Overview of the Mowjaz Multi-Topic Labelling Task
International Conference on Information, Communications and Signal Processing(2021)
摘要
Multilabel text classification is an important task in Natural Language Processing (NLP). One use case of such a task is in categorizing news articles, where each article may belong to one or more classes. In this work, we present the ICICS2021 Mowjaz Multi-Topic Labelling Task. Given a piece of news, systems participating in this task are expected to select its topic(s). The systems are evaluated based on the F1 score measure. In total, 46 teams registered on the task's CodaLab page. Out of them, 28 teams submitted 309 runs. The results are surprisingly high. Moreover, they are very close to each other with all teams having systems achieving F1 scores ranging between 0.7965 and 0.8567. Most of these systems used deep learning models, such as Recurrent Neural Networks (RNN), coupled with pretrained word embeddings such as BERT-based models. Few of them experimented with traditional machine learning models such as Support Vector Machine (SVM) and Naive Bayes (NB).
更多查看译文
关键词
Multi-label Text Classification,SVM,RNN,LSTM,GRU,AraVec,Arabic BERT,AraBERT,GigaBERT
AI 理解论文
溯源树
样例
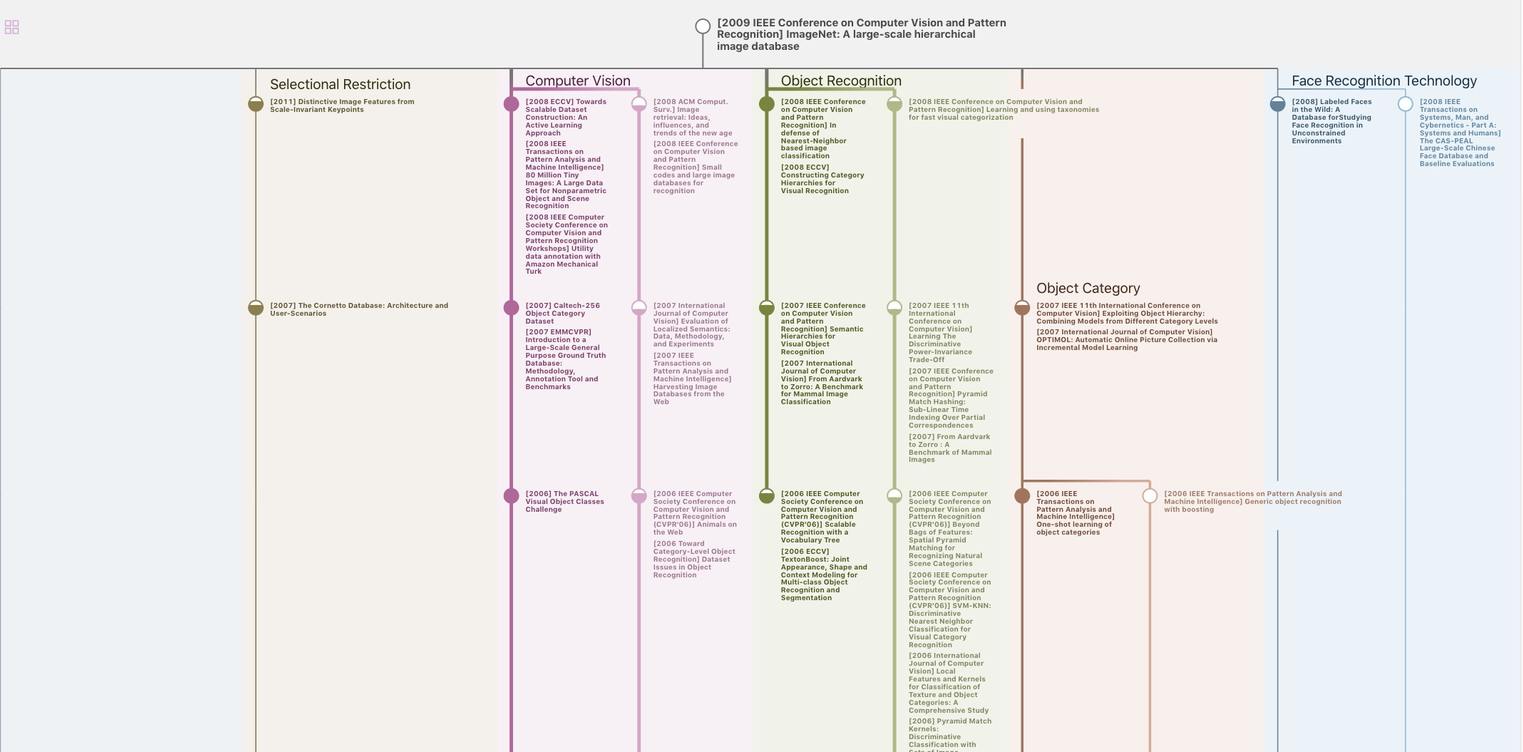
生成溯源树,研究论文发展脉络
Chat Paper
正在生成论文摘要