An Impact Evaluation Framework of Personalized News Aggregation and Recommendation Systems
WI/IAT(2020)
摘要
There are mounting concerns that personalized recommenders contribute to population differentiation and polarization by creating “information cocoons” that insulate people from opposing views. Despite the vast amount of research devoting to design new algorithms to improve the performance of recommendation systems, the evaluation mostly focuses on recommendation quality. Little attention is paid to the social impact of recommendation systems. On the other hand, it is noted in the literature that the users of recommendation systems suffer from “partial information blindness”, i.e. only exposed to the like-minded content information cocoon or filter bubble. Therefore, in this paper, we propose an impact evaluation framework of recommendation systems, comprising of five constructs, namely, diversity, objectivity, information cocoon, affect cocoon, and intentionality. The evaluation is conducted in a “black-box” way based on the empirical interaction data between the recommender and its individual users. To enable the individual level evaluation, we propose a lightweight agent-based simulation to collect individual’s interaction data with recommenders in the complex real-world scenarios. Furthermore, we illustrate the effectiveness of the proposed methodology with a case study in Toutiao and Baidu News, which are the top two news aggregator apps in China. The results show that both applications demonstrate a periodic information cocoon of about 7-day time interval, the news recommended through these two apps bear distinct differences: Toutiao has better captured the user’s reading preference and the quality of the recommended articles, while Baidu News demonstrate a higher affect cocoon and intentionality in the recommended articles, with average perceived difficulty of the recommended articles 2.9% higher than Toutiao, and average perceived persuasiveness 9.6% higher than Toutiao.
更多查看译文
关键词
evaluation framework,personalized recommendation,social impact
AI 理解论文
溯源树
样例
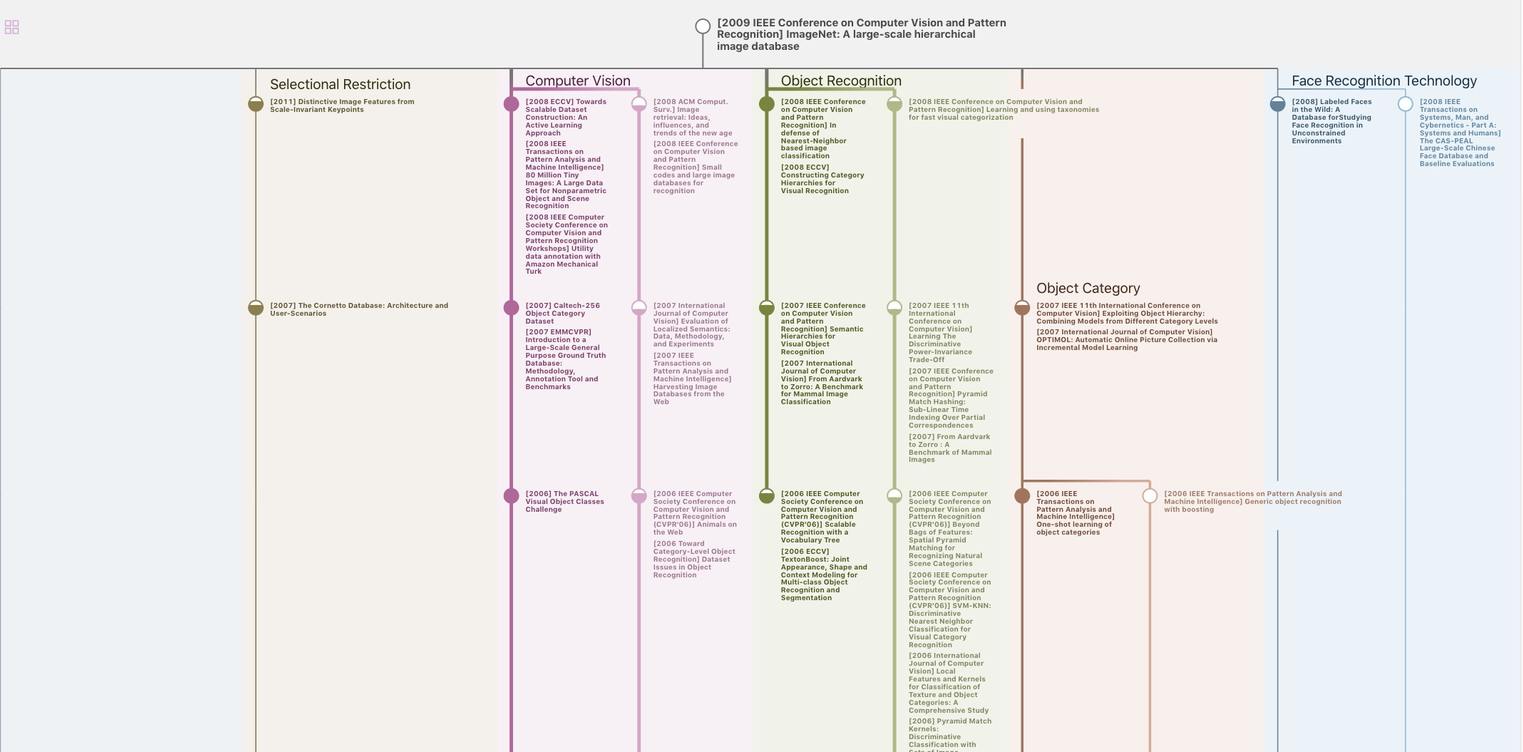
生成溯源树,研究论文发展脉络
Chat Paper
正在生成论文摘要