Turbo: Fraud Detection in Deposit-free Leasing Service via Real-Time Behavior Network Mining
2021 IEEE 37TH INTERNATIONAL CONFERENCE ON DATA ENGINEERING (ICDE 2021)(2021)
摘要
Online deposit-free leasing service has witnessed rapid growth in China and shows a promising market in the future. While eliminating the requirement of a deposit does attract more users to the service, it also lowers the cost for fraudsters. Since the emergence of this service is relatively new, there are few works in literature focusing on detecting fraud transactions in it. Existing efforts mainly fall into hard-coded solutions such as block-listing or scorecard methods, which can be impotent in the face of the diverse fraud tactics, e.g., identity theft, or even suffering concept drift problem as the tactics evolve. In this paper, we contribute Turbo, an efficient graph-based anti-fraud system, to fully exploit the abundant user behavior logs in a real-time manner. Turbo is able to additionally make use of the implicit user relationships beyond the user features in the logs. To capture the user relationships, we first propose a novel algorithm to construct a time-evolving user behavior network called BN. Empirical analysis demonstrates that fraudsters in BN exhibit unique temporal aggregation and homophilic patterns, which inspires us to develop a novel heterogeneous adaptive graph neural network algorithm called HAG. Specifically, in HAG two graph operators are presented to mitigate the over-smoothing problem and make better use of the heterogeneous behavior relations in BN. Extensive experiments on a real-world dataset show that our method outperforms state-of-the-art methods significantly and can give a response in seconds for each detection request.
更多查看译文
AI 理解论文
溯源树
样例
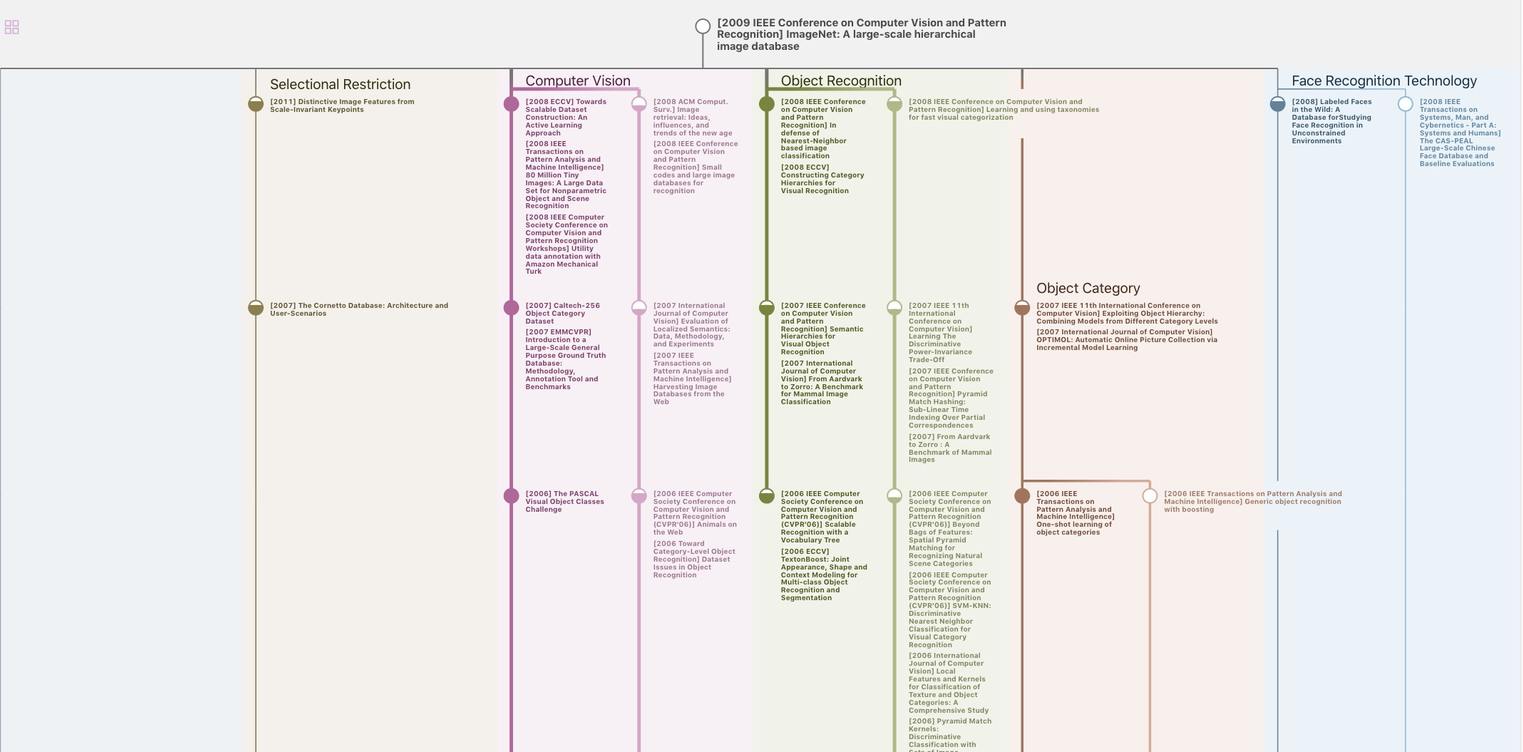
生成溯源树,研究论文发展脉络
Chat Paper
正在生成论文摘要