Dependency-Based Embedding for Distinguishing Between Hate Speech and Offensive Language
WI/IAT(2020)
摘要
The task of detecting online textual hate speech focuses on various objectives with one of the most important being distinguishing between hate speech and generic offensive language. This distinction is very blurry since the two classes are strongly overlapped. Using only keywords for identification falls short because of this overlap, hence the context in which each textual instance exists in, needs to be extracted for increased effectiveness. To achieve this, we investigate the use of embeddings learned with syntactic dependency context (words that have a grammatical relationship with the target word) as opposed to linear context (words that precede and succeed the target word as determined by the window size) in various forms as features for the classification task on a multi-class dataset. Our results and analysis show that for the downstream task of hate speech detection, specifically for distinguishing between hateful and offensive language, the dependency-based embedding has a very comparable performance with its linear-based counterpart even outperforming it in some settings. Moreover, compared to the state-of-the-art (BERT), it demonstrates competitive performance especially when in an ensemble with linear-based embedding. Also, we observed that for a specialised task such as hate speech detection, a domain-specific embedding is probably more important than a large out-of-domain embedding with a larger vocabulary size.
更多查看译文
关键词
hate speech detection,offensive language,text classification,syntactic dependency,dependency based embeddings,word embeddings,convolutional neural networks,bidirectional long short term memory,support vector machines,BERT
AI 理解论文
溯源树
样例
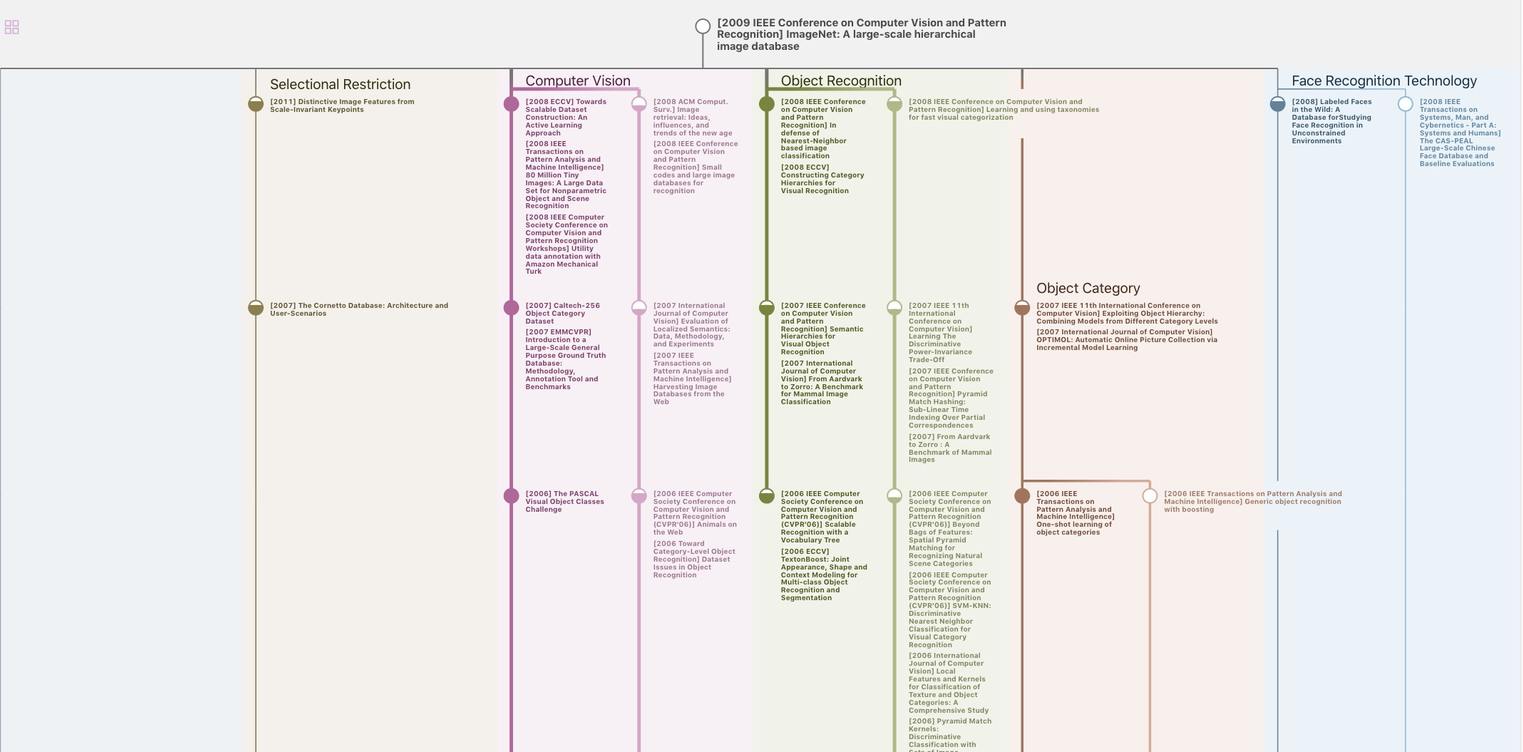
生成溯源树,研究论文发展脉络
Chat Paper
正在生成论文摘要