Realistic utility functions prove difficult for state-of-the-art interactive multiobjective optimization algorithms
Genetic and Evolutionary Computation Conference(2021)
摘要
ABSTRACTImprovements to the design of interactive Evolutionary Multiobjective Algorithms (iEMOAs) are unlikely without quantitative assessment of their behaviour in realistic settings. Experiments with human decision-makers (DMs) are of limited scope due to the difficulty of isolating individual biases and replicating the experiment with enough subjects, and enough times, to obtain confidence in the results. Simulation studies may help to overcome these issues, but they require the use of realistic simulations of decision-makers. Machine decision-makers (MDMs) provide a way to carry out such simulation studies, however, studies so far have relied on simple utility functions. In this paper, we analyse and compare two state-of-the-art iEMOAs by means of a MDM that uses a sigmoid-shaped utility function. This sigmoid utility function is based on psychologically realistic models from behavioural economics, and replicates several realistic human behaviours. Our findings are that, on a variety of well-known benchmarks with two and three objectives, the two iEMOAs do not consistently recover the most-preferred points. We hope that these findings provide an impetus for more directed design and analysis of future iEMOAs.
更多查看译文
关键词
Interactive Evolutionary Multi-Objective Optimization, Design of Experiments, Machine Decision Maker
AI 理解论文
溯源树
样例
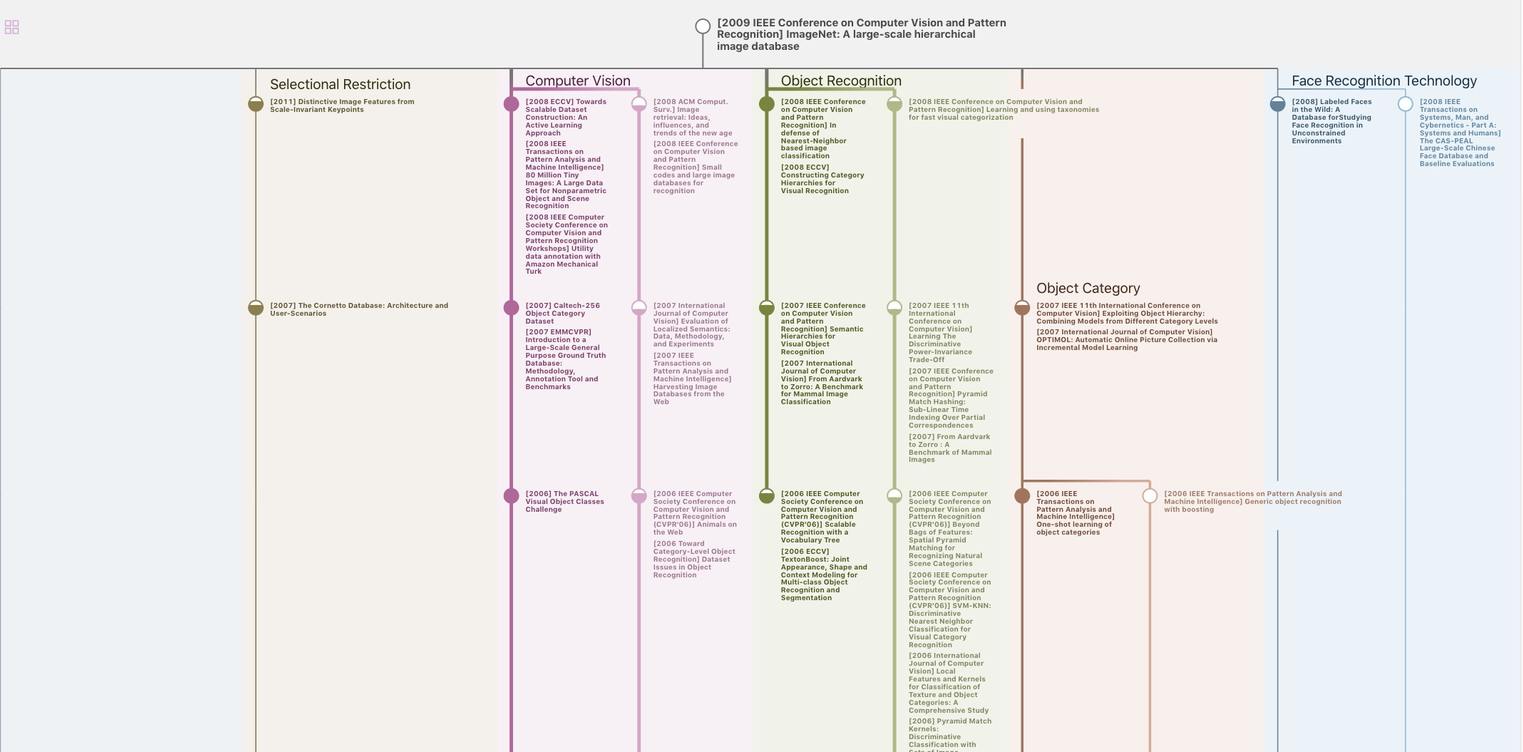
生成溯源树,研究论文发展脉络
Chat Paper
正在生成论文摘要