Integrating topic modeling and word embedding to characterize violent deaths
PROCEEDINGS OF THE NATIONAL ACADEMY OF SCIENCES OF THE UNITED STATES OF AMERICA(2022)
摘要
There is an escalating need for methods to identify latent patterns in text data from many domains. We introduce a method to identify topics in a corpus and represent documents as topic sequences. Discourse atom topic modeling (DATM) draws on advances in theoretical machine learning to integrate topic modeling and word embedding, capitalizing on their distinct capabilities. We first identify a set of vectors ("discourse atoms") that provide a sparse representation of an embedding space. Discourse atoms can be interpreted as latent topics; through a generative model, atoms map onto distributions over words. We can also infer the topic that generated a sequence of words. We illustrate our method with a prominent example of underutilized text: the US National Violent Death Reporting System (NVDRS). The NVDRS summarizes violent death incidents with structured variables and unstructured narratives. We identify 225 latent topics in the narratives (e.g., preparation for death and physical aggression); many of these topics are not captured by existing structured variables. Motivated by known patterns in suicide and homicide by gender and recent research on gender biases in semantic space, we identify the gender bias of our topics (e.g., a topic about pain medication is feminine). We then compare the gender bias of topics to their prevalence in narratives of female versus male victims. Results provide a detailed quantitative picture of reporting about lethal violence and its gendered nature. Our method offers a flexible and broadly applicable approach to model topics in text data.
更多查看译文
关键词
natural language processing, mortality surveillance, gender, topic models, word embeddings
AI 理解论文
溯源树
样例
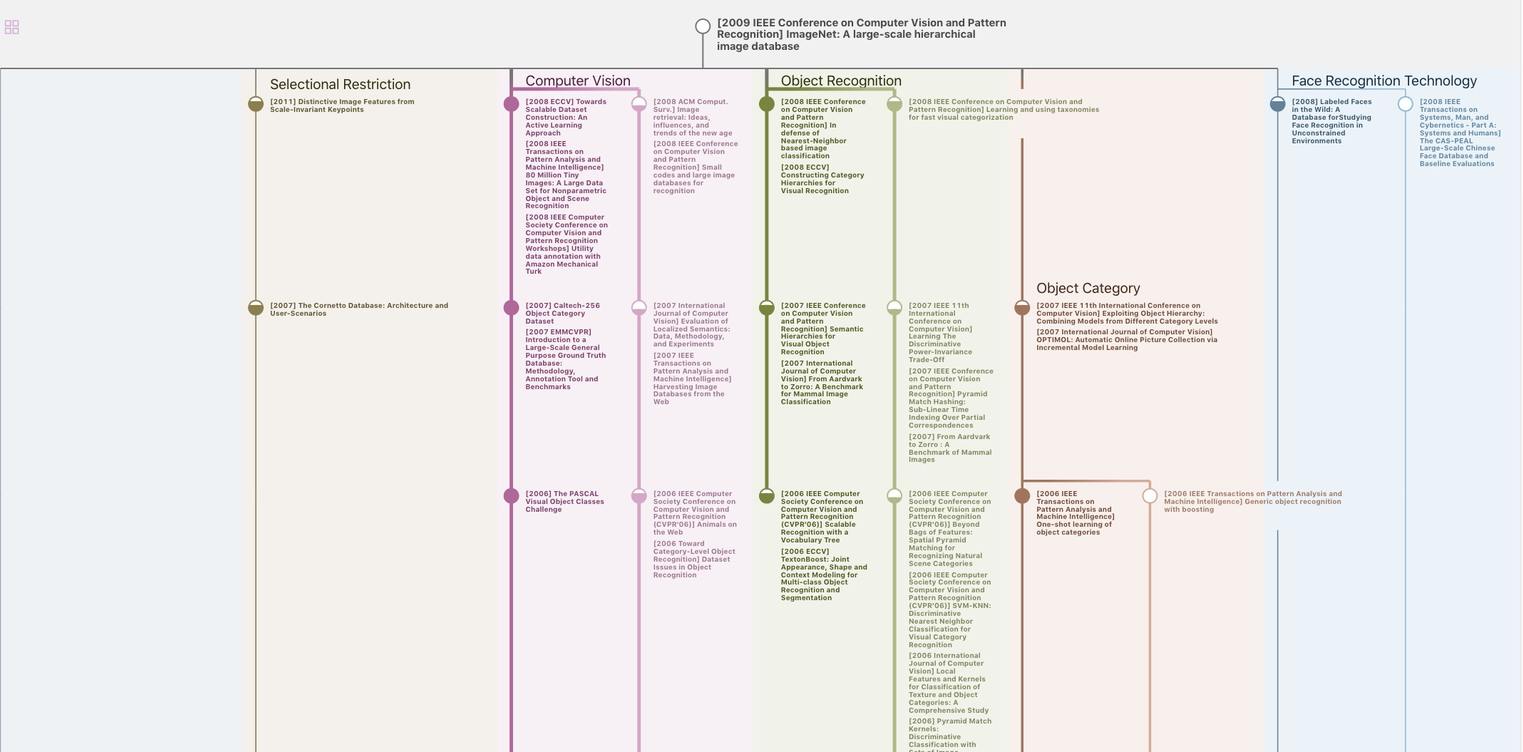
生成溯源树,研究论文发展脉络
Chat Paper
正在生成论文摘要