Optimal Rates for Random Order Online Optimization.
Annual Conference on Neural Information Processing Systems(2021)
摘要
We study online convex optimization in the random order model, recently proposed by Garber et al. (2020), where the loss functions may be chosen by an adversary, but are then presented to the online algorithm in a uniformly random order. Focusing on the scenario where the cumulative loss function is (strongly) convex, yet individual loss functions are smooth but might be non-convex, we give algorithms that achieve the optimal bounds and significantly outperform the results of Garber et al. (2020), completely removing the dimension dependence and improve their scaling with respect to the strong convexity parameter. Our analysis relies on novel connections between algorithmic stability and generalization for sampling without-replacement analogous to those studied in the with-replacement i.i.d. setting, as well as on a refined average stability analysis of stochastic gradient descent.
更多查看译文
关键词
optimal rates,optimization,online
AI 理解论文
溯源树
样例
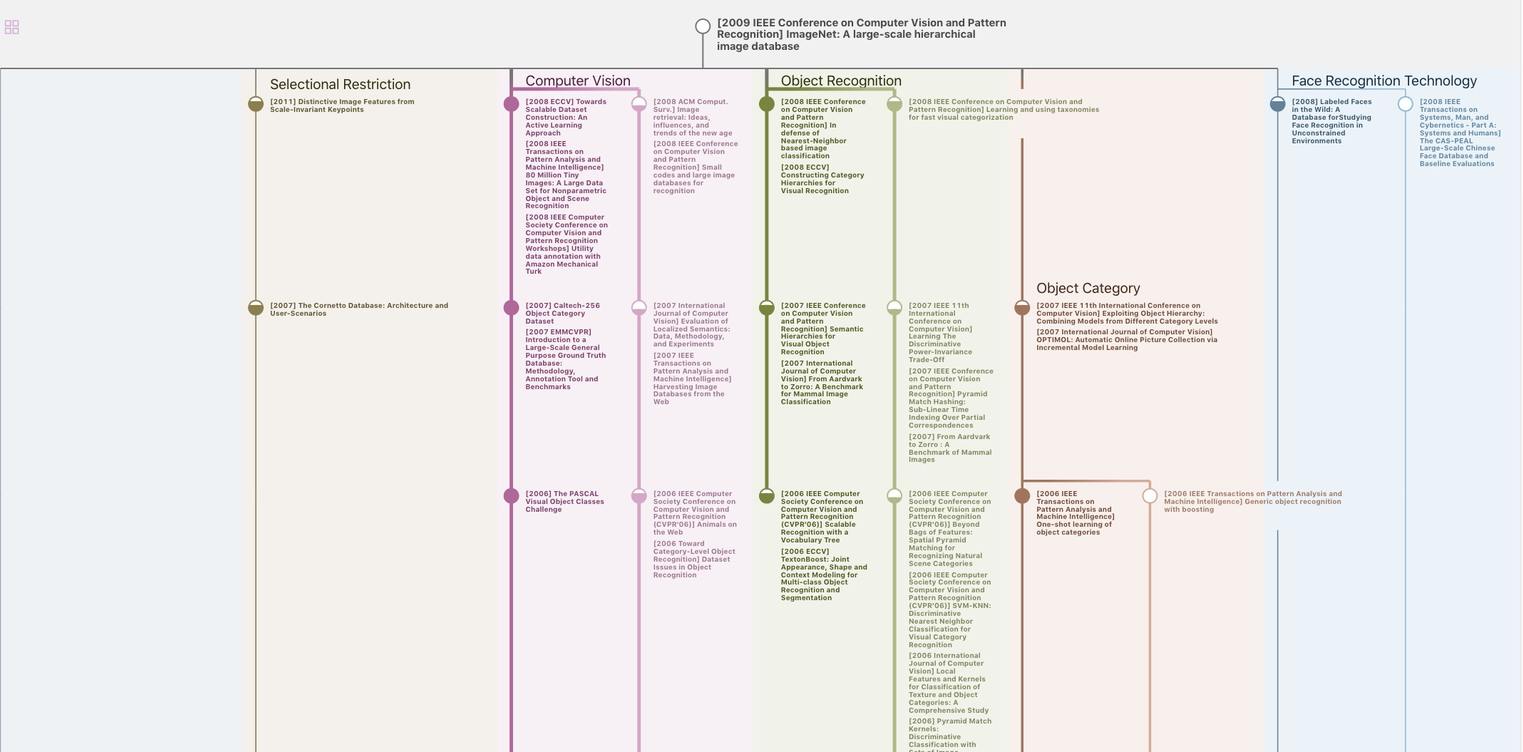
生成溯源树,研究论文发展脉络
Chat Paper
正在生成论文摘要