Multi-Behavior Enhanced Recommendation with Cross-Interaction Collaborative Relation Modeling
2021 IEEE 37TH INTERNATIONAL CONFERENCE ON DATA ENGINEERING (ICDE 2021)(2021)
摘要
Many previous studies aim to augment collaborative filtering with deep neural network techniques, so as to achieve better recommendation performance. However, most existing deep learning-based recommender systems are designed for modeling singular type of user-item interaction behavior, which can hardly distill the heterogeneous relations between user and item. In practical recommendation scenarios, there exist multi-typed user behaviors, such as browse and purchase. Due to the overlook of user’s multi-behavioral patterns over different items, existing recommendation methods are insufficient to capture heterogeneous collaborative signals from user multi-behavior data. Inspired by the strength of graph neural networks for structured data modeling, this work proposes a Graph Neural Multi-Behavior Enhanced Recommendation (GNMR) framework which explicitly models the dependencies between different types of user-item interactions under a graph-based message passing architecture. GNMR devises a relation aggregation network to model interaction heterogeneity, and recursively performs embedding propagation between neighboring nodes over the user-item interaction graph. Experiments on real-world recommendation datasets show that our GNMR consistently outperforms state-of-the-art methods. The source code is available at https://github.com/akaxlh/GNMR.
更多查看译文
关键词
Recommender Systems, Multi-Behavior Recommendation, Graph Neural Networks
AI 理解论文
溯源树
样例
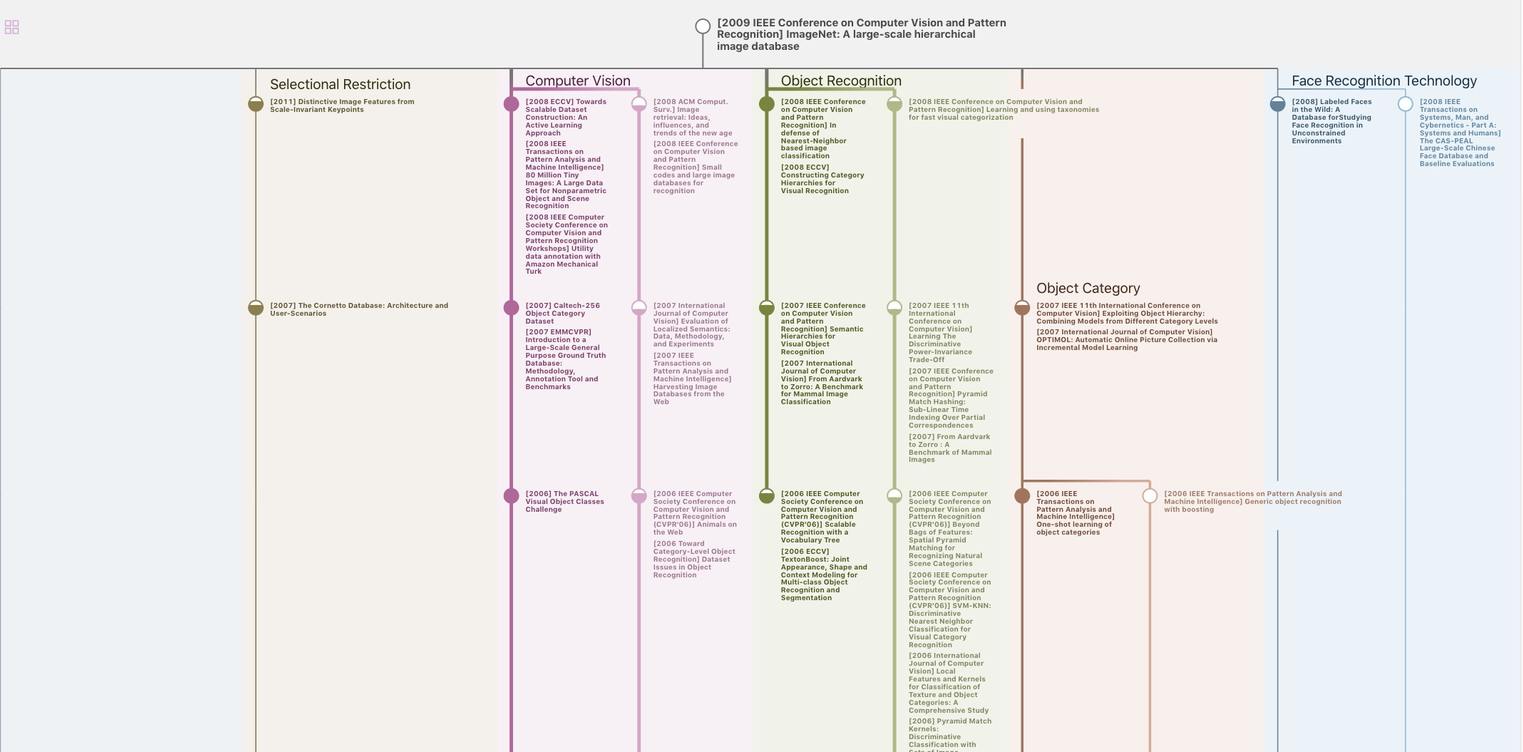
生成溯源树,研究论文发展脉络
Chat Paper
正在生成论文摘要