ELBERT: FAST ALBERT WITH CONFIDENCE-WINDOW BASED EARLY EXIT
2021 IEEE INTERNATIONAL CONFERENCE ON ACOUSTICS, SPEECH AND SIGNAL PROCESSING (ICASSP 2021)(2021)
摘要
Despite the great success in Natural Language Processing (NLP) area, large pre-trained language models like BERT are not well-suited for resource-constrained or real-time applications owing to the large number of parameters and slow inference speed. Recently, compressing and accelerating BERT have become important topics. By incorporating a parameter-sharing strategy, ALBERT greatly reduces the number of parameters while achieving competitive performance. Nevertheless, ALBERT still suffers from a long inference time. In this work, we propose the ELBERT, which significantly improves the average inference speed compared to ALBERT due to the proposed confidence-window based early exit mechanism, without introducing additional parameters or extra training overhead. Experimental results show that ELBERT achieves an adaptive inference speedup varying from 2x to 10x with negligible accuracy degradation compared to ALBERT on various datasets. Besides, ELBERT achieves higher accuracy than existing early exit methods used for accelerating BERT under the same computation cost. Furthermore, to understand the principle of the early exit mechanism, we also visualize the decision-making process of it in ELBERT. Our code is publicly available online.(1)
更多查看译文
关键词
Natural Language Processing, BERT, Inference Acceleration, Early Exit, Model Compression
AI 理解论文
溯源树
样例
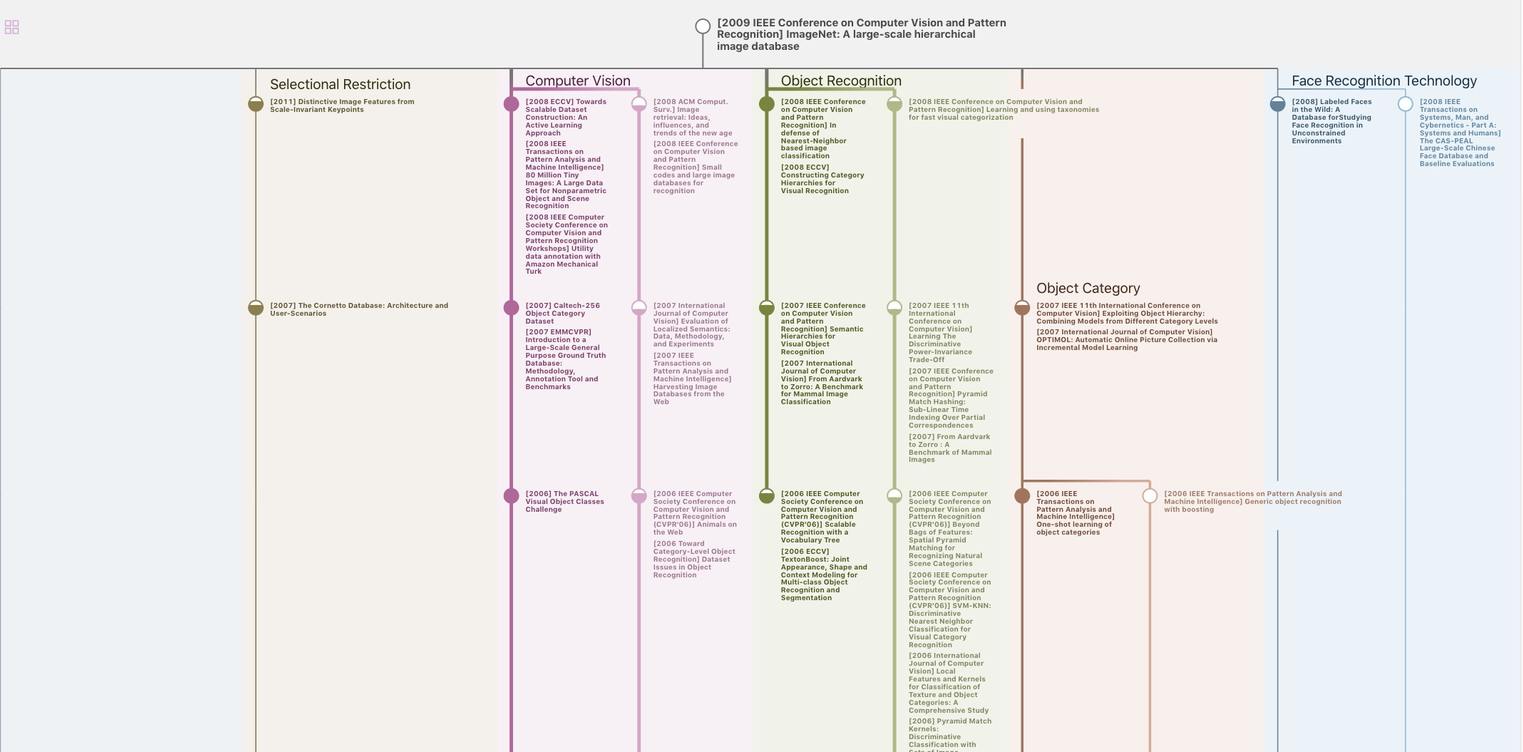
生成溯源树,研究论文发展脉络
Chat Paper
正在生成论文摘要