A Consistency-Based Loss for Deep Odometry Through Uncertainty Propagation
2023 IEEE INTERNATIONAL CONFERENCE ON ROBOTICS AND AUTOMATION (ICRA 2023)(2023)
Abstract
Conventionally, deep odometry networks use objective functions that only penalize short-term deviations from the true path. Since such an objective does not impose any constraints on the long-term deviations from the path, a second consistency-based loss term may be added to lower long-term drift. However, maintaining a balance between the two loss terms is challenging and often treated as a design hyperparameter. To mitigate this balancing issue, we propose to use the uncertainty over both odometry and the long-term transformations in a maximum likelihood setting and allow the network to tune the weighting between the two loss terms. To this end, we derive the odometry uncertainty alongside the pose outputs using the network itself and to derive the covariance matrix over the integrated transformation, we propose to propagate the odometry uncertainty through each iteration. This formulation provides an adaptive and statistically consistent method to weigh the incremental and integrated loss terms against each other, noting the increase in uncertainty as more steps are integrated over. We show that our approach to consistency-based losses allows the network to surpass the accuracy of the state-of-the-art visual odometry approaches. Then, the efficacy of the derived uncertainty as weighting medium is visualized and the performance benefits of uncertainty quantification are shown in a pose-graph based localization scenario.
MoreTranslated text
Key words
adaptive method,consistency-based loss term,covariance matrix,deep odometry networks,design hyperparameter,incremental loss terms,integrated loss terms,integrated transformation,long-term deviations,long-term drift,long-term transformations,maximum likelihood setting,objective functions,odometry uncertainty,pose-graph based localization scenario,short-term deviations,statistically consistent method,uncertainty propagation,uncertainty quantification,visual odometry approaches,weighting medium
AI Read Science
Must-Reading Tree
Example
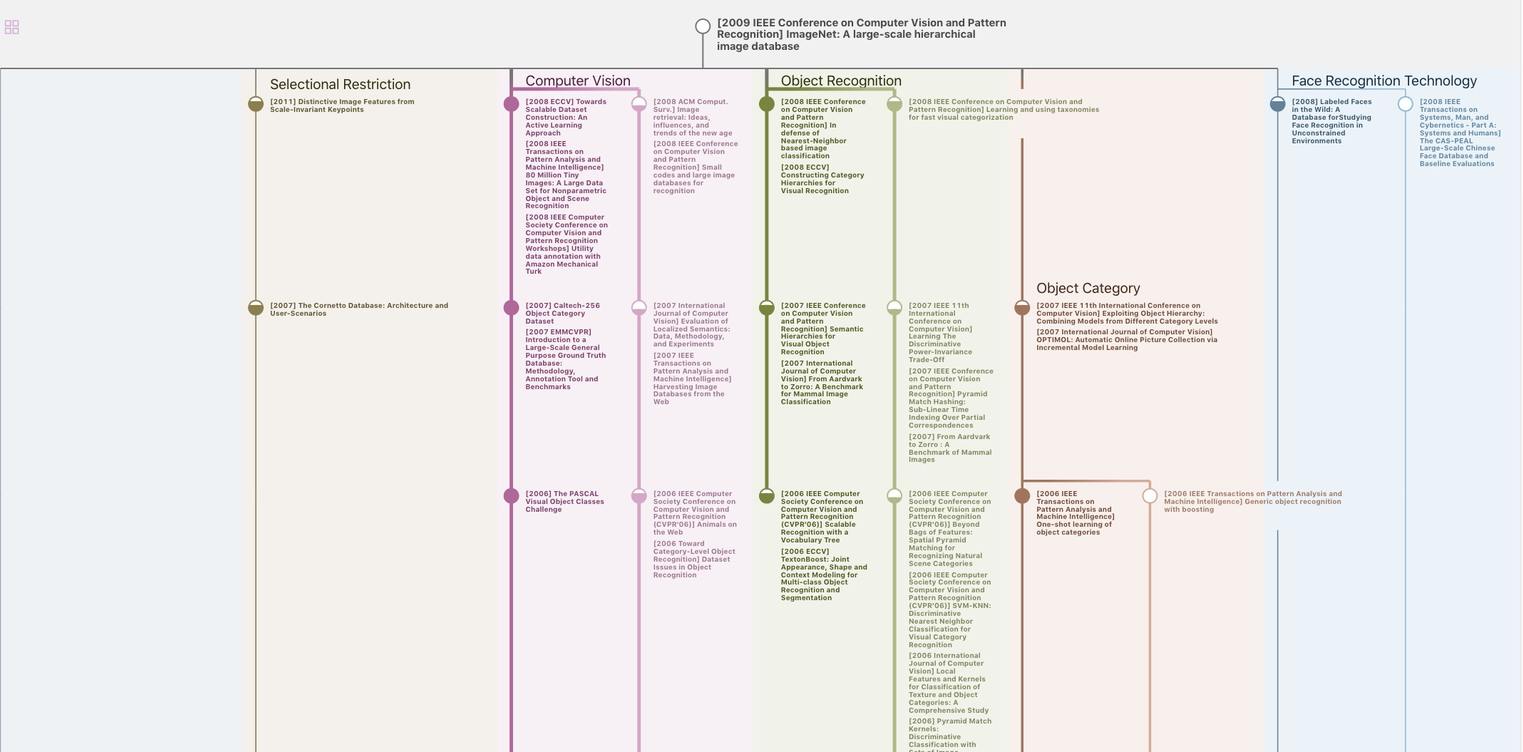
Generate MRT to find the research sequence of this paper
Chat Paper
Summary is being generated by the instructions you defined