Learning in Continuous Action Space for Determination of High Dimensional Potential Energy Surfaces
user-5f8cf9244c775ec6fa691c99(2021)
摘要
Abstract Reinforcement learning (RL) approaches that combine a tree search with deep learning have found remarkable success in searching exorbitantly large, albeit discrete action spaces, as demonstrated recently in board games like chess, Shogi, and Go. Many real-world materials discovery and design applications, however, involve multi-dimensional search problems and learning domains that have continuous action spaces. Exploring high-dimensional potential energy surfaces (PES) of materials to represent inter- and intra-molecular interactions, for example, involves a continuous action search to find optimal potential parameters or coefficients. Traditionally, these searches are time consuming (often several years for a single system) and have been driven by human intuition and/or expertise and more recently by global/local optimization searches that have issues with convergence and/or do not scale well with the search dimensionality. Here, in a departure from discrete action and other gradient-based approaches, we introduce a RL strategy based on decision trees that incorporates modified rewards for improved exploration, efficient sampling during playouts, and a “window scaling scheme” for enhanced exploitation, to enable efficient and scalable search for continuous action space problems. Using high-dimensional artificial landscapes and control RL problems, we successfully benchmark our approach against popular global optimization schemes and state-of-the-art policy gradient methods, respectively. We further demonstrate its efficacy to perform high-throughput PES search for 54 different elemental systems across the Periodic table, in- including alkali, alkaline-earth, transition metals, metalloids, as well as non-metals. Using a well-sampled (∼165,000 configurations) first-principles derived training and test dataset, we demonstrate that the new class of RL trained bond-order potentials capture the size-dependent energetic landscape from few atom clusters to bulk (energy errors << 200 meV/atom over a 3-6 eV sampled range) as well as their dynamics (force errors << 0.5 eV/A over a 50-100 eV/A range). We analyze the error trends across different elements in the latent space and trace their origin to elemental structural diversity and the smoothness of the element energy surface. Finally, we run molecular dynamics using these RL trained potentials and perform a comprehensive test of dynamic stability of more than 40,000 clusters sampled for different elements across the Periodic table. Our newly developed high-quality potentials will enable accelerated nanoscale materials design and discovery. Broadly, our RL strategy will be applicable to many other physical science problems involving search over continuous action spaces.
更多查看译文
关键词
Space (mathematics),Action (physics),Potential energy,Classical mechanics,Physics,High dimensional
AI 理解论文
溯源树
样例
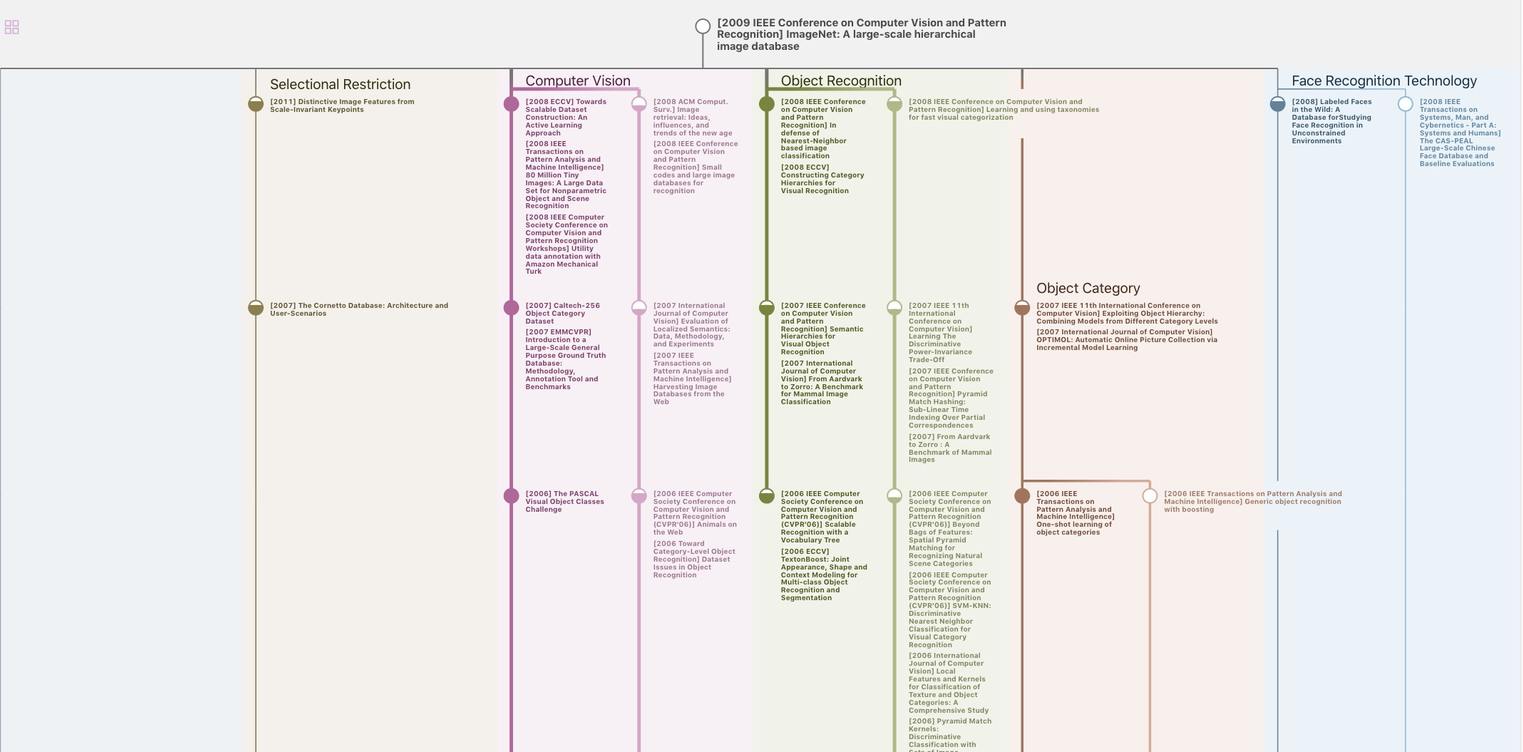
生成溯源树,研究论文发展脉络
Chat Paper
正在生成论文摘要