Federated Dynamic Spectrum Access
arxiv(2021)
摘要
Due to the growing volume of data traffic produced by the surge of Internet of Things (IoT) devices, the demand for radio spectrum resources is approaching their limitation defined by Federal Communications Commission (FCC). To this end, Dynamic Spectrum Access (DSA) is considered as a promising technology to handle this spectrum scarcity. However, standard DSA techniques often rely on analytical modeling wireless networks, making its application intractable in under-measured network environments. Therefore, utilizing neural networks to approximate the network dynamics is an alternative approach. In this article, we introduce a Federated Learning (FL) based framework for the task of DSA, where FL is a distributive machine learning framework that can reserve the privacy of network terminals under heterogeneous data distributions. We discuss the opportunities, challenges, and opening problems of this framework. To evaluate its feasibility, we implement a Multi-Agent Reinforcement Learning (MARL)-based FL as a realization associated with its initial evaluation results.
更多查看译文
关键词
spectrum,access
AI 理解论文
溯源树
样例
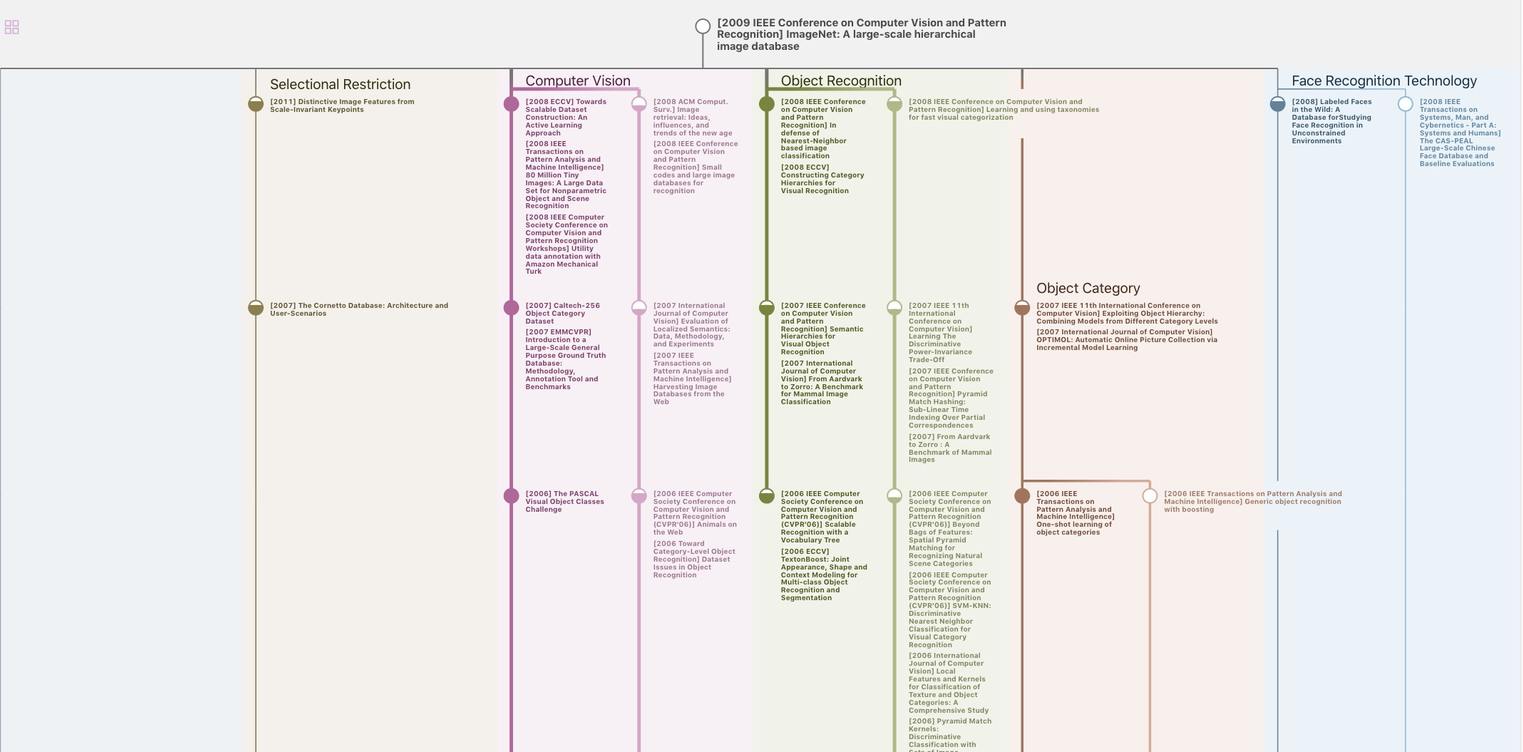
生成溯源树,研究论文发展脉络
Chat Paper
正在生成论文摘要