Automated segmentation and quantification of the healthy and diseased aorta in CT angiographies using a dedicated deep learning approach
European Radiology(2021)
摘要
Objectives To develop and validate a deep learning–based algorithm for segmenting and quantifying the physiological and diseased aorta in computed tomography angiographies. Methods CTA exams of the aorta of 191 patients (68.1 ± 14 years, 128 male), performed between 2015 and 2018, were retrospectively identified from our imaging archive and manually segmented by two investigators. A 3D U-Net model was trained on the data, which was divided into a training, a validation, and a test group at a ratio of 7:1:2. Cases in the test group (n = 41) were evaluated to compare manual and automatic segmentations. Dice similarity coefficient (DSC), mean surface distance (MSD), and Hausdorff surface distance (HSD) were extracted. Maximum diameter, effective diameter, and area were quantified and compared between both segmentations at eight anatomical landmarks, and at the maximum area of an aneurysms if present (n = 14). Statistics included error calculation, intraclass correlation coefficient, and Bland-Altman analysis. Results A DSC of 0.95 [0.94; 0.95] and an MSD of 0.76 [0.06; 0.99] indicated close agreement between segmentations. HSD was 8.00 [4.47; 10.00]. The largest absolute errors were found in the ascending aorta with 0.8 ± 1.5 mm for maximum diameter and at the coeliac trunk with − 30.0 ± 81.6 mm 2 for area. Results for absolute errors in aneurysms were − 0.5 ± 2.3 mm for maximum diameter, 0.3 ± 1.6 mm for effective diameter, and 64.9 ± 114.9 mm 2 for area. ICC showed excellent agreement (> 0.9; p < 0.05) between quantitative measurements. Conclusions Automated segmentation of the aorta on CTA data using a deep learning algorithm is feasible and allows for accurate quantification of the aortic lumen even if the vascular architecture is altered by disease. Key Points • A deep learning–based algorithm can automatically segment the aorta, mostly within acceptable margins of error, even if the vascular architecture is altered by disease. • Quantifications performed in the segmentations were mostly within clinically acceptable limits, even in pathologically altered segments of the aorta.
更多查看译文
关键词
Artificial intelligence,Neuronal networks,Image processing,Computer-assisted, Aorta
AI 理解论文
溯源树
样例
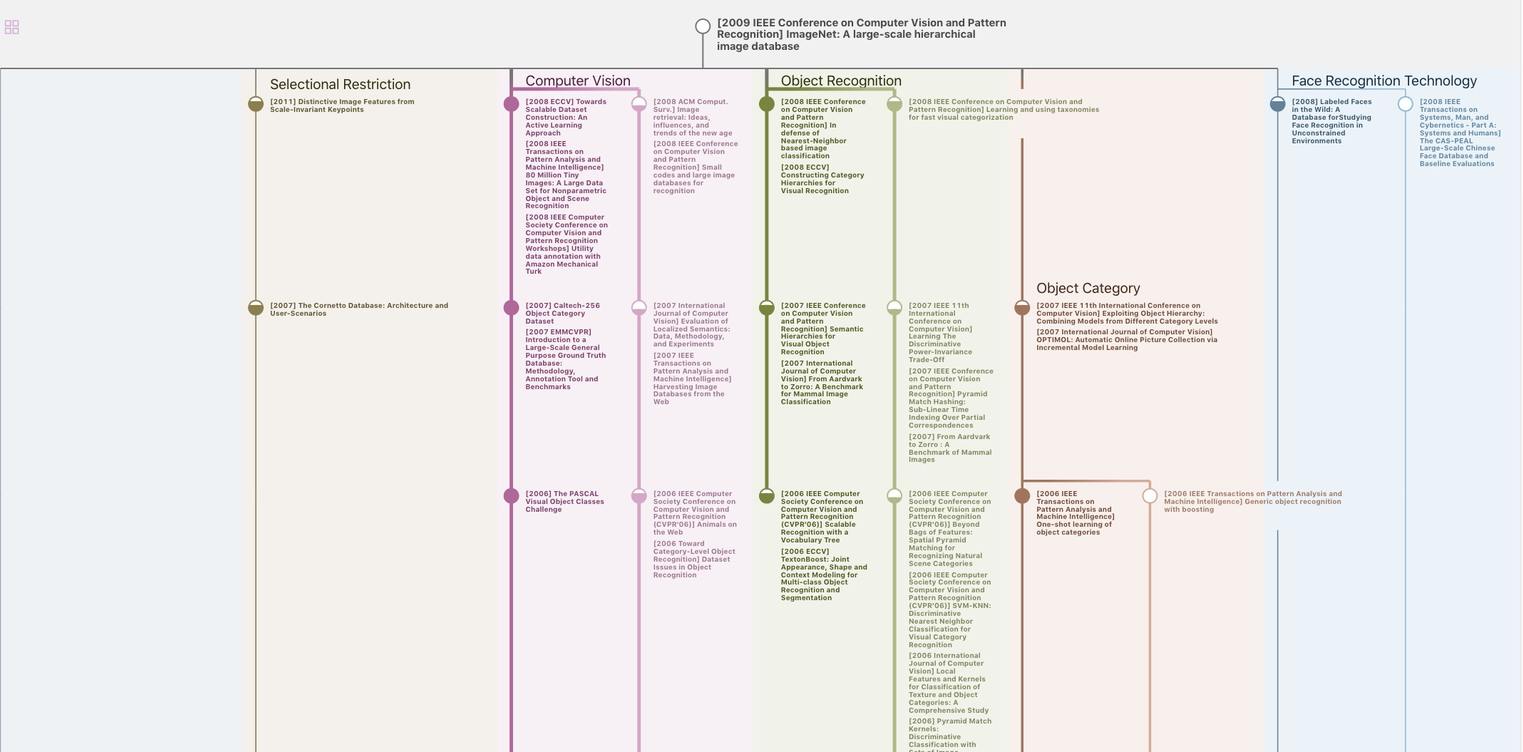
生成溯源树,研究论文发展脉络
Chat Paper
正在生成论文摘要