Cross Attention Squeeze Excitation Network (Case-Net) for Whole Body Fetal MRI Segmentation
Italian National Conference on Sensors(2021)
摘要
Segmentation of the fetus from 2-dimensional (2D) magnetic resonance imaging (MRI) can aid radiologists with clinical decision making for disease diagnosis. Machine learning can facilitate this process of automatic segmentation, making diagnosis more accurate and user independent. We propose a deep learning (DL) framework for 2D fetal MRI segmentation using a Cross Attention Squeeze Excitation Network (CASE-Net) for research and clinical applications. CASE-Net is an end-to-end segmentation architecture with relevant modules that are evidence based. The goal of CASE-Net is to emphasize localization of contextual information that is relevant in biomedical segmentation, by combining attention mechanisms with squeeze-and-excitation (SE) blocks. This is a retrospective study with 34 patients. Our experiments have shown that our proposed CASE-Net achieved the highest segmentation Dice score of 87.36%, outperforming other competitive segmentation architectures.
更多查看译文
关键词
deep learning,fetal MRI,convolutional neural networks,automatic segmentation,squeeze-and-excitation,attention mechanisms
AI 理解论文
溯源树
样例
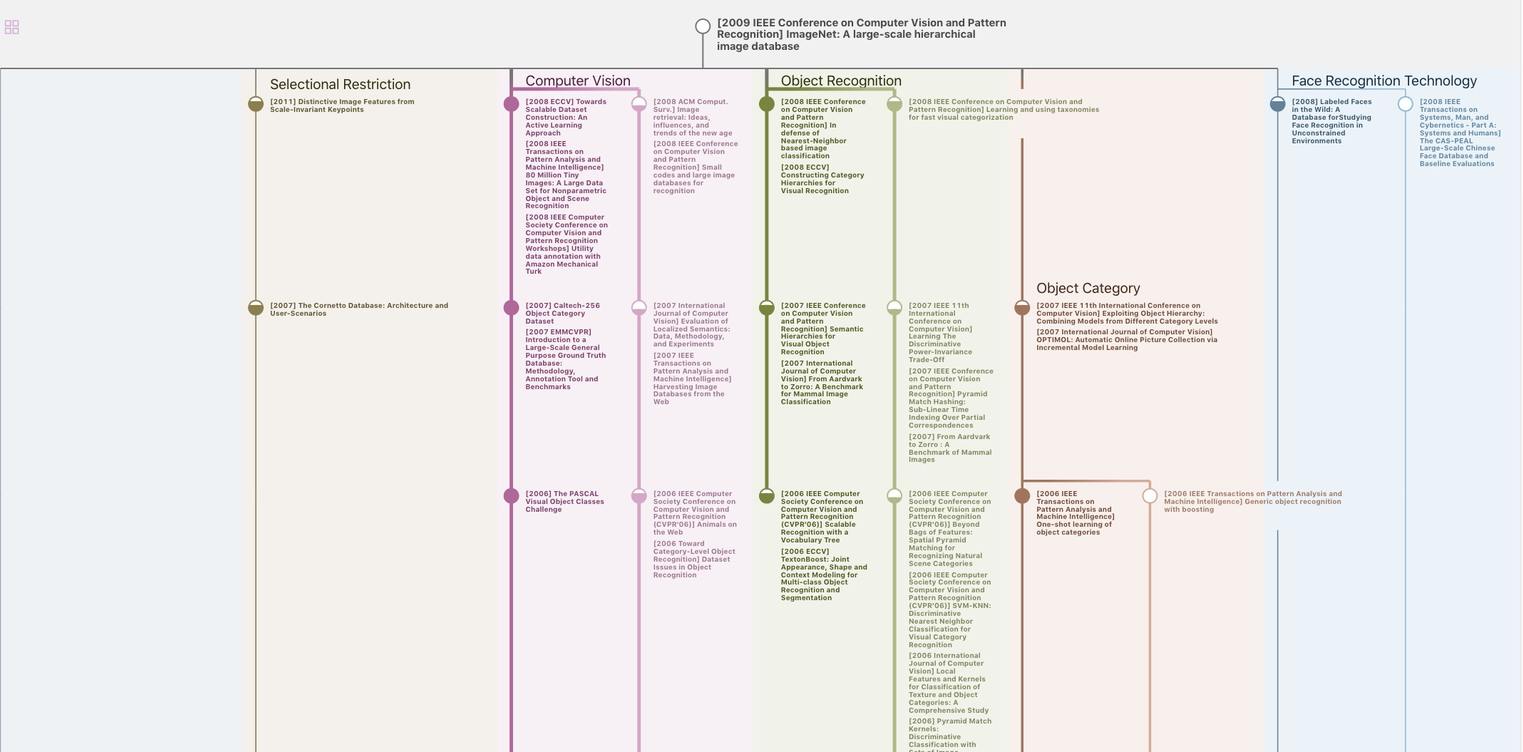
生成溯源树,研究论文发展脉络
Chat Paper
正在生成论文摘要