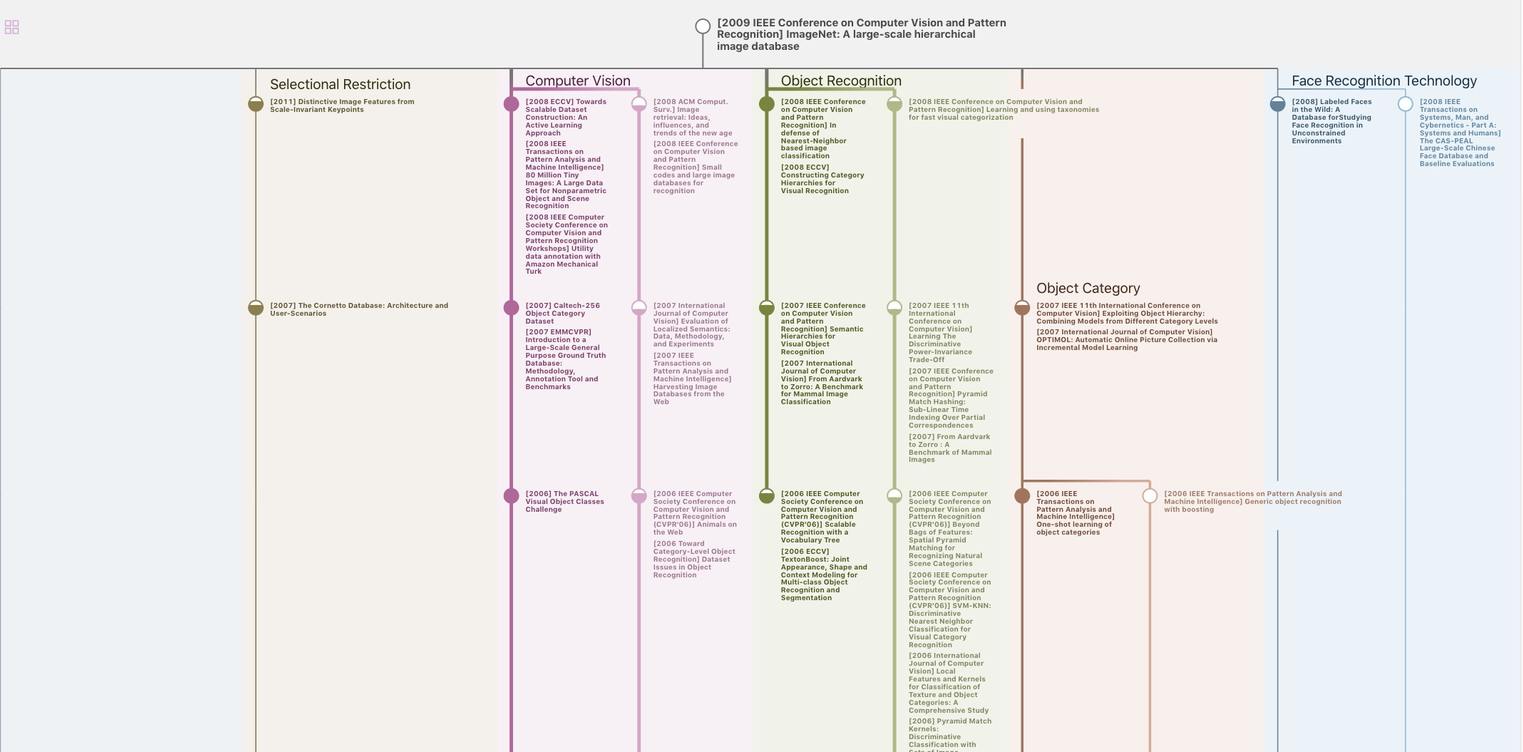
生成溯源树,研究论文发展脉络
Chat Paper
正在生成论文摘要