Predicting Lung Cancer In The United States: A Multiple Model Examination Of Public Health Factors
INTERNATIONAL JOURNAL OF ENVIRONMENTAL RESEARCH AND PUBLIC HEALTH(2021)
摘要
In this research, we take a multivariate, multi-method approach to predicting the incidence of lung cancer in the United States. We obtain public health and ambient emission data from multiple sources in 2000-2013 to model lung cancer in the period 2013-2017. We compare several models using four sources of predictor variables: adult smoking, state, environmental quality index, and ambient emissions. The environmental quality index variables pertain to macro-level domains: air, land, water, socio-demographic, and built environment. The ambient emissions consist of Cyanide compounds, Carbon Monoxide, Carbon Disulfide, Diesel Exhaust, Nitrogen Dioxide, Tropospheric Ozone, Coarse Particulate Matter, Fine Particulate Matter, and Sulfur Dioxide. We compare various models and find that the best regression model has variance explained of 62 percent whereas the best machine learning model has 64 percent variance explained with 10% less error. The most hazardous ambient emissions are Coarse Particulate Matter, Fine Particulate Matter, Sulfur Dioxide, Carbon Monoxide, and Tropospheric Ozone. These ambient emissions could be curtailed to improve air quality, thus reducing the incidence of lung cancer. We interpret and discuss the implications of the model results, including the tradeoff between transparency and accuracy. We also review limitations of and directions for the current models in order to extend and refine them.
更多查看译文
关键词
adult smoking, lung cancer, united states, regression, environmental quality index, ambient emissions, machine learning, transparency, iterative modeling
AI 理解论文
溯源树
样例
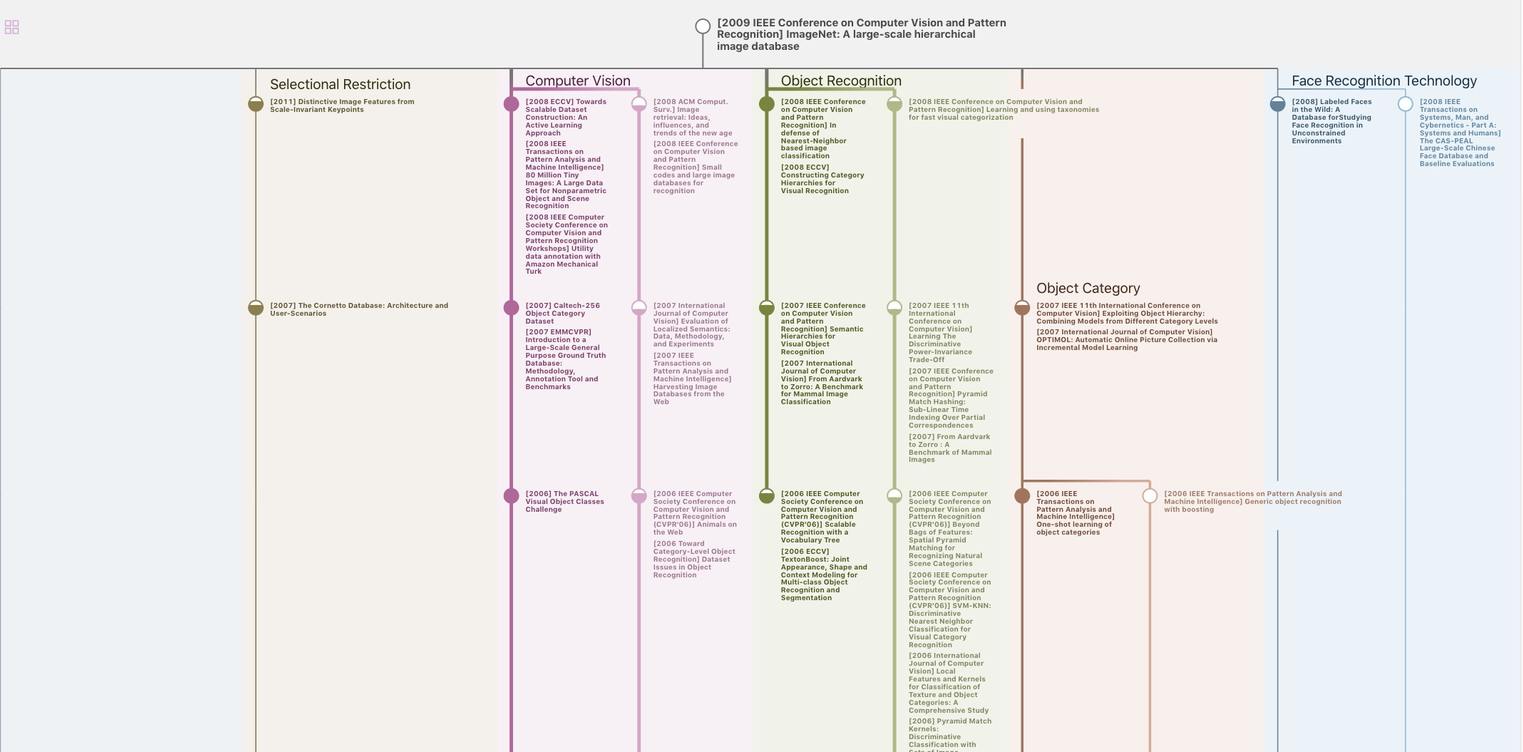
生成溯源树,研究论文发展脉络
Chat Paper
正在生成论文摘要