A Hybrid Model-Based and Learning-Based Approach for Classification Using Limited Number of Training Samples
IEEE open journal of signal processing(2022)
摘要
The fundamental task of classification given a limited number of training data samples is considered for physicalsystems with known parametric statistical models. The standalone learning-based and statistical model-based classifiers face major challenges towards the fulfillment of the classification task using a small training set. Specifically, classifiers that solely rely on the physics-based statistical models usually suffer from their inability to properly tune the underlying unobservable parameters, which leads to a mismatched representation of the system’s behaviors. Learning-based classifiers, on the other hand, typically rely on a large number of training data from the underlying physical process, which might not be feasible in most practical scenarios. In this paper, a hybrid classification method—termed HyPhyLearn —is proposed that exploits both the physics-based statistical models and the learning-based classifiers. The proposed solution is based on the conjecture that HyPhyLearn would alleviate the challenges associated with the individual approaches of learning-based and statistical model-based classifiers by fusing their respective strengths. The proposed hybrid approach first estimates the unobservable model parameters using the available (suboptimal) statistical estimation procedures, and subsequently use the physics-based statistical models to generate synthetic data . Then, the training data samples are incorporated with the synthetic data in a learning-based classifier that is based on domain-adversarial training of neural networks. Specifically, in order to address the mismatch problem, the classifier learns a mapping from the training data and the synthetic data to a common feature space. Simultaneously, the classifier is trained to find discriminative features within this space in order to fulfill the classification task. Two case studies from communications systems (physical layer security and multi-user detection) are presented in order to highlight the usefulness of HyPhyLearn . Numerical results demonstrate that the proposed approach leads to major classification improvements in comparison to the existing standalone or hybrid classification methods.
更多查看译文
关键词
Classification algorithms,data models,deep learning,wireless communication,physical layer security
AI 理解论文
溯源树
样例
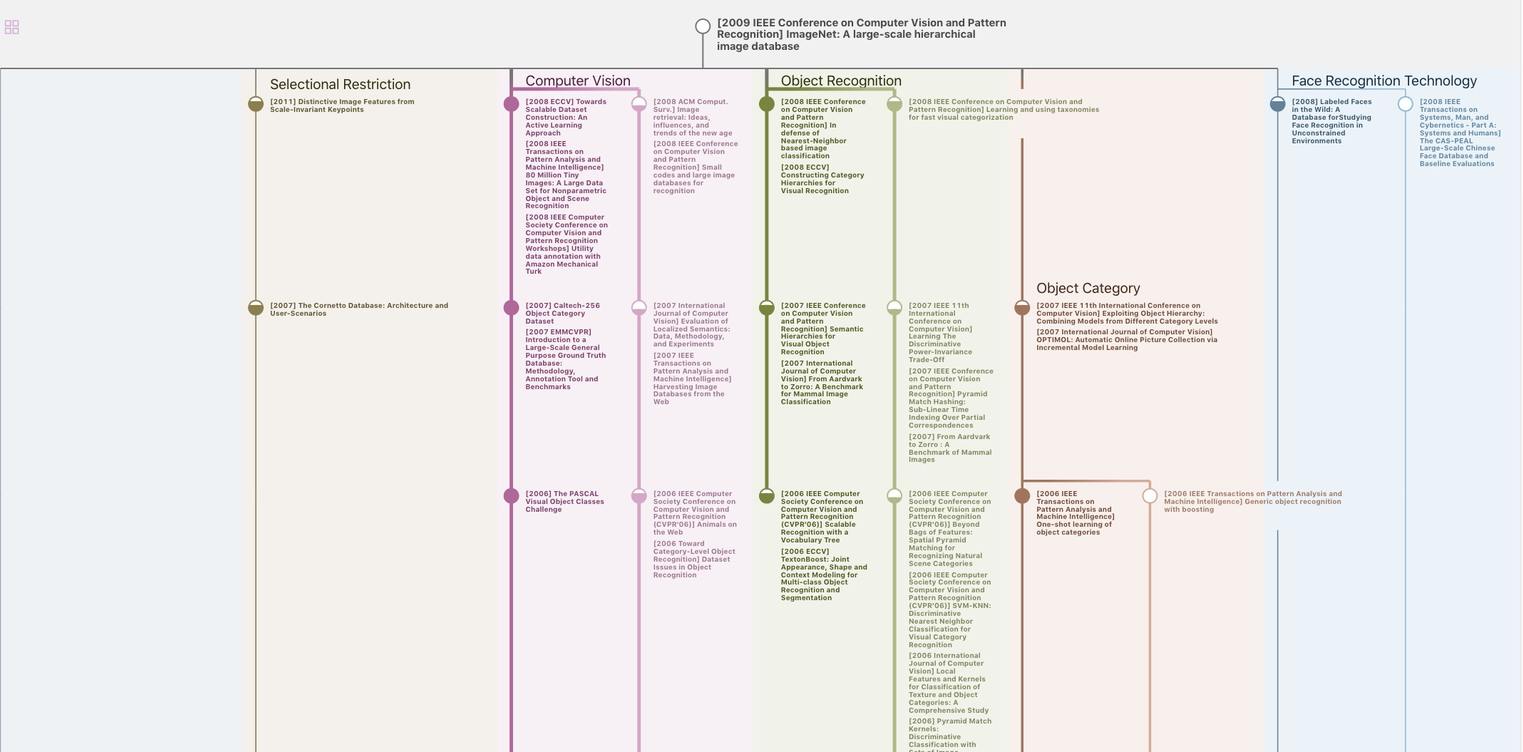
生成溯源树,研究论文发展脉络
Chat Paper
正在生成论文摘要