DRLPart: A Deep Reinforcement Learning Framework for Optimally Efficient and Robust Resource Partitioning on Commodity Servers
HPDC(2021)
摘要
ABSTRACTWorkload consolidation is a commonly used approach for improving resource utilization of commodity servers. However, colocated workloads often suffer from significant performance degradations due to resource contention, which makes resource partitioning an important research problem. Partitioning multiple resources coordinately is particularly challenging due to the complex contention behaviors and huge solution space, which is not well-addressed in the literature. In this paper, we propose a deep reinforcement learning (DRL) framework, named DRLPart, for solving the problem of partitioning multiple resources coordinately. DRLPart learns the optimal partitioning decision from easy-to-collect real-time system state, without need of domain knowledge and handcrafted search heuristics. We solve two critical challenges of applying DRL to the resource partitioning problem. First, we build a deep-learning based performance model, which significantly reduces the training overhead, by estimating the rewards of actions without interacting with real system. Second, we propose a fine-tuning process to improve bad decisions occasionally made by the DRL model, which enhances the adaptivity to new situations. Results from extensive evaluations show that the proposed framework is optimally efficient and robust, which improves the system throughput by 13.3%~18.5 compared to the state-of-the-art baselines.
更多查看译文
AI 理解论文
溯源树
样例
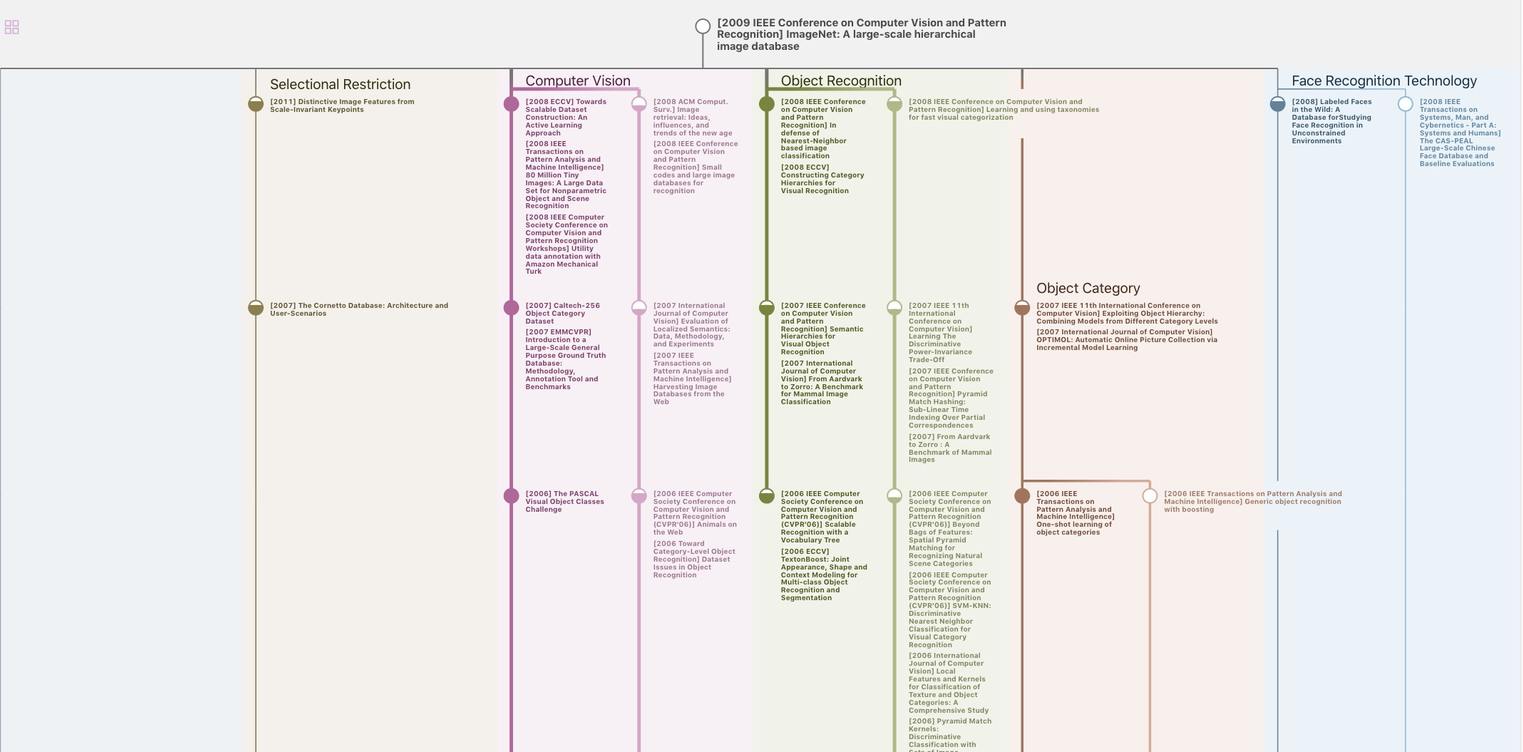
生成溯源树,研究论文发展脉络
Chat Paper
正在生成论文摘要