Palette: Towards Multi-Source Model Selection And Ensemble For Reuse
2021 IEEE 37TH INTERNATIONAL CONFERENCE ON DATA ENGINEERING (ICDE 2021)(2021)
摘要
The success of deep learning presents exciting opportunities for reusing pre-trained models from source domains to solve a target task with possibly limited training data. While various pre-trained models have been developed and become available, there is no principled way to select appropriate models for reuse. Although it is suggested that source and target tasks should be sufficiently similar, the calculation of task relevance usually requires extra storage of source training data and great efforts from domain experts, which is impractical in many applications. In this paper, we study the Multi-source Model Selection and Ensemble (MSMSE) problem. Given a collection of source models, we aim to select a subset of source models and develop an ensemble model that achieves the best performance for a target task. An ensemble of multiple models enables a boarder utilization of various underlying source knowledge and leads to better generalization ability. To this end, we present Palette, a generic framework that first selects potentially well-performed models from a source model pool, and then builds an ensemble with refinement. We introduce different model selection strategies that combine multi-armed bandits with adaptive resource allocation and Bayesian optimization techniques to accelerate the selection process. Extensive experimental results validate the effectiveness and efficiency of Palette and our model selection strategies.
更多查看译文
关键词
model selection, model ensemble, model reuse
AI 理解论文
溯源树
样例
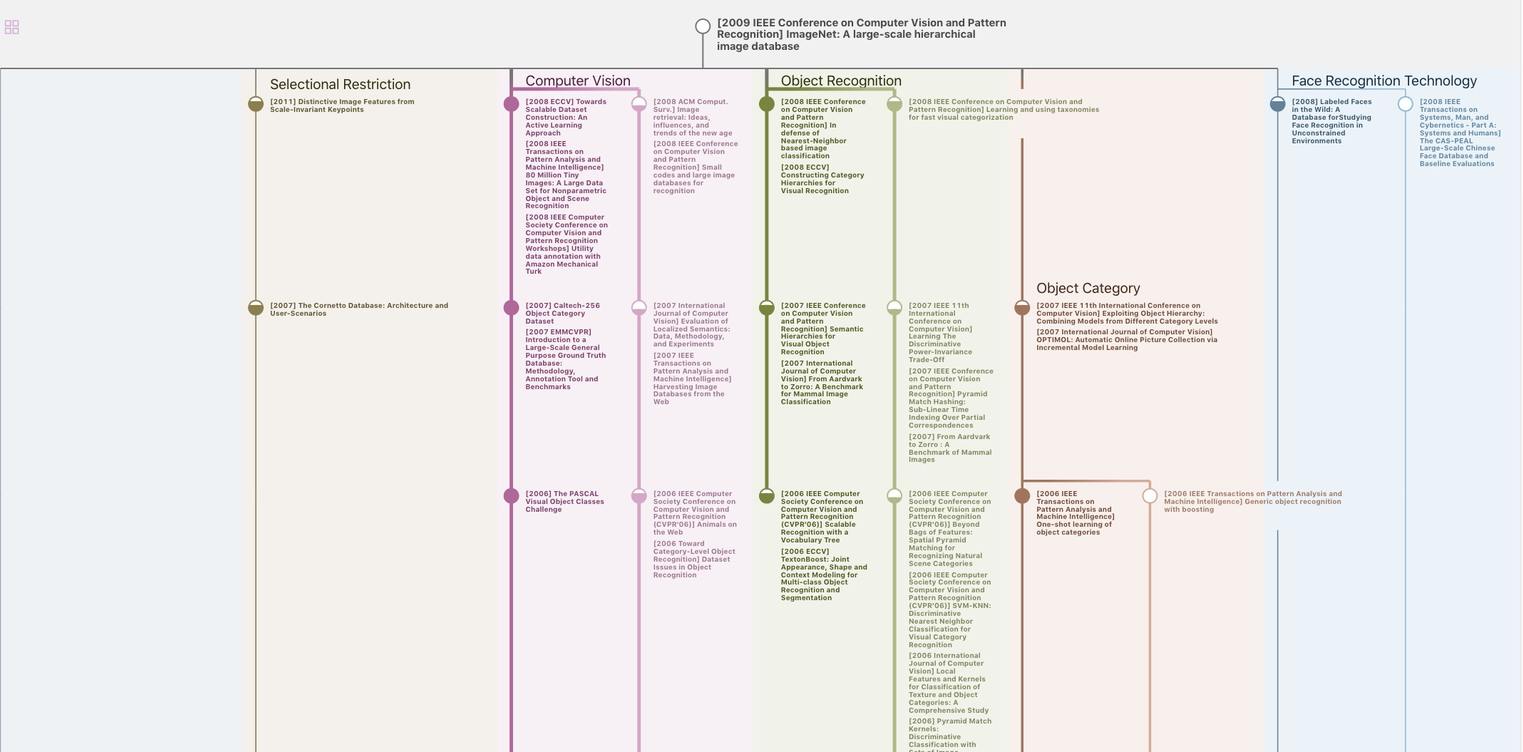
生成溯源树,研究论文发展脉络
Chat Paper
正在生成论文摘要