O2O-Afford - Annotation-Free Large-Scale Object-Object Affordance Learning.
CoRL(2021)
摘要
Contrary to the vast literature in modeling, perceiving, and understanding agent-object (e.g., human-object, hand-object, robot-object) interaction in computer vision and robotics, very few past works have studied the task of object-object interaction, which also plays an important role in robotic manipulation and planning tasks. There is a rich space of object-object interaction scenarios in our daily life, such as placing an object on a messy tabletop, fitting an object inside a drawer, pushing an object using a tool, etc. In this paper, we propose a unified affordance learning framework to learn object-object interaction for various tasks. By constructing four object-object interaction task environments using physical simulation (SAPIEN) and thousands of ShapeNet models with rich geometric diversity, we are able to conduct large-scale object-object affordance learning without the need for human annotations or demonstrations. At the core of technical contribution, we propose an object-kernel point convolution network to reason about detailed interaction between two objects. Experiments on large-scale synthetic data and real-world data prove the effectiveness of the proposed approach. Please refer to the project webpage for code, data, video, and more materials: https://cs.stanford.edu/~kaichun/o2oafford
更多查看译文
关键词
learning,o-afford,annotation-free,large-scale,object-object
AI 理解论文
溯源树
样例
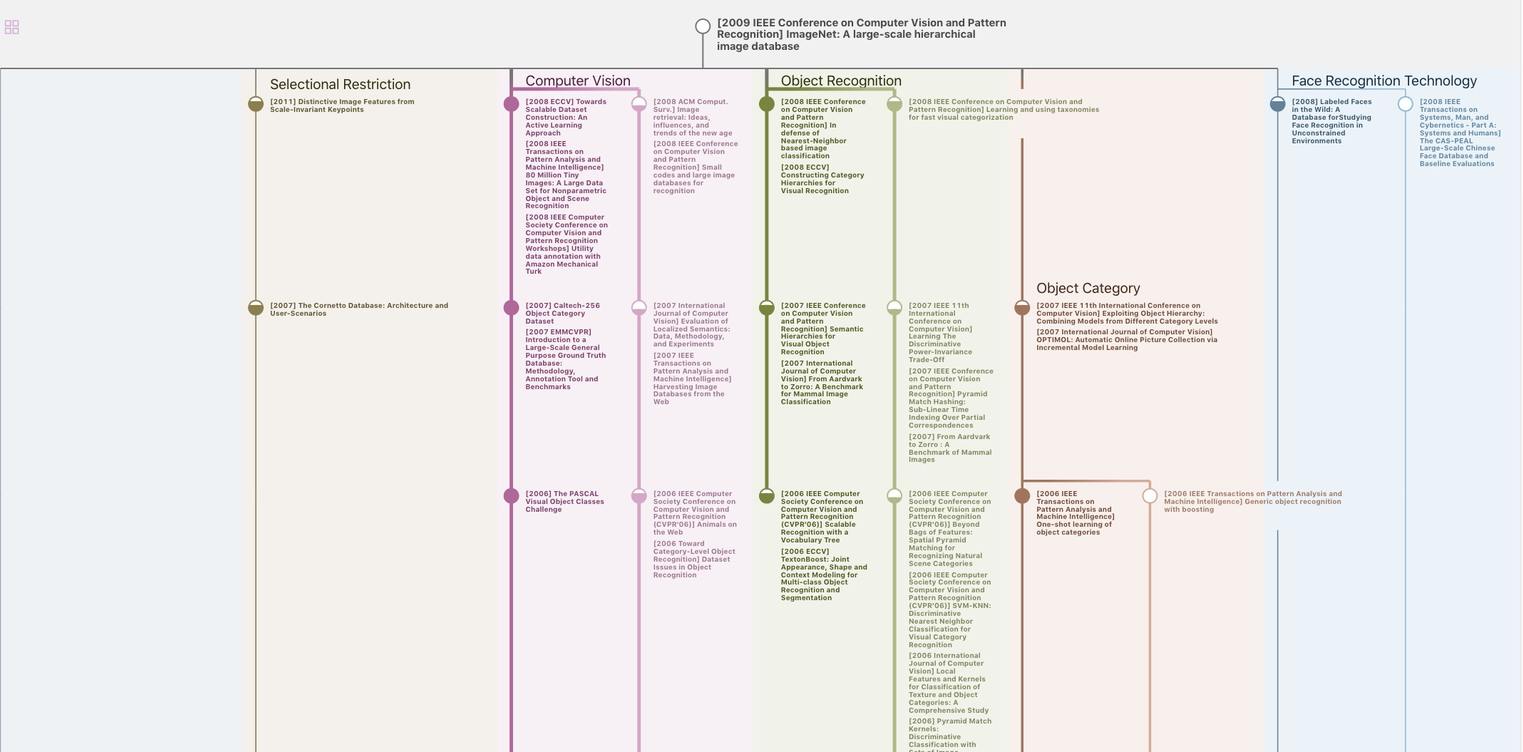
生成溯源树,研究论文发展脉络
Chat Paper
正在生成论文摘要