Sdof-Gan: Symmetric Dense Optical Flow Estimation With Generative Adversarial Networks
IEEE TRANSACTIONS ON IMAGE PROCESSING(2021)
摘要
There is a growing consensus in computer vision that symmetric optical flow estimation constitutes a better model than a generic asymmetric one for its independence of the selection of source/target image. Yet, convolutional neural networks (CNNs), that are considered the de facto standard vision model, deal with the asymmetric case only in most cutting-edge CNNs-based optical flow techniques. We bridge this gap by introducing a novel model named SDOF-GAN: symmetric dense optical flow with generative adversarial networks (GANs). SDOF-GAN realizes a consistency between the forward mapping (source-to-target) and the backward one (target-to-source) by ensuring that they are inverse of each other with an inverse network. In addition, SDOF-GAN leverages a GAN model for which the generator estimates symmetric optical flow fields while the discriminator differentiates the "real" ground-truth flow field from a "fake" estimation by assessing the flow warping error. Finally, SDOF-GAN is trained in a semi-supervised fashion to enable both the precious labeled data and large amounts of unlabeled data to be fully-exploited. We demonstrate significant performance benefits of SDOF-GAN on five publicly-available datasets in contrast to several representative state-of-the-art models for optical flow estimation.
更多查看译文
关键词
Estimation, Optical imaging, Adaptive optics, Generative adversarial networks, Brightness, Optical fiber networks, Semisupervised learning, Symmetric optical flow estimation, generative adversarial networks, forward-backward consistency, semi-supervised learning
AI 理解论文
溯源树
样例
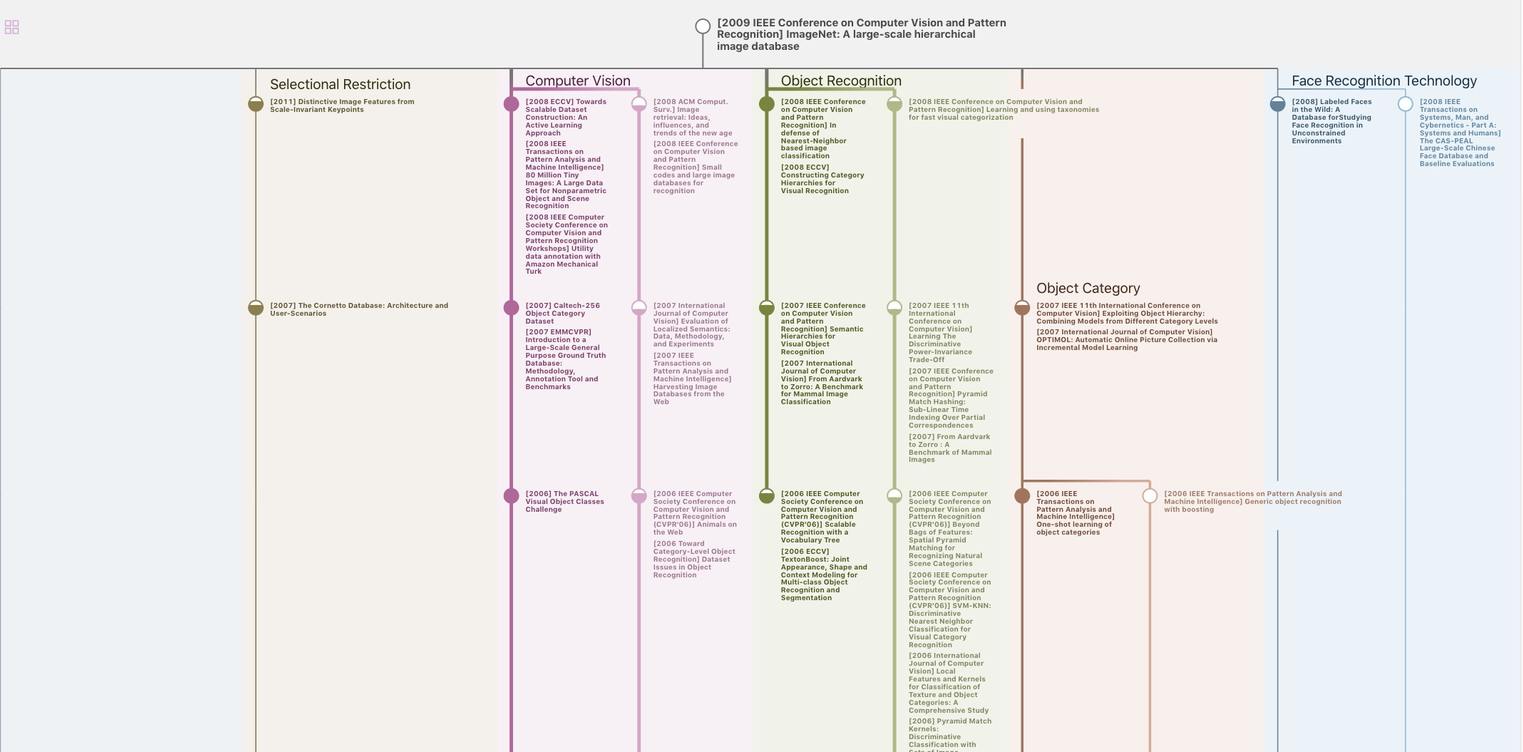
生成溯源树,研究论文发展脉络
Chat Paper
正在生成论文摘要