Text-based Person Search via Multi-Granularity Embedding Learning.
IJCAI(2021)
摘要
Most existing text-based person search methods highly depend on exploring the corresponding relations between the regions of the image and the words in the sentence. However, these methods correlated image regions and words in the same semantic granularity. It 1) results in irrelevant corresponding relations between image and text, 2) causes an ambiguity embedding problem. In this study, we propose a novel multi-granularity embedding learning model for text-based person search. It generates multi-granularity embeddings of partial person bodies in a coarse-to-fine manner by revisiting the person image at different spatial scales. Specifically, we distill the partial knowledge from image scrips to guide the model to select the semantically relevant words from the text description. It can learn discriminative and modality-invariant visual-textual embeddings. In addition, we integrate the partial embeddings at each granularity and perform multi-granularity image-text matching. Extensive experiments validate the effectiveness of our method, which can achieve new state-of-the-art performance by the learned discriminative partial embeddings.
更多查看译文
关键词
person search,text-based,multi-granularity
AI 理解论文
溯源树
样例
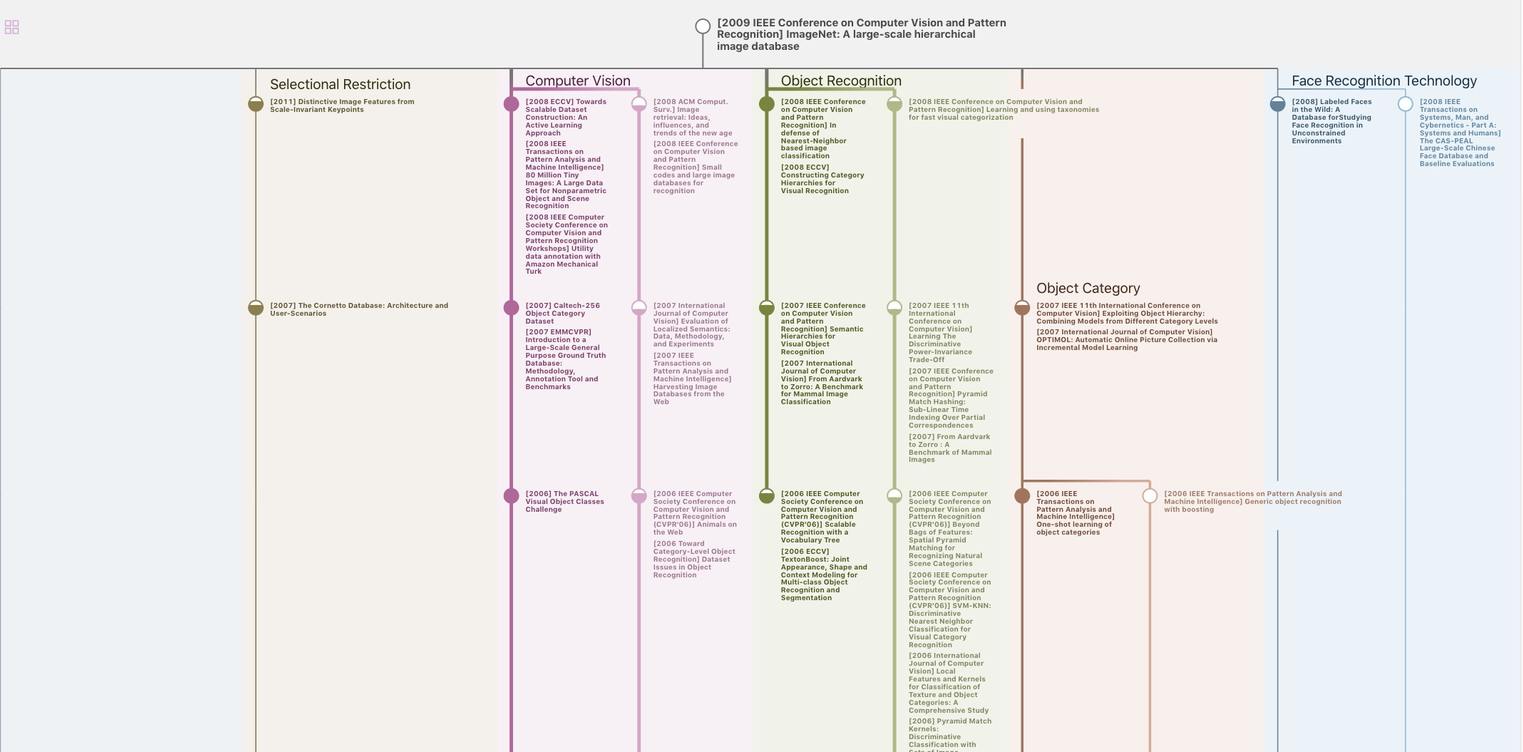
生成溯源树,研究论文发展脉络
Chat Paper
正在生成论文摘要