AN EMPIRICAL STUDY ON TASK-ORIENTED DIALOGUE TRANSLATION
2021 IEEE INTERNATIONAL CONFERENCE ON ACOUSTICS, SPEECH AND SIGNAL PROCESSING (ICASSP 2021)(2021)
摘要
Translating conversational text, in particular task-oriented dialogues, is an important application task for machine translation technology. However, it has so far not been extensively explored due to its inherent characteristics including data limitation, discourse, informality and personality. In this paper, we systematically investigate advanced models on the task-oriented dialogue translation task, including sentence-level, document-level and non-autoregressive NMT models. Besides, we explore existing techniques such as data selection, back/forward translation, larger batch learning, finetuning and domain adaptation. To alleviate low-resource problem, we transfer general knowledge from four different pre-training models to the downstream task. Encouragingly, we find that the best model with mBART pre-training pushes the SOTA performance on WMT20 English-German and IWSLT DIALOG Chinese-English datasets up to 62.67 and 23.21 BLEU points, respectively.(1)
更多查看译文
关键词
Task-Oriented Dialogue, Neural Machine Translation, Multilingual, Pre-Training, Discourse
AI 理解论文
溯源树
样例
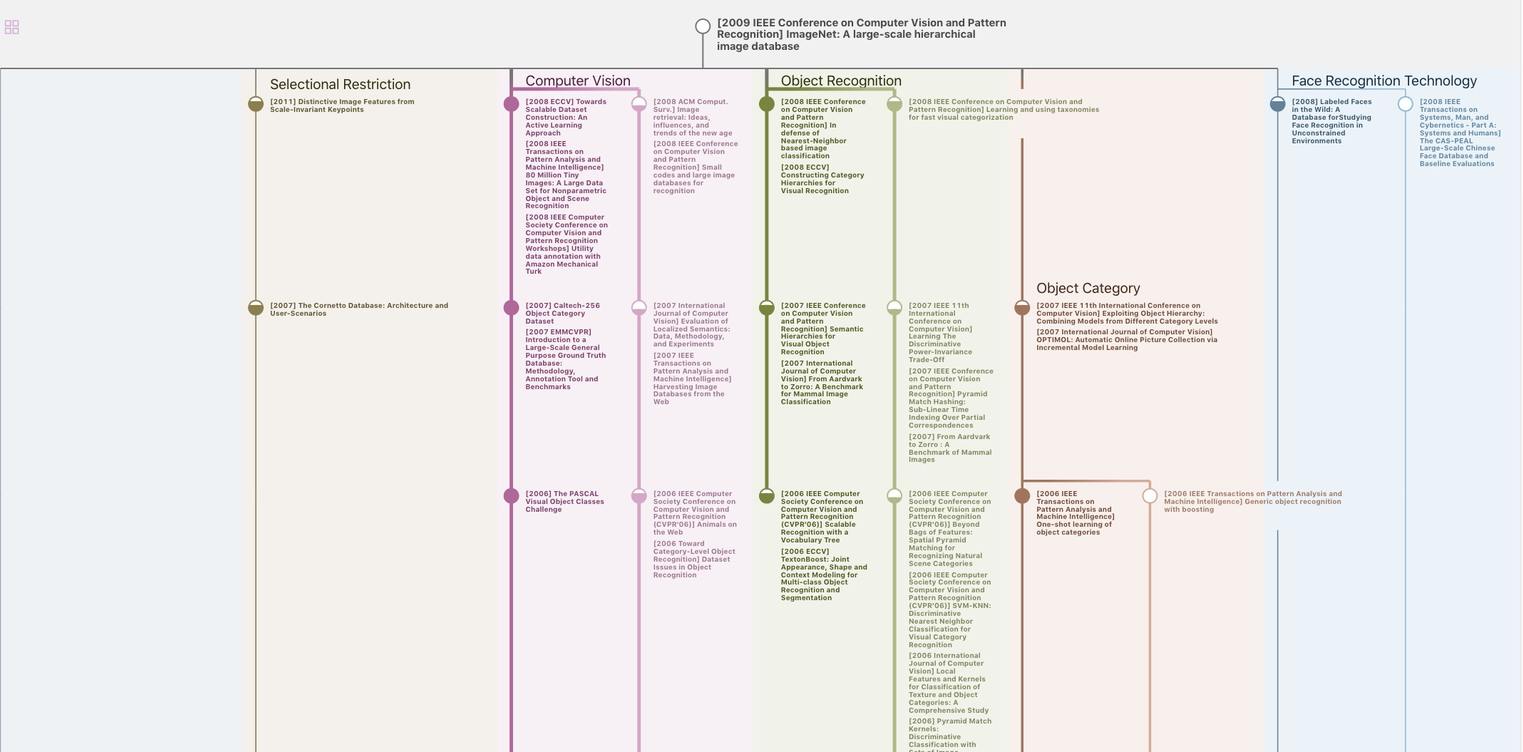
生成溯源树,研究论文发展脉络
Chat Paper
正在生成论文摘要