IMPROVING IDENTIFICATION OF SYSTEM-DIRECTED SPEECH UTTERANCES BY DEEP LEARNING OF ASR-BASED WORD EMBEDDINGS AND CONFIDENCE METRICS
2021 IEEE INTERNATIONAL CONFERENCE ON ACOUSTICS, SPEECH AND SIGNAL PROCESSING (ICASSP 2021)(2021)
摘要
In this paper, we extend our previous work on the detection of system-directed speech utterances. This type of binary classification can be used by virtual assistants to create a more natural and fluid interaction between the system and the user. We explore two methods that both improve the Equal-Error-Rate (EER) performance of the previous model. The first exploits the supplementary information independently captured by ASR models through integrating ASR decoder-based features as additional inputs to the final classification stage of the model. This relatively improves EER performance by 13%. The second proposed method further integrates word embeddings into the architecture and, when combined with the first method, achieves a significant EER performance improvement of 48%, relative to that of the baseline.
更多查看译文
关键词
Human-machine interaction, spoken utterance classification, wake-up phrase/word
AI 理解论文
溯源树
样例
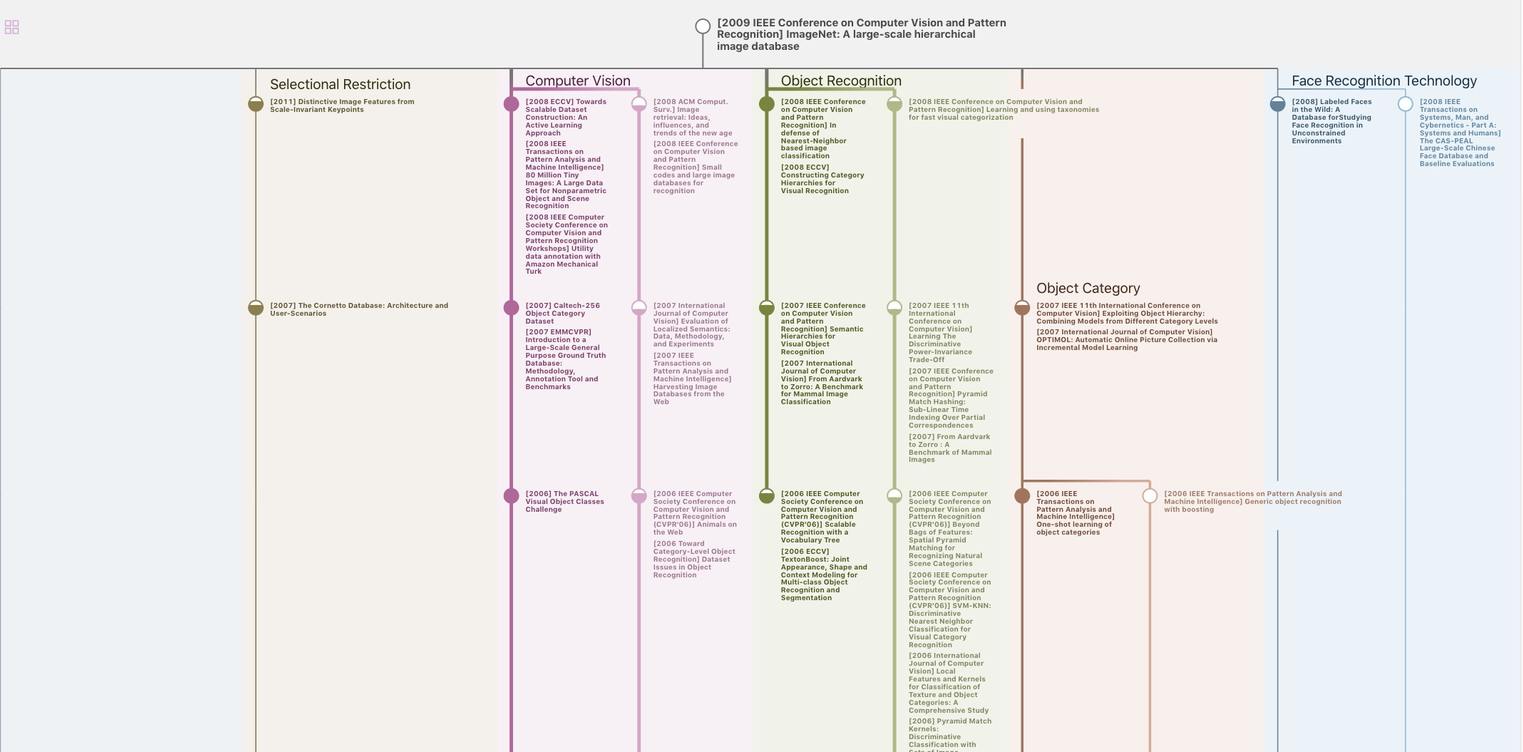
生成溯源树,研究论文发展脉络
Chat Paper
正在生成论文摘要