Recommender Performance on Collaborative Filtering using Latent Topics under Several Sparsity Levels.
WI/IAT(2020)
摘要
Collaborative filtering (CF) is one of the popular methods for selecting contents suited to user's preference. CF represents user's preference as ratings of matrix form. In real systems and services, the percentage of missing ratings (called sparsity) is usually high. Matrix factorization (MF) is an efficient method to estimate missing ratings from a small number of ratings. There is, however, a limit to how well MF can deal with missing ratings. Collaborative topic regression (CTR) is a pioneer model, which uses not only ratings but also document information regarding items and users. CTR is quite effective in extremely high sparsity situations, but its performance may be inferior to that of MF in relatively low sparsity situations. We assume that it is important to select words in the documents based on document frequency according to sparsity. And CTR has several hyperparameters that adjust the influence strength of the ratings and the latent topics extracted from the documents. In this paper, we investigate the impact of the combination of words used in latent topic extraction and hyperparameters under several sparsity levels. These elements are likely to be closely related to each other; hence, it is important to clarify their relationships to leverage CTR. As a result of our experiments, we summarize guidelines for word selection and hyperparameter settings for leveraging CTR according to sparsity.
更多查看译文
关键词
recommender system,collaborative filtering,matrix factorization,topic model
AI 理解论文
溯源树
样例
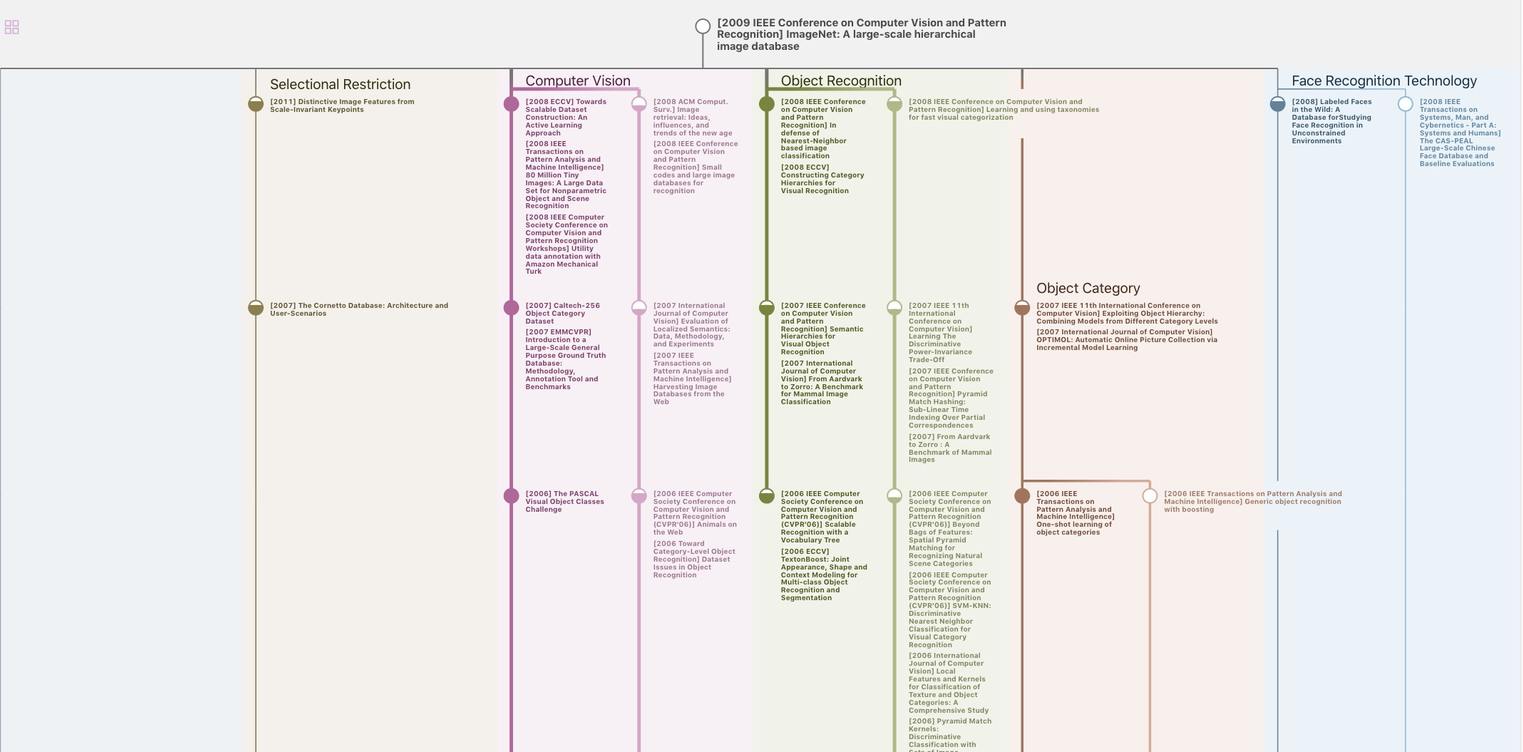
生成溯源树,研究论文发展脉络
Chat Paper
正在生成论文摘要