Geometric Learning of the Conformational Dynamics of Molecules Using Dynamic Graph Neural Networks
arxiv(2021)
摘要
We apply a temporal edge prediction model for weighted dynamic graphs to predict time-dependent changes in molecular structure. Each molecule is represented as a complete graph in which each atom is a vertex and all vertex pairs are connected by an edge weighted by the Euclidean distance between atom pairs. We ingest a sequence of complete molecular graphs into a dynamic graph neural network (GNN) to predict the graph at the next time step. Our dynamic GNN predicts atom-to-atom distances with a mean absolute error of 0.017 Å, which is considered “chemically accurate” for molecular simulations. We also explored the transferability of a trained network to new molecular systems and found that finetuning with less than 10 mean absolute error of the same order of magnitude as that when training from scratch on the full molecular trajectory.
更多查看译文
AI 理解论文
溯源树
样例
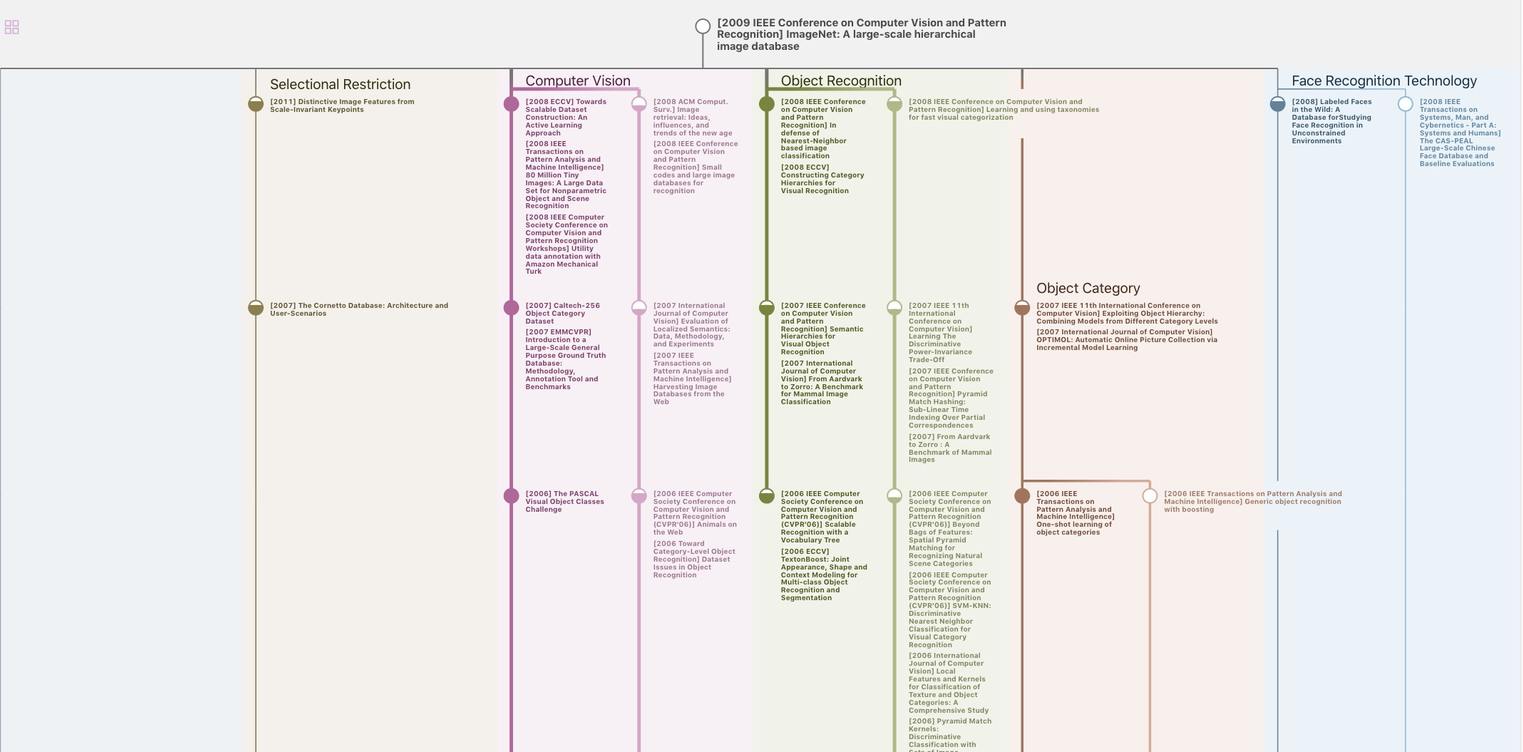
生成溯源树,研究论文发展脉络
Chat Paper
正在生成论文摘要