Optimal Sampling Rates for Reliable Continuous-Time First-Order Autoregressive and Vector Autoregressive Modeling
PSYCHOLOGICAL METHODS(2021)
摘要
Translational Abstract Autoregressive and vector autoregressive models are a driving force in current psychological research. In affect research they are, for instance, frequently used to describe affective processes and how these evolve over time. Model variants that estimate processes over discrete time points are most commonly used, but continuous-time formulations are gaining popularity. They allow to handle data from longitudinal studies in which participants were assessed at irregular intervals, and to compare results across data sets obtained at different sampling rates. However, continuous-time models are still estimated from data sampled at discrete time points. Whether and how the data's sampling rate affects how well such continuous-time models can be estimated, has largely been ignored in the literature. In the present article, we show how the sampling rate affects the estimation reliability (i.e., the standard errors of the parameter estimators, with smaller values indicating higher reliability) of continuous-time autoregressive and vector autoregressive models. Moreover, we determine which sampling rates are optimal for estimating the models, in the sense that they lead to standard errors of minimal size. We illustrate our results with real data from the COGITO Study. We make recommendations for study planning, and elaborate on strengths and limitations of our approach. Autoregressive and vector autoregressive models are a driving force in current psychological research. In affect research they are, for instance, frequently used to formalize affective processes and estimate affective dynamics. Discrete-time model variants are most commonly used, but continuous-time formulations are gaining popularity, because they can handle data from longitudinal studies in which the sampling rate varies within the study period, and yield results that can be compared across data sets from studies with different sampling rates. However, whether and how the sampling rate affects the quality with which such continuous-time models can be estimated, has largely been ignored in the literature. In the present article, we show how the sampling rate affects the estimation reliability (i.e., the standard errors of the parameter estimators, with smaller values indicating higher reliability) of continuous-time autoregressive and vector autoregressive models. Moreover, we determine which sampling rates are optimal in the sense that they lead to standard errors of minimal size (subject to the assumption that the models are correct). Our results are based on the theories of optimal design and maximum likelihood estimation. We illustrate them making use of data from the COGITO Study. We formulate recommendations for study planning, and elaborate on strengths and limitations of our approach.
更多查看译文
关键词
continuous time first-order vector autoregressive modeling, estimation reliability, maximum likelihood estimation, sampling rate, optimal design
AI 理解论文
溯源树
样例
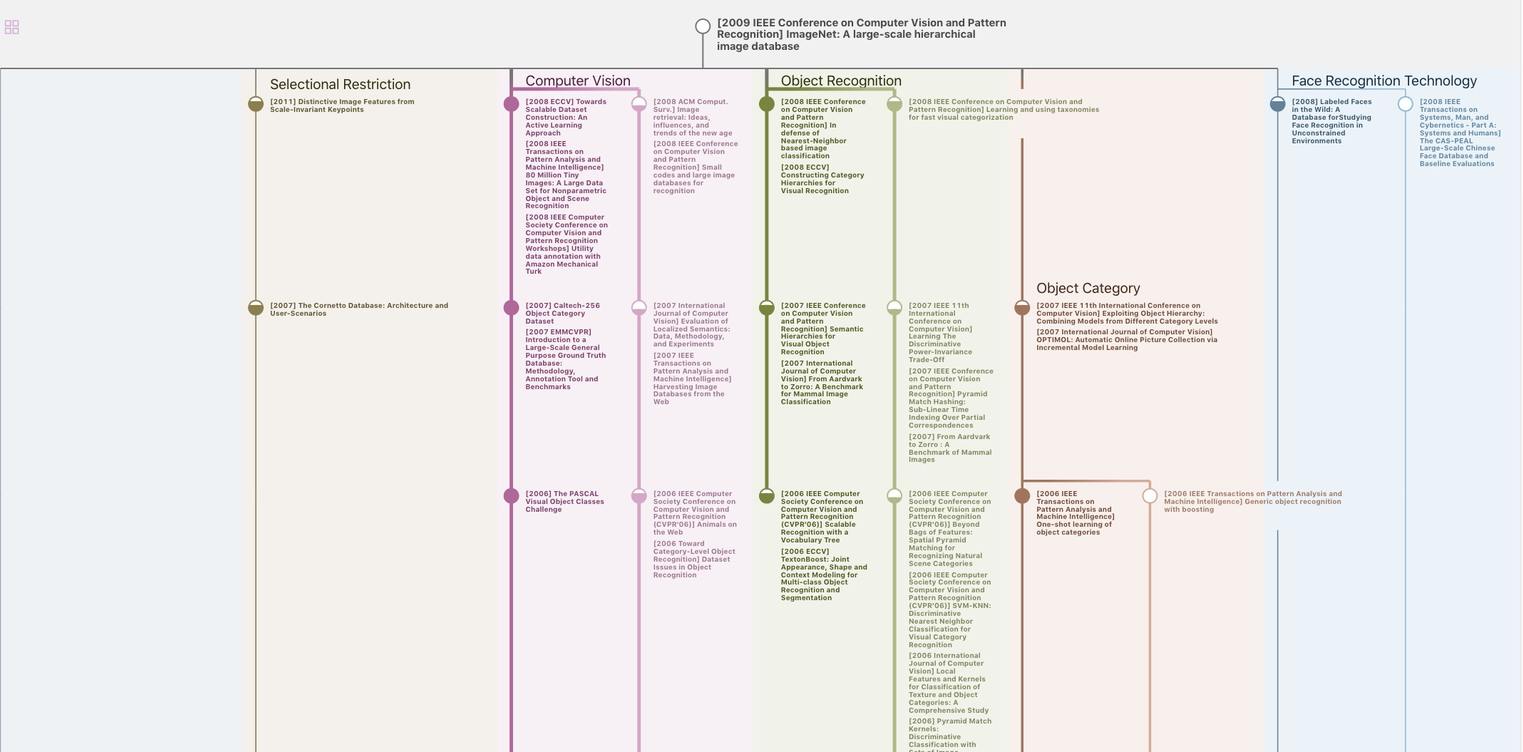
生成溯源树,研究论文发展脉络
Chat Paper
正在生成论文摘要