Towards Fairness-Aware Ranking by Defining Latent Groups Using Inferred Features.
BIAS(2021)
Abstract
Group fairness in search and recommendation is drawing increasing attention in recent years. This paper explores how to define latent groups, which cannot be determined by self-contained features but must be inferred from external data sources, for fairness-aware ranking. In particular, taking the Semantic Scholar dataset released in TREC 2020 Fairness Ranking Track as a case study, we infer and extract multiple fairness related dimensions of author identity including gender and location to construct groups. Furthermore, we propose a fairness-aware re-ranking algorithm incorporating both weighted relevance and diversity of returned items for given queries. Our experimental results demonstrate that different combinations of relative weights assigned to relevance, gender, and location groups perform as expected.
MoreTranslated text
Key words
Fair ranking,Text retrieval,Fair exposure,Information retrieval,Fairness,Ranking
AI Read Science
Must-Reading Tree
Example
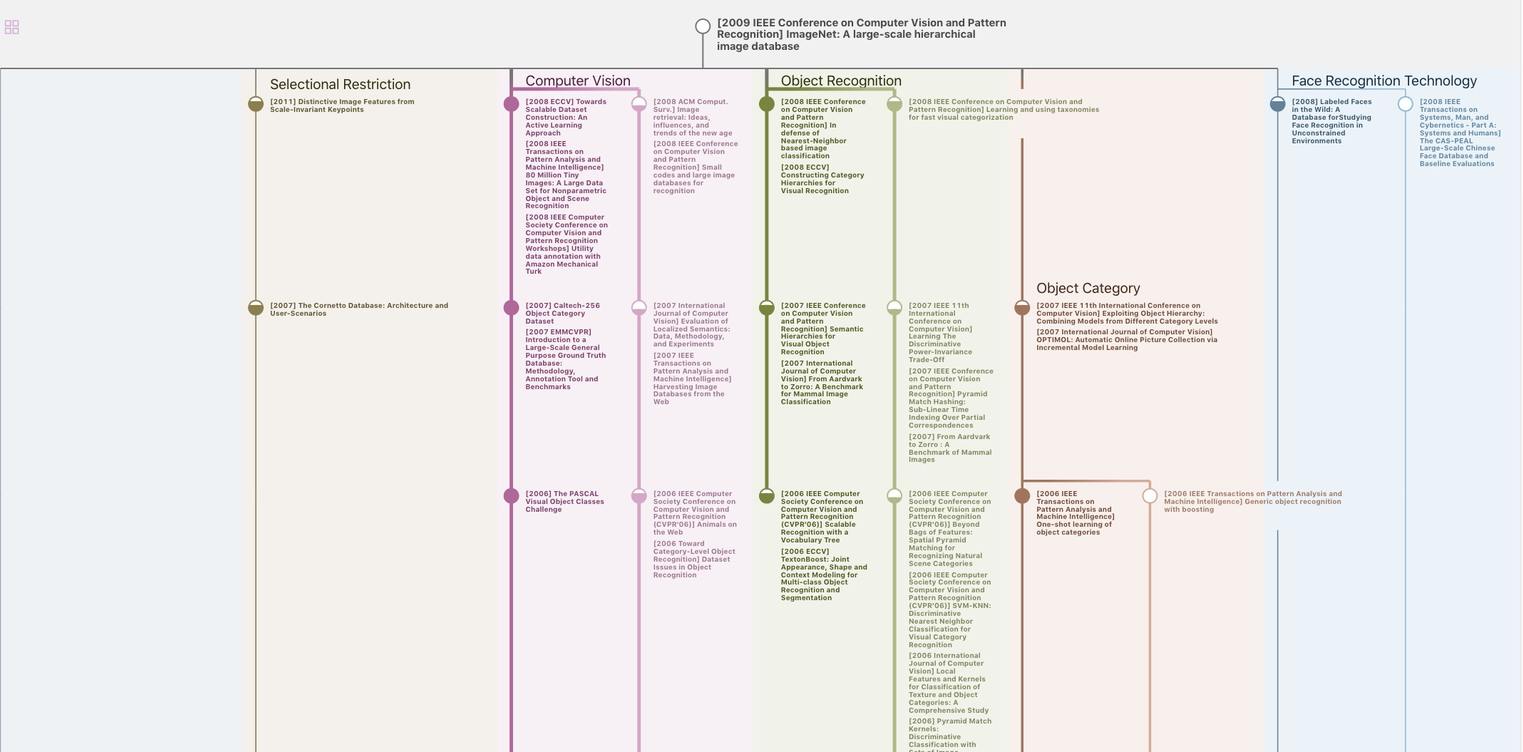
Generate MRT to find the research sequence of this paper
Chat Paper
Summary is being generated by the instructions you defined